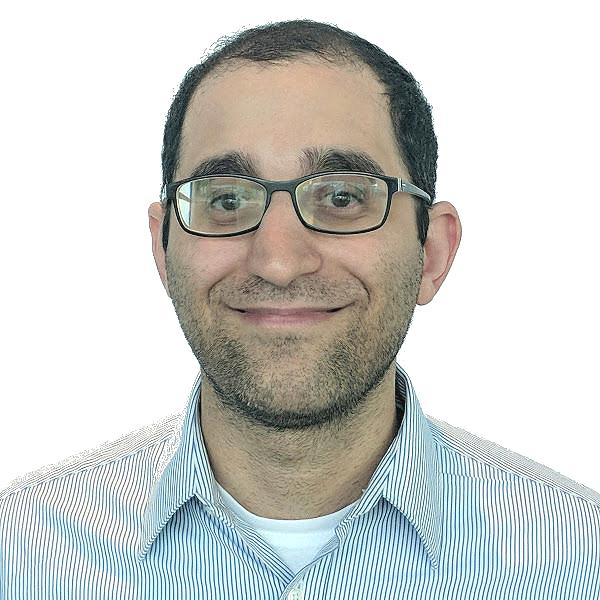
Farhad Hormozdiari
Farhad is a research scientist in the genomics team at Google Health, where he combines genetic data and machine learning techniques to improve disease predictions for a diverse set of populations. His long-term research aims are to better healthcare outcomes and to lower costs. Prior to Google, Farhad was a postdoctoral fellow at Broad Institute and Harvard T.H. Chan School of Public Health working on understanding the biological mechanisms of diseases. Farhad obtained his PhD in computer science at UCLA while working on statistical methods to detect causal variants for a wide range of diseases. Farhad has published over 70+ peer-reviewed journals including Nature, Nature Genetics and Science. Farhad has won many awards including the Best Paper in ISMB/ECCB 2015.
Research Areas
Authored Publications
Sort By
Unsupervised representation learning on high-dimensional clinical data improves genomic discovery and prediction
Babak Behsaz
Zachary Ryan Mccaw
Davin Hill
Robert Luben
Dongbing Lai
John Bates
Howard Yang
Tae-Hwi Schwantes-An
Yuchen Zhou
Anthony Khawaja
Andrew Carroll
Brian Hobbs
Michael Cho
Nature Genetics (2024)
Preview abstract
Although high-dimensional clinical data (HDCD) are increasingly available in biobank-scale datasets, their use for genetic discovery remains challenging. Here we introduce an unsupervised deep learning model, Representation Learning for Genetic Discovery on Low-Dimensional Embeddings (REGLE), for discovering associations between genetic variants and HDCD. REGLE leverages variational autoencoders to compute nonlinear disentangled embeddings of HDCD, which become the inputs to genome-wide association studies (GWAS). REGLE can uncover features not captured by existing expert-defined features and enables the creation of accurate disease-specific polygenic risk scores (PRSs) in datasets with very few labeled data. We apply REGLE to perform GWAS on respiratory and circulatory HDCD—spirograms measuring lung function and photoplethysmograms measuring blood volume changes. REGLE replicates known loci while identifying others not previously detected. REGLE are predictive of overall survival, and PRSs constructed from REGLE loci improve disease prediction across multiple biobanks. Overall, REGLE contain clinically relevant information beyond that captured by existing expert-defined features, leading to improved genetic discovery and disease prediction.
View details
Inference of chronic obstructive pulmonary disease with deep learning on raw spirograms identifies new genetic loci and improves risk models
Babak Behsaz
Babak Alipanahi
Zachary Ryan Mccaw
Davin Hill
Tae-Hwi Schwantes-An
Dongbing Lai
Andrew Carroll
Brian Hobbs
Michael Cho
Nature Genetics (2023)
Preview abstract
Chronic obstructive pulmonary disease (COPD), the third leading cause of death worldwide, is highly heritable. While COPD is clinically defined by applying thresholds to summary measures of lung function, a quantitative liability score has more power to identify genetic signals. Here we train a deep convolutional neural network on noisy self-reported and International Classification of Diseases labels to predict COPD case-control status from high-dimensional raw spirograms and use the model's predictions as a liability score. The machine-learning-based (ML-based) liability score accurately discriminates COPD cases and controls, and predicts COPD-related hospitalization without any domain-specific knowledge. Moreover, the ML-based liability score is associated with overall survival and exacerbation events. A genome-wide association study on the ML-based liability score replicates existing COPD and lung function loci and also identifies 67 new loci. Lastly, our method provides a general framework to use ML methods and medical-record-based labels that does not require domain knowledge or expert curation to improve disease prediction and genomic discovery for drug design.
View details
Multimodal LLMs for health grounded in individual-specific data
Anastasiya Belyaeva
Krish Eswaran
Shravya Shetty
Andrew Carroll
Nick Furlotte
ICML Workshop on Machine Learning for Multimodal Healthcare Data (2023)
Preview abstract
Large language models (LLMs) have shown an impressive ability to solve tasks in a wide range of fields including health. Within the health domain, there are many data modalities that are relevant to an individual’s health status. To effectively solve tasks related to individual health, LLMs will need the ability to use a diverse set of features as context. However, the best way to encode and inject complex high-dimensional features into the input stream of an LLM remains an active area of research. Here, we explore the ability of a foundation LLM to estimate disease risk given health-related input features. First, we evaluate serialization of structured individual-level health data into text along with in context learning and prompt tuning approaches. We find that the LLM performs better than random in the zero-shot and few-shot cases, and has comparable and often equivalent performance to baseline after prompt tuning. Next, we propose a way to encode complex non-text data modalities into the token embedding space and then use this encoding to construct multimodal sentences. We show that this multimodal LLM achieves better or equivalent performance compared to baseline models. Overall, our results show the potential for using multi-modal LLMs grounded in individual health data to solve complex tasks such as risk prediction.
View details
Preview abstract
Genome-wide association studies (GWAS) are used to identify genetic variants significantly correlated with a target disease or phenotype as a first step to detect potentially causal genes. The availability of high-dimensional biomedical data in population-scale biobanks has enabled novel machine-learning-based phenotyping approaches in which machine learning (ML) algorithms rapidly and accurately phenotype large cohorts with both genomic and clinical data, increasing the statistical power to detect variants associated with a given phenotype. While recent work has demonstrated that these methods can be extended to diseases for which only low quality medical-record-based labels are available, it is not possible to quantify changes in statistical power since the underlying ground-truth liability scores for the complex, polygenic diseases represented by these medical-record-based phenotypes is unknown. In this work, we aim to empirically study the robustness of ML-based phenotyping procedures to label noise by applying varying levels of random noise to vertical cup-to-disc ratio (VCDR), a quantitative feature of the optic nerve that is predictable from color fundus imagery and strongly influences glaucoma referral risk. We show that the ML-based phenotyping procedure recovers the underlying liability score across noise levels, significantly improving genetic discovery and PRS predictive power relative to noisy equivalents. Furthermore, initial denoising experiments show promising preliminary results, suggesting that improving such methods will yield additional gains.
View details
DeepNull models non-linear covariate effects to improve phenotypic prediction and association power
Andrew Carroll
Babak Alipanahi
Zachary Ryan Mccaw
Nick Furlotte
Nature Communications (2022)
Preview abstract
Genome-wide association studies (GWAS) examine the association between genotype and phenotype while adjusting for a set of covariates. Although the covariates may have non-linear or interactive effects, due to the challenge of specifying the model, GWAS often neglect such terms. Here we introduce DeepNull, a method that identifies and adjusts for non-linear and interactive covariate effects using a deep neural network. In analyses of simulated and real data, we demonstrate that DeepNull maintains tight control of the type I error while increasing statistical power by up to 20% in the presence of non-linear and interactive effects. Moreover, in the absence of such effects, DeepNull incurs no loss of power. When applied to 10 phenotypes from the UK Biobank (n=370K), DeepNull discovered more hits (+6%) and loci (+7%), on average, than conventional association analyses, many of which are biologically plausible or have previously been reported. Finally, DeepNull improves upon linear modeling for phenotypic prediction (+23% on average).
View details
DeepNull models non-linear covariate effects to improve phenotypic prediction and association power
Zachary R. Mccaw
Nicholas A. Furlotte
Andrew Carroll
Babak Alipanahi
Nature Communications (2022)
Preview abstract
Genome-wide association studies (GWASs) examine the association between genotype and phenotype while adjusting for a set of covariates. Although the covariates may have non-linear or interactive effects, due to the challenge of specifying the model, GWAS often neglect such terms. Here we introduce DeepNull, a method that identifies and adjusts for non-linear and interactive covariate effects using a deep neural network. In analyses of simulated and real data, we demonstrate that DeepNull maintains tight control of the type I error while increasing statistical power by up to 20% in the presence of non-linear and interactive effects. Moreover, in the absence of such effects, DeepNull incurs no loss of power. When applied to 10 phenotypes from the UK Biobank (n = 370K), DeepNull discovered more hits (+6%) and loci (+7%), on average, than conventional association analyses, many of which are biologically plausible or have previously been reported. Finally, DeepNull improves upon linear modeling for phenotypic prediction (+23% on average).
View details
Large-scale machine learning-based phenotyping significantly improves genomic discovery for optic nerve head morphology
Babak Alipanahi
Babak Behsaz
Zachary Ryan Mccaw
Emanuel Schorsch
D. Sculley
Lizzie Dorfman
Sonia Phene
Andrew Walker Carroll
Anthony Khawaja
American Journal of Human Genetics (2021)
Preview abstract
Genome-wide association studies (GWAS) require accurate cohort phenotyping, but expert labeling can be costly, time-intensive, and variable. Here we develop a machine learning (ML) model to predict glaucomatous features from color fundus photographs. We used the model to predict vertical cup-to-disc ratio (VCDR), a diagnostic parameter and cardinal endophenotype for glaucoma, in 65,680 Europeans in the UK Biobank (UKB). A GWAS of ML-based VCDR identified 299 independent genome-wide significant (GWS; P≤5×10-8) hits in 156 loci. The ML-based GWAS replicated 62 of 65 GWS loci from a recent VCDR GWAS in the UKB for which two ophthalmologists manually labeled images for 67,040 Europeans. The ML-based GWAS also identified 93 novel loci, significantly expanding our understanding of the genetic etiologies of glaucoma and VCDR. Pathway analyses support the biological significance of the novel hits to VCDR, with select loci near genes involved in neuronal and synaptic biology or known to cause severe Mendelian ophthalmic disease. Finally, the ML-based GWAS results significantly improve polygenic prediction of VCDR in independent datasets.
View details
Underspecification Presents Challenges for Credibility in Modern Machine Learning
Dan Moldovan
Ben Adlam
Babak Alipanahi
Alex Beutel
Christina Chen
Jon Deaton
Matthew D. Hoffman
Shaobo Hou
Neil Houlsby
Ghassen Jerfel
Yian Ma
Diana Mincu
Akinori Mitani
Andrea Montanari
Christopher Nielsen
Thomas Osborne
Rajiv Raman
Kim Ramasamy
Jessica Schrouff
Martin Gamunu Seneviratne
Shannon Sequeira
Harini Suresh
Victor Veitch
Steve Yadlowsky
Xiaohua Zhai
D. Sculley
Journal of Machine Learning Research (2020)
Preview abstract
ML models often exhibit unexpectedly poor behavior when they are deployed in real-world domains. We identify underspecification as a key reason for these failures. An ML pipeline is underspecified when it can return many predictors with equivalently strong held-out performance in the training domain. Underspecification is common in modern ML pipelines, such as those based on deep learning. Predictors returned by underspecified pipelines are often treated as equivalent based on their training domain performance, but we show here that such predictors can behave very differently in deployment domains. This ambiguity can lead to instability and poor model behavior in practice, and is a distinct failure mode from previously identified issues arising from structural mismatch between training and deployment domains. We show that this problem appears in a wide variety of practical ML pipelines, using examples from computer vision, medical imaging, natural language processing, clinical risk prediction based on electronic health records, and medical genomics. Our results show the need to explicitly account for underspecification in modeling pipelines that are intended for real-world deployment in any domain.
View details