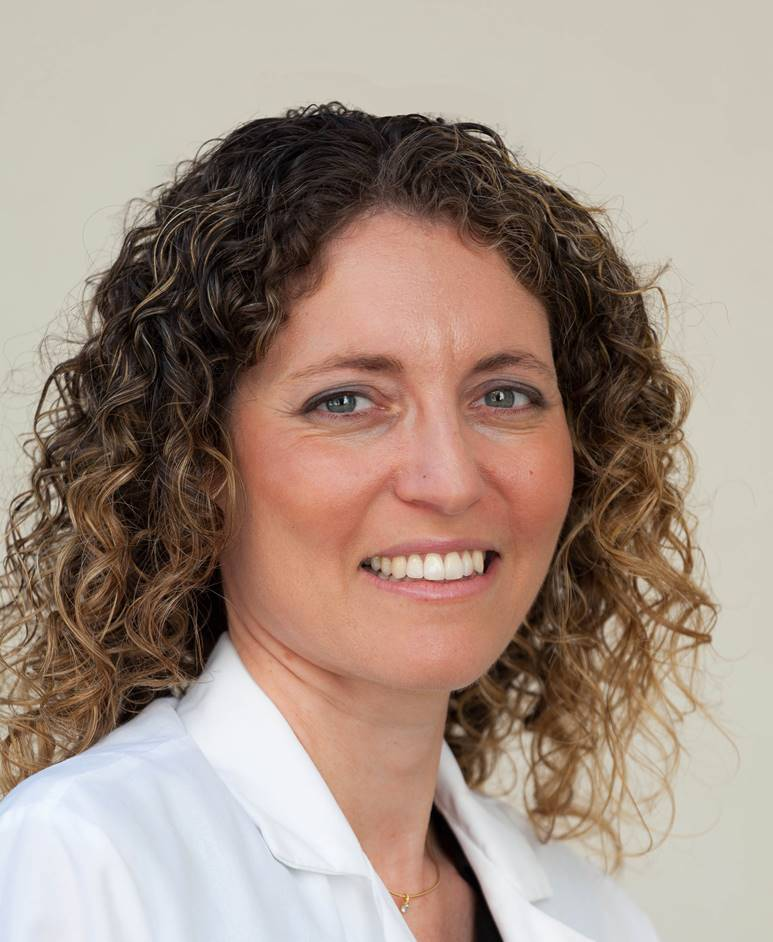
Naama Hammel
Naama is a clinical research scientist in Google Health. In this role she focuses on developing and validating machine learning for medical applications across multiple fields including ophthalmology, dermatology, and more. Naama is an ophthalmologist with a subspecialty in glaucoma. She completed her medical and ophthalmology training at Tel-Aviv University; her glaucoma fellowship at the Shiley Eye Institute, UC San Diego; and her ophthalmic informatics fellowship at the UC Davis Eye Center.
Research Areas
Authored Publications
Sort By
Google
Validation of a Deep Learning Model for Diabetic Retinopathy on Patients with Young-Onset Diabetes
Tony Tan-Torres
Pradeep Praveen
Divleen Jeji
Arthur Brant
Xiang Yin
Lu Yang
Tayyeba Ali
Ilana Traynis
Dushyantsinh Jadeja
Rajroshan Sawhney
Sunny Virmani
Pradeep Venkatesh
Nikhil Tandon
Ophthalmology and Therapy (2025)
Conversational AI in health: Design considerations from a Wizard-of-Oz dermatology case study with users, clinicians and a medical LLM
Brenna Li
Amy Wang
Patricia Strachan
Julie Anne Seguin
Sami Lachgar
Karyn Schroeder
Renee Wong
Extended Abstracts of the 2024 CHI Conference on Human Factors in Computing Systems, Association for Computing Machinery, pp. 10
A deep learning model for novel systemic biomarkers in photographs of the external eye: a retrospective study
Ilana Traynis
Christina Chen
Akib Uddin
Jorge Cuadros
Lauren P. Daskivich
April Y. Maa
Ramasamy Kim
Eugene Yu-Chuan Kang
Lily Peng
Avinash Varadarajan
The Lancet Digital Health (2023)
Beyond Predictions: Explainability and Learning from Machine Learning
Chih-Ying Deng
Akinori Mitani
Christina Chen
Lily Peng
Digital Eye Care and Teleophthalmology, Springer (2023)
Lessons learned from translating AI from development to deployment in healthcare
Sunny Virmani
Jay Nayar
Elin Rønby Pedersen
Divleen Jeji
Lily Peng
Nature Medicine (2023)
Using generative AI to investigate medical imagery models and datasets
Charles Lau
Chloe Nichols
Doron Yaya-Stupp
Heather Cole-Lewis
Ilana Traynis
https://arxiv.org/ (2022)
Detection of signs of disease in external photographs of the eyes via deep learning
Akinori Mitani
Ilana Traynis
Naho Kitade
April Maa
Jorge Cuadros
Lily Hao Yi Peng
Avinash Vaidyanathan Varadarajan
Nature Biomedical Engineering (2022)
Retinal fundus photographs capture hemoglobin loss after blood donation
Akinori Mitani
Ilana Traynis
Lily Hao Yi Peng
Avinash Vaidyanathan Varadarajan
medRxiv (2022)
Deep learning to detect optical coherence tomography-derived diabetic macular edema from retinal photographs: a multicenter validation study
Xinle Sheila Liu
Tayyeba Ali
Ami Shah
Scott Mayer McKinney
Paisan Ruamviboonsuk
Angus W. Turner
Pearse A. Keane
Peranut Chotcomwongse
Variya Nganthavee
Mark Chia
Josef Huemer
Jorge Cuadros
Rajiv Raman
Lily Hao Yi Peng
Avinash Vaidyanathan Varadarajan
Reena Chopra
Ophthalmology Retina (2022)
Iterative quality control strategies for expert medical image labeling
Sonia Phene
Abigail Huang
Rebecca Ackermann
Olga Kanzheleva
Caitlin Taggart
Proceedings of the AAAI Conference on Human Computation and Crowdsourcing (2021)