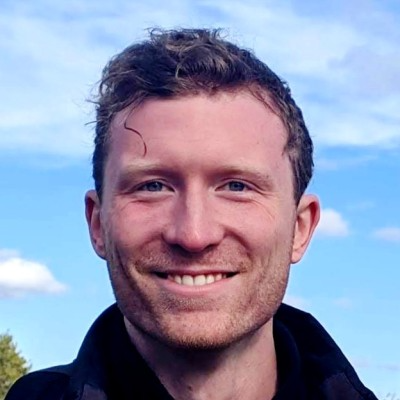
Mike Schaekermann
Authored Publications
Sort By
Google
Generative AI for medical education: Insights from a case study with medical students and an AI tutor for clinical reasoning
Amy Wang
Roma Ruparel
Paul Jhun
Julie Anne Seguin
Patricia Strachan
Renee Wong
2025
Towards Conversational AI for Disease Management
Khaled Saab
David Stutz
Kavita Kulkarni
Sara Mahdavi
Joelle Barral
James Manyika
Ryutaro Tanno
Adam Rodman
arXiv (2025)
Towards Generalist Biomedical AI
Danny Driess
Andrew Carroll
Chuck Lau
Ryutaro Tanno
Ira Ktena
Basil Mustafa
Aakanksha Chowdhery
Simon Kornblith
Philip Mansfield
Sushant Prakash
Renee Wong
Sunny Virmani
Sara Mahdavi
Bradley Green
Ewa Dominowska
Joelle Barral
Karan Singhal
Pete Florence
NEJM AI (2024)
A Toolbox for Surfacing Health Equity Harms and Biases in Large Language Models
Heather Cole-Lewis
Nenad Tomašev
Liam McCoy
Leo Anthony Celi
Alanna Walton
Chirag Nagpal
Akeiylah DeWitt
Philip Mansfield
Sushant Prakash
Joelle Barral
Ivor Horn
Karan Singhal
Nature Medicine (2024)
Conversational AI in health: Design considerations from a Wizard-of-Oz dermatology case study with users, clinicians and a medical LLM
Brenna Li
Amy Wang
Patricia Strachan
Julie Anne Seguin
Sami Lachgar
Karyn Schroeder
Renee Wong
Extended Abstracts of the 2024 CHI Conference on Human Factors in Computing Systems, Association for Computing Machinery, pp. 10
Health equity assessment of machine learning performance (HEAL): a framework and dermatology AI model case study
Terry Spitz
Malcolm Chelliah
Heather Cole-Lewis
Tiam Jaroensri
Geoff Keeling
Stephanie Farquhar
Qinghan Xue
Jenna Lester
Cían Hughes
Patricia Strachan
Fraser Tan
Peggy Bui
Craig Mermel
Lily Peng
Sunny Virmani
Ivor Horn
Cameron Chen
The Lancet eClinicalMedicine (2024)
Towards Conversational Diagnostic AI
Khaled Saab
Jan Freyberg
Ryutaro Tanno
Amy Wang
Brenna Li
Nenad Tomašev
Karan Singhal
Le Hou
Albert Webson
Kavita Kulkarni
Sara Mahdavi
Juro Gottweis
Joelle Barral
Kat Chou
Arxiv (2024) (to appear)
Towards Physician-Level Medical Question Answering with Large Language Models
Karan Singhal
Juro Gottweis
Le Hou
Kevin Clark
Heather Cole-Lewis
Amy Wang
Sami Lachgar
Philip Mansfield
Sushant Prakash
Bradley Green
Ewa Dominowska
Nenad Tomašev
Renee Wong
Sara Mahdavi
Joelle Barral
Arxiv (2023) (to appear)
Towards Accurate Differential Diagnosis with Large Language Models
Daniel McDuff
Amy Wang
Karan Singhal
Yash Sharma
Kavita Kulkarni
Le Hou
Sara Mahdavi
Sushant Prakash
Anupam Pathak
Shwetak Patel
Ewa Dominowska
Juro Gottweis
Joelle Barral
Kat Chou
Jake Sunshine
Arxiv (2023)
Consensus, dissensus and synergy between clinicians and specialist foundation models in radiology report generation
Ryutaro Tanno
David Barrett
Sumedh Ghaisas
Sumanth Dathathri
Abi See
Johannes Welbl
Karan Singhal
Rhys May
Roy Lee
SiWai Man
Zahra Ahmed
Sara Mahdavi
Joelle Barral
Ali Eslami
Danielle Belgrave
Shravya Shetty
Po-Sen Huang
Ira Ktena
Arxiv (2023)