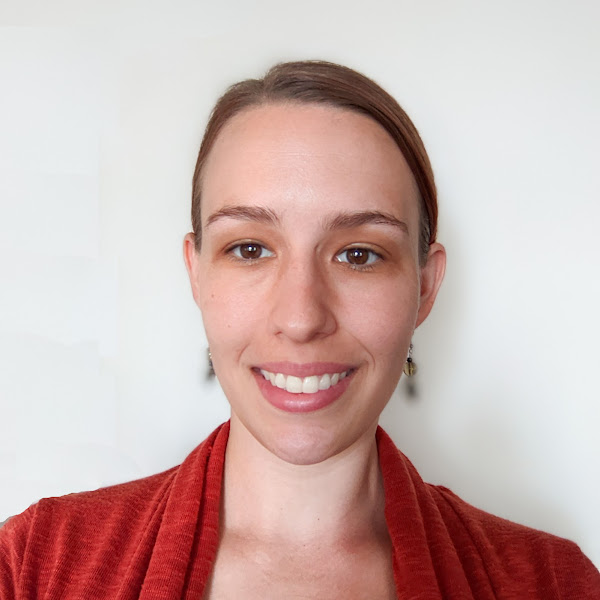
Alicia Vail Parrish
As a research scientist, I use tools from linguistics and psychology to study how we can collect and understand high-quality NLP data. I received my M.A. in linguistics from Michigan State University and my
PhD in Linguistics from New York University.
Research Areas
Authored Publications
Sort By
Google
A Toolbox for Surfacing Health Equity Harms and Biases in Large Language Models
Heather Cole-Lewis
Nenad Tomašev
Liam McCoy
Leo Anthony Celi
Alanna Walton
Chirag Nagpal
Akeiylah DeWitt
Philip Mansfield
Sushant Prakash
Joelle Barral
Ivor Horn
Karan Singhal
Nature Medicine (2024)
DICES Dataset: Diversity in Conversational AI Evaluation for Safety
Alex Taylor
Chris Homan
Greg Serapio-García
NeurIPS2023 (2023)
Intersecting Demographics: Bayesian Multilevel Models Reveal Age, Gender, and Racial Differences in Safety Perception of Chatbot Conversations
Chris Homan
Greg Serapio-García
Alex Taylor
(2023)
All that Agrees Is Not Gold: Evaluating Ground Truth Labels and Dialogue Content for Safety
Chris Homan
Greg Serapio-García
Alex Taylor
(2023)
Adversarial Nibbler: A DataPerf Challenge for Text-to-Image Models
Hannah Kirk
Jessica Quaye
Charvi Rastogi
Max Bartolo
Oana Inel
D. Sculley
Meg Risdal
Will Cukierski
Vijay Reddy
Online (2023)