Responsible AI
The mission of the Responsible AI and Human Centered Technology (RAI-HCT) team is to conduct research and develop methodologies, technologies, and best practices to ensure AI systems are built responsibly.
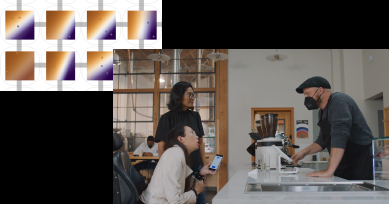
The mission of the Responsible AI and Human Centered Technology (RAI-HCT) team is to conduct research and develop methodologies, technologies, and best practices to ensure AI systems are built responsibly.
About the team
We want to ensure that AI, and its development, have a positive impact on everyone. To meet this goal, we research and develop technology with a human-centered perspective, building tools and processes that put our AI Principles into practice at scale. Working alongside numerous collaborators, including our partner teams and external contributors as we strive to make AI more transparent, fair, and useful to all communities. We also seek to constantly improve the reliability and safety of our entire AI ecosystem.
Our intention is to create a future where technology benefits all users and society.
What we do
- Foundational Research: Build foundational insights and methodologies that define the state-of-the-art of Responsible AI development across the field
- Impact at Google: Collaborate with and contribute to teams across Alphabet to ensure that Google’s products are built following our AI Principles
- Democratize AI: Embed varied cultural contexts and voices in AI development, and empower a broader audience with consistent access, control, and explainability
- Tools and Guidance: Develop tools and technical guidance that can be used by Google, our customers, and the community to test and improve AI products for RAI objectives
Team focus summaries
Featured publications
Highlighted projects
-
Know Your DataAn interactive visualization tool for understanding datasets with the goal of improving data quality and mitigating fairness and bias issues. See the case study for the COCO Captions dataset.
-
More Inclusive People Annotations for Fairness (MIAP)The More Inclusive People Annotations for Fairness (MIAP) collection is a new set of annotations from the 9 million-plus Open Images dataset that uses a new labeling protocol designed to help researchers incorporate fairness analysis into their work.
-
Monk Skin Tone ScaleThe Monk Skin Tone Scale provides a broader spectrum of skin tones that can be used to evaluate datasets and ML models for better representation.
-
ExplorablesInteractive visualizations exploring important concepts in machine learning. "Big ideas in machine learning, simply explained"
-
Language Interpretability ToolAn open-source platform for visualization and understanding of ML models
-
Causality and FairnessMachine learning research that takes a hard look at whether methods are modeling the causal mechanisms we think they are, and when we expect them to be fair.
-
HealthsheetsQualitative evaluation documentation for health datasets designed in consultation with a diverse set of health experts.
-
Societal Context Understanding Tools and Solutions (SCOUTS)Societal Context Understanding Tools and Solutions (SCOUTS) is a Google Research initiative with the mission to provide people and ML systems with the scalable, trustworthy societal context knowledge required to realize responsible and robust AI.
-
Measuring Gendered Correlations in Pre-Trained NLP ModelsA case study looking at correlation related to gender to formulate a series of best practices for using pre-trained language models
-
TensorFlow Model RemediationA library with techniques for addressing bias and fairness issues in ML models.
-
AI for Social GoodA portfolio of projects demonstrating AI’s societal benefit to enable real-world impact.
Some of our people
-
Anton Kast
- General Science
- Mobile Systems
- Software Engineering
-
Jimmy Tobin
- Machine Perception
- Speech Processing
-
Asma Ghandeharioun
- Human-Computer Interaction and Visualization
- Machine Intelligence
- Machine Perception
-
Katrin Tomanek
- Machine Intelligence
- Machine Translation
- Natural Language Processing
-
Nitesh Goyal
- Human-Computer Interaction and Visualization
-
Vinodkumar Prabhakaran
- Information Retrieval and the Web
- Machine Intelligence
- Natural Language Processing
-
James Wexler
- Human-Computer Interaction and Visualization
- Machine Intelligence
-
Courtney Heldreth
- Human-Computer Interaction and Visualization
- Machine Intelligence
-
Parker Barnes
- Machine Intelligence
- Responsible AI
- Security, Privacy and Abuse Prevention
-
Lucas Dixon
- Data Management
- Data Mining and Modeling
- Algorithms and Theory
-
Ian Tenney
- Human-Computer Interaction and Visualization
- Machine Intelligence
- Natural Language Processing
-
Kathy Meier-Hellstern
- Machine Intelligence
- Networking
- Software Engineering
-
Ananth Balashankar
-
Andrew Smart
- Algorithms and Theory
- General Science
- Machine Intelligence
-
Katherine Heller
- Machine Intelligence
- Health & Bioscience
-
Adam Pearce
- Human-Computer Interaction and Visualization
- Machine Intelligence
-
Philip Nelson
- General Science
- Machine Intelligence
-
Preethi Lahoti
- Data Mining and Modeling
- Machine Intelligence
- Natural Language Processing
-
Lora Aroyo
- Human-Computer Interaction and Visualization
- Natural Language Processing
- Responsible AI
-
Donald Martin, Jr.
- Algorithms and Theory
- Data Mining and Modeling
- Human-Computer Interaction and Visualization
-
Remi Denton
- Responsible AI
-
Fernando Diaz
- Information Retrieval and the Web
- Machine Intelligence
- Natural Language Processing
-
Mark Díaz
- Human-Computer Interaction and Visualization
- Responsible AI
-
Ding Wang
- Human-Computer Interaction and Visualization
-
Mahima Pushkarna
- Human-Computer Interaction and Visualization
- Machine Intelligence
- Responsible AI
-
Andrew Zaldivar
- Human-Computer Interaction and Visualization
- Machine Intelligence
-
Pan-Pan Jiang
- Health & Bioscience
-
Sunipa Dev
- Natural Language Processing
- Responsible AI
-
Diana Mincu
- Machine Intelligence
- Health & Bioscience
-
Subhrajit Roy
- Machine Intelligence
- Health & Bioscience
-
Ben Hutchinson
- Data Mining and Modeling
- Machine Intelligence
- Natural Language Processing
-
Dan Liebling
- Human-Computer Interaction and Visualization
- Information Retrieval and the Web
- Machine Learning
-
Jilin Chen
- Data Mining and Modeling
- Human-Computer Interaction and Visualization
- Natural Language Processing
-
Jamila Smith-Loud
- Algorithms and Theory
- General Science
-
Diana Akrong
- Human-Computer Interaction and Visualization
-
Michael Terry
- Human-Computer Interaction and Visualization
- Machine Intelligence