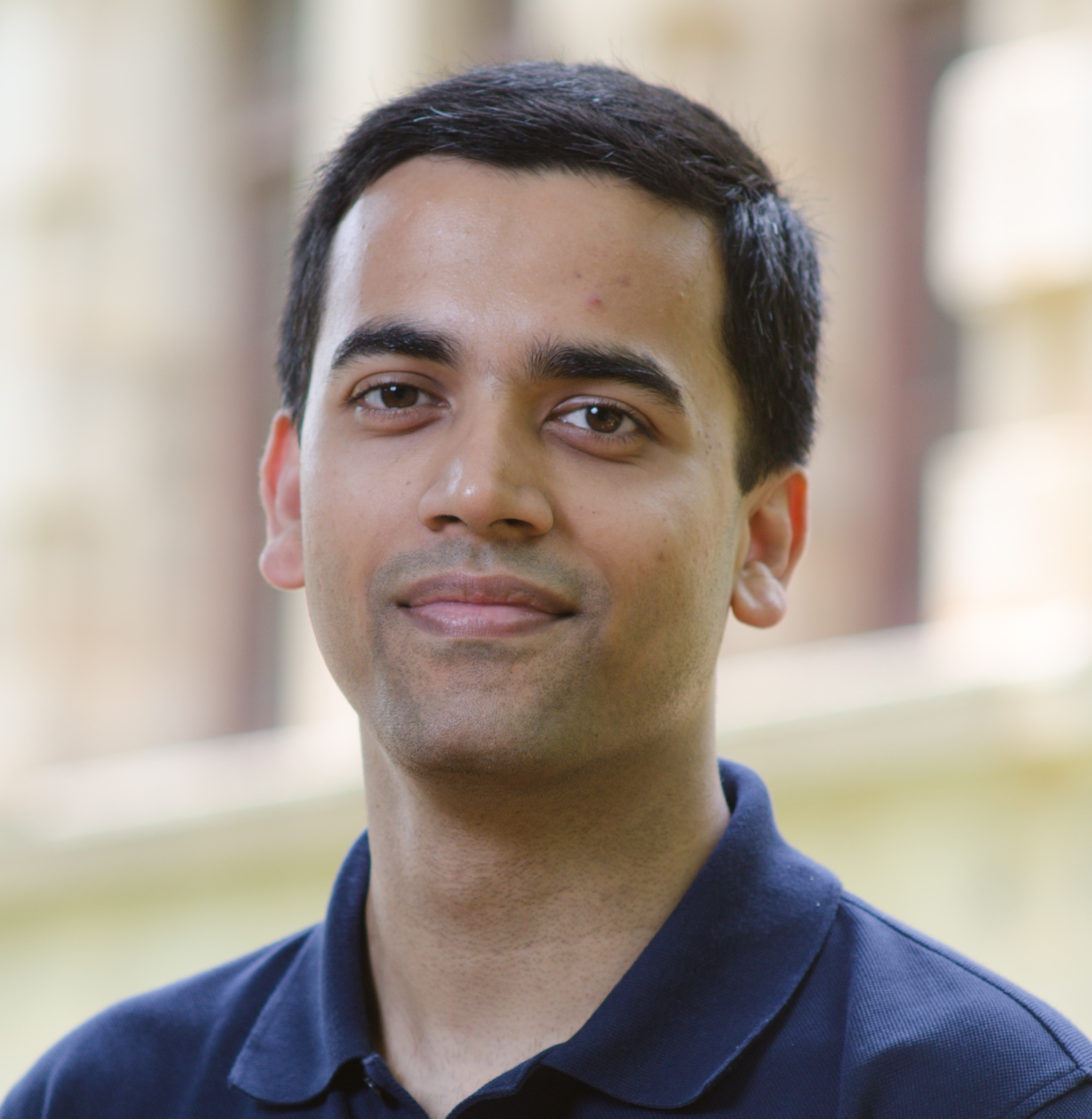
Partha Talukdar
Partha is a Senior Staff Research Scientist at Google Research, Bangalore where he leads a group focused on Natural Language Understanding. He is also an Associate Professor (on leave) at IISc Bangalore. Partha founded KENOME, an enterprise Knowledge graph company with the mission to help enterprises make sense of unstructured data. Previously, Partha was a Postdoctoral Fellow in the Machine Learning Department at Carnegie Mellon University, working with Tom Mitchell on the NELL project. He received his PhD (2010) in CIS from the University of Pennsylvania. Partha is broadly interested in Natural Language Processing, Machine Learning, and Knowledge Graphs. Partha is a recipient of several awards, including an Outstanding Paper Award at ACL 2019 and ACM India Early Career Award 2022. He is a co-author of a book on Graph-based Semi-Supervised Learning. Homepage: https://parthatalukdar.github.io
Research Areas
Authored Publications
Sort By
Google
Multimodal Modeling for Spoken Language Identification
Shikhar Bharadwaj
Sriram (Sri) Ganapathy
Sid Dalmia
Wei Han
Yu Zhang
Proceedings of 2024 IEEE International Conference on Acoustics, Speech and Signal Processing (ICASSP 2024) (2024)
UGIF-DataSet: A New Dataset for Cross-lingual, Cross-modal Sequential actions on the UI
Findings of the Association for Computational Linguistics: NAACL 2024
MASR: Multi-Label Aware Speech Representation
Anjali Raj
Shikhar Bharadwaj
Sriram Ganapathy
2023 Workshop on Automatic Speech Recognition and Understanding (ASRU) (2023)
Bootstrapping Multilingual Semantic Parsers using Large Language Models
Abhijeet Awasthi
Bidisha Samanta
Sunita Sarawagi
Conference of the European Chapter of the Association for Computational Linguistics (EACL) (2023)
Self-influence Guided Data Reweighing for Language Model Pre-training
Megh Thakkar
Sarath Chandar
Sriram (Sri) Ganapathy
EMNLP (2023)
Evaluating Inclusivity, Equity, and Accessibility of NLP Technology: A Case Study for Indian Languages
Simran Khanuja
Sebastian Ruder
Findings of the Association for Computational Linguistics: EACL 2023
Parameter-Efficient Finetuning for Robust Continual Multilingual Learning
Findings of the Association for Computational Linguistics: ACL 2023
Label Aware Speech Representation Learning For Language Identification
Shikhar Bharadwaj
Sriram Ganapathy
Wei Han
Proceedings of Interspeech 2023, pp. 5351-5355
XTREME-UP: A User-Centric Scarce-Data Benchmark for Under-Represented Languages
Sebastian Ruder
Mihir Sanjay Kale
Shruti Rijhwani
Jean-Michel Sarr
Cindy Wang
John Wieting
Christo Kirov
Dana L. Dickinson
Bidisha Samanta
Connie Tao
David Adelani
Reeve Ingle
Dmitry Panteleev
Findings of the Association for Computational Linguistics: EMNLP 2023, Association for Computational Linguistics, Singapore, pp. 1856-1884