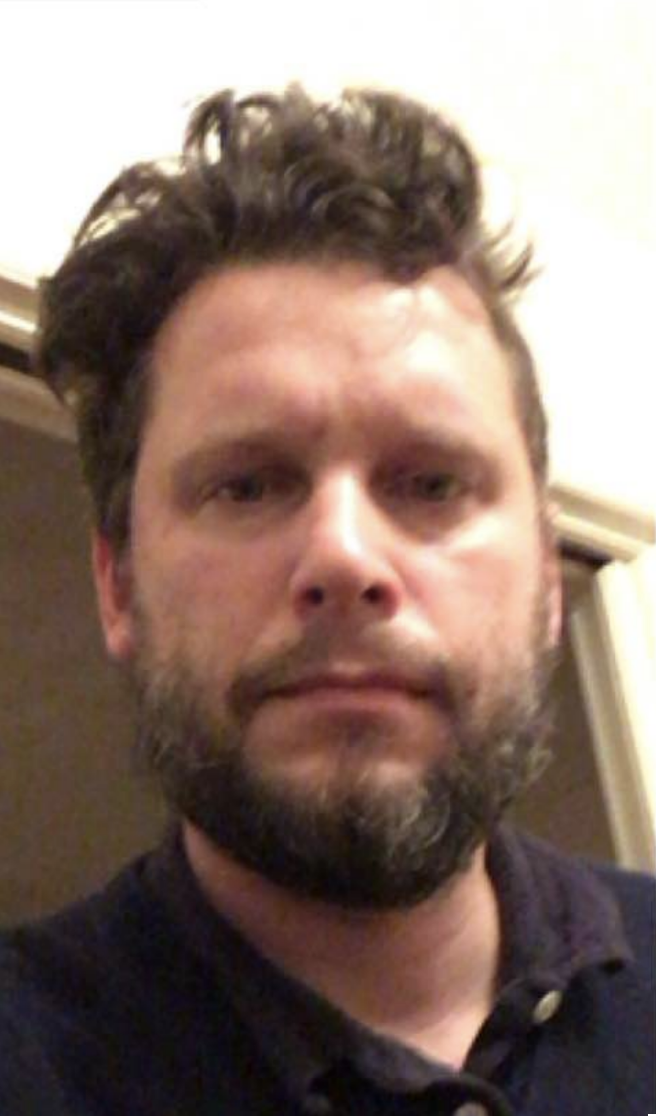
Andrew Smart
I'm a researcher in the Responsible AI Impact Lab working on machine learning fairness and the governance of AI. My background is in anthropology, philosophy, cognitive science and brain imaging. I'm interested in the relationships between social ontology, causality and how to estimate the risks and impacts of using machine learning in high-stakes domains.
Authored Publications
Sort By
Google
Beyond the ML Model: Applying Safety Engineering Frameworks to Text-to-Image Development
Shalaleh Rismani
Renelito Delos Santos
AJung Moon
AIES 2023 (2023)
Identifying Sociotechnical Harms of Algorithmic Systems: Scoping a Taxonomy for Harm Reduction
Shalaleh Rismani
Kathryn Henne
AJung Moon
Paul Nicholas
N'Mah Yilla-Akbari
Jess Gallegos
Emilio Garcia
Gurleen Virk
Proceedings of the 2023 AAAI/ACM Conference on AI, Ethics, and Society, Association for Computing Machinery, 723–741
Healthsheet: development of a transparency artifact for health datasets
Diana Mincu
Lauren Wilcox
Jessica Schrouff
Razvan Adrian Amironesei
Nyalleng Moorosi
ACM FAccT Conference 2022, ACM (2022)
Towards Accountability for Machine Learning Datasets
Alex Hanna
Christina Greer
Margaret Mitchell
Proceedings of FAccT 2021 (2021) (to appear)
The Use and Misuse of Counterfactuals in Ethical Machine Learning
Atoosa Kasirzadeh
FAccT '21: Proceedings of the 2021 ACM Conference on Fairness, Accountability, and TransparencyMarch 2021 (2021)