Publications
Our teams aspire to make discoveries that impact everyone, and core to our approach is sharing our research and tools to fuel progress in the field.
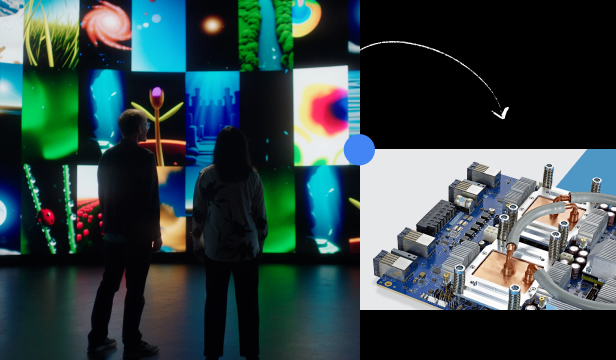
Our teams aspire to make discoveries that impact everyone, and core to our approach is sharing our research and tools to fuel progress in the field.
Sort By
1 - 15 of 10092 publications
Preview abstract
Grounded generation aims to equip language models (LMs) with the ability to produce more credible and accountable responses by accurately citing verifiable sources. However, existing methods, by either feeding LMs with raw or preprocessed materials, remain prone to errors. To address this, we introduce CaLM, a novel verification framework. CaLM leverages the insight that a robust grounded response should be consistent with information derived solely from its cited sources. Our framework empowers smaller LMs, which rely less on parametric memory and excel at processing relevant information given a query, to validate the output of larger LMs. Larger LM responses that closely align with the smaller LMs' output, which relies exclusively on cited documents, are verified. Responses showing discrepancies are iteratively refined through a feedback loop. Experiments on three open-domain question-answering datasets demonstrate significant performance gains of 1.5% to 7% absolute average without any required model fine-tuning.
View details
Preview abstract
Given copies of a quantum state $\rho$, a shadow tomography protocol aims to learn all expectation values from a fixed set of observables, to within a given precision $\epsilon$. We say that a shadow tomography protocol is \textit{triply efficient} if it is sample- and time-efficient, and only employs measurements that entangle a constant number of copies of $\rho$ at a time. The classical shadows protocol based on random single-copy measurements is triply efficient for the set of local Pauli observables. This and other protocols based on random single-copy Clifford measurements can be understood as arising from fractional colorings of a graph $G$ that encodes the commutation structure of the set of observables. Here we describe a framework for two-copy shadow tomography that uses an initial round of Bell measurements to reduce to a fractional coloring problem in an induced subgraph of $G$ with bounded clique number. This coloring problem can be addressed using techniques from graph theory known as \textit{chi-boundedness}. Using this framework we give the first triply efficient shadow tomography scheme for the set of local fermionic observables, which arise in a broad class of interacting fermionic systems in physics and chemistry. We also give a triply efficient scheme for the set of all $n$-qubit Pauli observables. Our protocols for these tasks use two-copy measurements, which is necessary: sample-efficient schemes are provably impossible using only single-copy measurements. Finally, we give a shadow tomography protocol that compresses an $n$-qubit quantum state into a $\poly(n)$-sized classical representation, from which one can extract the expected value of any of the $4^n$ Pauli observables in $\poly(n)$ time, up to a small constant error.
View details
Quantum Computation of Stopping power for Inertial Fusion Target Design
Dominic Berry
Alina Kononov
Alec White
Joonho Lee
Andrew Baczewski
Proceedings of the National Academy of Sciences, 121 (2024), e2317772121
Preview abstract
Stopping power is the rate at which a material absorbs the kinetic energy of a charged particle passing through it - one of many properties needed over a wide range of thermodynamic conditions in modeling inertial fusion implosions. First-principles stopping calculations are classically challenging because they involve the dynamics of large electronic systems far from equilibrium, with accuracies that are particularly difficult to constrain and assess in the warm-dense conditions preceding ignition. Here, we describe a protocol for using a fault-tolerant quantum computer to calculate stopping power from a first-quantized representation of the electrons and projectile. Our approach builds upon the electronic structure block encodings of Su et al. [PRX Quantum 2, 040332 2021], adapting and optimizing those algorithms to estimate observables of interest from the non-Born-Oppenheimer dynamics of multiple particle species at finite temperature. We also work out the constant factors associated with a novel implementation of a high order Trotter approach to simulating a grid representation of these systems. Ultimately, we report logical qubit requirements and leading-order Toffoli costs for computing the stopping power of various projectile/target combinations relevant to interpreting and designing inertial fusion experiments. We estimate that scientifically interesting and classically intractable stopping power calculations can be quantum simulated with
roughly the same number of logical qubits and about one hundred times more Toffoli gates than is required for state-of-the-art quantum simulations of industrially relevant molecules such as FeMoCo or P450.
View details
SkipWriter: LLM-Powered Abbreviated Writing on Tablets
Zheer Xu
Mukund Varma T
Proceedings of UIST 2024 (2024)
Preview abstract
Large Language Models (LLMs) may offer transformative opportunities for text input, especially for physically demanding modalities like handwriting. We studied a form of abbreviated handwriting by designing, developing and evaluating a prototype, named SkipWriter, that convert handwritten strokes of a variable-length, prefix- based abbreviation (e.g., “ho a y” as handwritten strokes) into the intended full phrase (e.g., “how are you” in the digital format) based
on preceding context. SkipWriter consists of an in-production hand-writing recognizer and a LLM fine-tuned on this skip-writing task. With flexible pen input, SkipWriter allows the user to add and revise prefix strokes when predictions don’t match the user’s intent. An user evaluation demonstrated a 60% reduction in motor movements with an average speed of 25.78 WPM. We also showed that this reduction is close to the ceiling of our model in an offline simulation.
View details
Validation of a deep learning system for the detection of diabetic retinopathy in Indigenous Australians
Mark Chia
Fred Hersch
Pearse Keane
Angus Turner
British Journal of Ophthalmology, 108 (2024), pp. 268-273
Preview abstract
Background/aims: Deep learning systems (DLSs) for diabetic retinopathy (DR) detection show promising results but can underperform in racial and ethnic minority groups, therefore external validation within these populations is critical for health equity. This study evaluates the performance of a DLS for DR detection among Indigenous Australians, an understudied ethnic group who suffer disproportionately from DR-related blindness.
Methods: We performed a retrospective external validation study comparing the performance of a DLS against a retinal specialist for the detection of more-than-mild DR (mtmDR), vision-threatening DR (vtDR) and all-cause referable DR. The validation set consisted of 1682 consecutive, single-field, macula-centred retinal photographs from 864 patients with diabetes (mean age 54.9 years, 52.4% women) at an Indigenous primary care service in Perth, Australia. Three-person adjudication by a panel of specialists served as the reference standard.
Results: For mtmDR detection, sensitivity of the DLS was superior to the retina specialist (98.0% (95% CI, 96.5 to 99.4) vs 87.1% (95% CI, 83.6 to 90.6), McNemar’s test p<0.001) with a small reduction in specificity (95.1% (95% CI, 93.6 to 96.4) vs 97.0% (95% CI, 95.9 to 98.0), p=0.006). For vtDR, the DLS’s sensitivity was again superior to the human grader (96.2% (95% CI, 93.4 to 98.6) vs 84.4% (95% CI, 79.7 to 89.2), p<0.001) with a slight drop in specificity (95.8% (95% CI, 94.6 to 96.9) vs 97.8% (95% CI, 96.9 to 98.6), p=0.002). For all-cause referable DR, there was a substantial increase in sensitivity (93.7% (95% CI, 91.8 to 95.5) vs 74.4% (95% CI, 71.1 to 77.5), p<0.001) and a smaller reduction in specificity (91.7% (95% CI, 90.0 to 93.3) vs 96.3% (95% CI, 95.2 to 97.4), p<0.001).
Conclusion: The DLS showed improved sensitivity and similar specificity compared with a retina specialist for DR detection. This demonstrates its potential to support DR screening among Indigenous Australians, an underserved population with a high burden of diabetic eye disease.
View details
Limoncello: Prefetchers for Scale
Carlos Villavieja
Baris Kasikci
Proceedings of the 28th ACM International Conference on Architectural Support for Programming Languages and Operating Systems, Association for Computing Machinery, New York, NY, United States (2024)
Preview abstract
This paper presents Limoncello, a novel software system that dynamically configures data prefetching for high utilization systems. We demonstrate that in resource-constrained environments, such as large data centers, traditional methods of hardware prefetching can increase memory latency and decrease available memory bandwidth. To address this, Limoncello dynamically configures data prefetching, disabling hardware prefetchers when memory bandwidth utilization is high and leveraging targeted software prefetching to reduce cache misses when hardware prefetchers are disabled. Limoncello is software-centric and does not require any modifications to hardware. Our evaluation of the deployment on a real-world hyperscale system reveals that Limoncello unlocks significant performance gains for high-utilization systems: it improves application throughput by 10%, due to a 15% reduction in memory latency, while maintaining minimal change in cache miss rate for targeted library functions.
View details
Preview abstract
We present an approach to modeling an image-space prior on scene motion. Our prior is learned from a collection of motion trajectories extracted from real video sequences depicting natural, oscillatory dynamics such as trees, flowers, candles, and clothes swaying in the wind. We model this dense, long-term motion prior in the Fourier domain:given a single image, our trained model uses a frequency-coordinated diffusion sampling process to predict a spectral volume, which can be converted into a motion texture that spans an entire video. Along with an image-based rendering module, these trajectories can be used for a number of downstream applications, such as turning still images into seamlessly looping videos, or allowing users to realistically interact with objects in real pictures by interpreting the spectral volumes as image-space modal bases, which approximate object dynamics.
View details
"We Need Structured Output": Towards User-centered Constraints on Large Language Model Output
Michael Xieyang Liu
Frederick Liu
Alex Fiannaca
Terry Koo
In Extended Abstract in ACM CHI Conference on Human Factors in Computing Systems (CHI EA '24), ACM (2024), pp. 9 (to appear)
Preview abstract
Large language models can produce creative and diverse responses. However, to integrate them into current developer workflows, it is essential to constrain their outputs to follow specific formats or standards. In this work, we surveyed 51 experienced industry professionals to understand the range of scenarios and motivations driving the need for output constraints from a user-centered perspective. We identified 134 concrete use cases for constraints at two levels: low-level, which ensures the output adhere to a structured format and an appropriate length, and high-level, which requires the output to follow semantic and stylistic guidelines without hallucination. Critically, applying output constraints could not only streamline the currently repetitive process of developing, testing, and integrating LLM prompts for developers, but also enhance the user experience of LLM-powered features and applications. We conclude with a discussion on user preferences and needs towards articulating intended constraints for LLMs, alongside an initial design for a constraint prototyping tool.
View details
Preview abstract
Advances in deep learning systems have allowed large models to match or surpass human accuracy on a number of skills such as image classification, basic programming, and standardized test taking. As the performance of the most capable models begin to saturate on tasks where humans already achieve high accuracy, it becomes necessary to benchmark models on increasingly complex abilities. One such task is forecasting the future outcome of events. In this work we describe experiments using a novel dataset of real world events and associated human predictions, an evaluation metric to measure forecasting ability, and the accuracy of a number of different LLM based forecasting designs on the provided dataset. Additionally, we analyze the performance of the LLM forecasters against human predictions and find that models still struggle to make accurate predictions about the future. Our follow-up experiments indicate this is likely due to models' tendency to guess that most events are unlikely to occur (which tends to be true for many prediction datasets, but does not reflect actual forecasting abilities). We reflect on next steps for developing a systematic and reliable approach to studying LLM forecasting.
View details
A Chain-of-Thought Is as Strong as Its Weakest Link: A Benchmark for Verifiers of Reasoning Chains
Alon Jacovi
Or Honovich
Proceedings of the 62nd Annual Meeting of the Association for Computational Linguistics (2024), pp. 4615–4634
Preview abstract
Prompting language models to provide step-by-step answers (e.g., “Chain-of-Thought”) is the prominent approach for complex reasoning tasks, where more accurate reasoning chains typically improve downstream task performance. Recent literature discusses automatic methods to verify reasoning to evaluate and improve their correctness. However, no fine-grained step-level datasets are available to enable thorough evaluation of such verification methods, hindering progress in this direction. We introduce REVEAL: Reasoning Verification Evaluation, a dataset to benchmark automatic verifiers of complex Chain-of-Thought reasoning in open-domain question-answering settings. REVEAL includes comprehensive labels for the relevance, attribution to evidence passages, and logical correctness of each reasoning step in a language model’s answer, across a variety of datasets and state-of-the-art language models. Evaluation on REVEAL shows that verifiers struggle at verifying reasoning chains — in particular, verifying logical correctness and detecting contradictions. Available at https://reveal-dataset.github.io/.
View details
Preview abstract
Generative AI (GAI) is proliferating, and among its many applications are to support creative work (e.g., generating text, images, music) and to enhance accessibility (e.g., captions of images and audio). As GAI evolves, creatives must consider how (or how not) to incorporate these tools into their practices. In this paper, we present interviews at the intersection of these applications. We learned from 10 creatives with disabilities who intentionally use and do not use GAI in and around their creative work. Their mediums ranged from audio engineering to leatherwork, and they collectively experienced a variety of disabilities, from sensory to motor to invisible disabilities. We share cross-cutting themes of their access hacks, how creative practice and access work become entangled, and their perspectives on how GAI should and should not fit into their workflows. In turn, we offer qualities of accessible creativity with responsible AI that can inform future research.
View details
Perspective Chapter: Assessment of Subjective and Objective Sleep Quality from Wrist-Worn Wearable Data
Ben Yetton
Daniel McDuff
Andrew Barakat
Allen Jiang
Nicholas Allen
Logan Schneider
Ari Winbush
Conor Heneghan
Preview abstract
Researchers are interested in measuring both objective and subjective assessments of sleep, and associated phenomena such as sleepiness, quality and restoration. Predicting perceived sleep quality accurately from objective measurements remains an unsolved and interesting problem. Previous studies using polysomnograms and actigraphy have shown poor concordance between objective metrics and subjective sleep quality, but were often limited by study duration (e.g., one or two nights of PSG, study population in low 100 s). In this chapter, we consider whether consumer sleep trackers could significantly improve the assessment of subjective sleep quality through longer periods of assessment and larger data scale. We describe a recent study that modeled two subjective sleep quality metrics (PROMIS Sleep-Related Impairment (SI) and Sleep Disturbance (SD) Index) from objective sleep metrics acquired from a consumer wearable device (Fitbit). However, the goodness-of-fit parameter remains relatively low, even with the increased data availability and scale of data provided by consumer wearables. Specifically, for a well-characterized normative population of 2106 adults, we see that a linear multivariate model produces an R2 of 0.107 for predicting SI and R2 of 0.147 for SR, consistent with prior results using PSG and actigraphy. We conclude that subjective sleep quality remains broadly a psychological construct that cannot be fully modeled solely by objective sleep metrics.
View details
ViSAGe: A Global-Scale Analysis of Visual Stereotypes in Text-to-Image Generation
Akshita Jha
Sarah Laszlo
Rida Qadri
Chandan Reddy
ACL (2024)
Preview abstract
Recent studies have highlighted the issue of varying degrees of stereotypical depictions for different identity group. However, these existing approaches have several key limitations, including a noticeable lack of coverage of identity groups in their evaluation, and the range of their associated stereotypes. Additionally, these studies often lack a critical distinction between inherently visual stereotypes, such as `brown' or `sombrero', and culturally influenced stereotypes like `kind' or `intelligent'. In this work, we address these limitations by grounding our evaluation of regional, geo-cultural stereotypes in the generated images from Text-to-Image models by leveraging existing textual resources. We employ existing stereotype benchmarks to evaluate stereotypes and focus exclusively on the identification of visual stereotypes within the generated images spanning 135 identity groups. We also compute the offensiveness across identity groups, and check the feasibility of identifying stereotypes automatically. Further, through a detailed case study and quantitative analysis, we reveal how the default representations of all identity groups have a more stereotypical appearance, and for historically marginalized groups, how the images across different attributes are visually more similar than other groups, even when explicitly prompted otherwise.
View details
Preview abstract
Proving mathematical theorems at the olympiad level represents a notable milestone in human-level automated reasoning, owing to their reputed difficulty among the world’s best talents in pre-university mathematics. Current machine-learning approaches, however, are not applicable to most mathematical domains owing to the high cost of translating human proofs into machine-verifiable format. The problem is even worse for geometry because of its unique translation challenges, resulting in severe scarcity of training data. We propose AlphaGeometry, a theorem prover for Euclidean plane geometry that sidesteps the need for human demonstrations by synthesizing millions of theorems and proofs across different levels of complexity. AlphaGeometry is a neuro-symbolic system that uses a neural language model, trained from scratch on our large-scale synthetic data, to guide a symbolic deduction engine through infinite branching points in challenging problems. On a test set of 30 latest olympiad-level problems, AlphaGeometry solves 25, outperforming the previous best method that only solves ten problems and approaching the performance of an average International Mathematical Olympiad (IMO) gold medallist. Notably, AlphaGeometry produces human-readable proofs, solves all geometry problems in the IMO 2000 and 2015 under human expert evaluation and discovers a generalized version of a translated IMO theorem in 2004.
View details
Analysis of objective and subjective sleep metrics and smartphone usage patterns
Conor Heneghan
Daniel McDuff
Ari Winbush
Nicholas Allen
John Hernandez
Allen Jiang
Andrew Barakat
Logan Schneider
Benjamin Nelson
Ben Yetton
Preview abstract
Analysis of objective and subjective sleep metrics and smartphone usage patterns
Conor Heneghan, , Daniel McDuff, Ari Winbush, Nicholas Allen, John Hernandez, Allen Jiang,, Andrew Barakat, Logan Schneider, Benjamin Nelson, Ben Yetton
Consumer Health Research Team, Google Inc.
Department of Psychology, University of Oregon
Verily Life Sciences
Department of Psychiatry, Harvard Medical School and Beth Israel Deaconess Medical Center
Introduction: The Digital Wellbeing Study is an IRB approved joint study between the University of Oregon and Google to investigate how smartphone usage interacts with objective and
subjective parameters of well-being such as sleep, exercise and stress. The study recruited a demographically diverse population who each wore a smartwatch and installed a smartphone app linked to the study. Participants completed demographic and health questionnaires including the PROMIS Sleep Disturbance (SD) Short Form. Aims of the study included (a) whether objective sleep duration was correlated with smartphone use, and (b) whether smartphone usage could predict the subjective self reported sleep instrument.
Methods: There was sufficient data from 7,499 users to conduct a population modeling analysis. An Ordinary Least Squares linear model was used as a predictor of each subject’s average total sleep time (TST) and their SD t-score. The inputs to the model included demographics, and population z-scored activity measures (steps, sedentary time, time driving, time at work, home and other locations, phone screen time, frequency of phone unlocks)
over seven days prior to the survey.
Results: The activity measures and baseline demographics could only explain a small amount of the overall variance in TST and SD (R^2=0.04 for TST and R^2=0.05 for SD). Phone screen
time was a statistically significant predictor of both TST (-8.19 mins, p< 0.001) and self-reported sleep disruption (0.611 t-score units, p< 0.001). The number of phone unlocks was a predictor of variability in TST (-3.33 mins, p< 0.001) suggesting that longer session times are correlated with greater TST variability. The effects are minimal (e.g., a subject who has one standard
deviation greater phone screen time than average would be predicted to only see a 2% reduction in TST, and a 0.6% increase in perceived sleep disturbance). Time driving and step count were
also minor predictors of SD and TST.
Conclusion: At a population level, average activity measures from wearables and smartphones such as steps, smartphone usage time, sedentary activity etc. are limited predictors of
objective sleep metrics such as Total Sleep Time, and subjective sleep metrics such as the PROMIS Sleep Disturbance t-score.
Support (if any): This research was funded by Google Inc.
View details