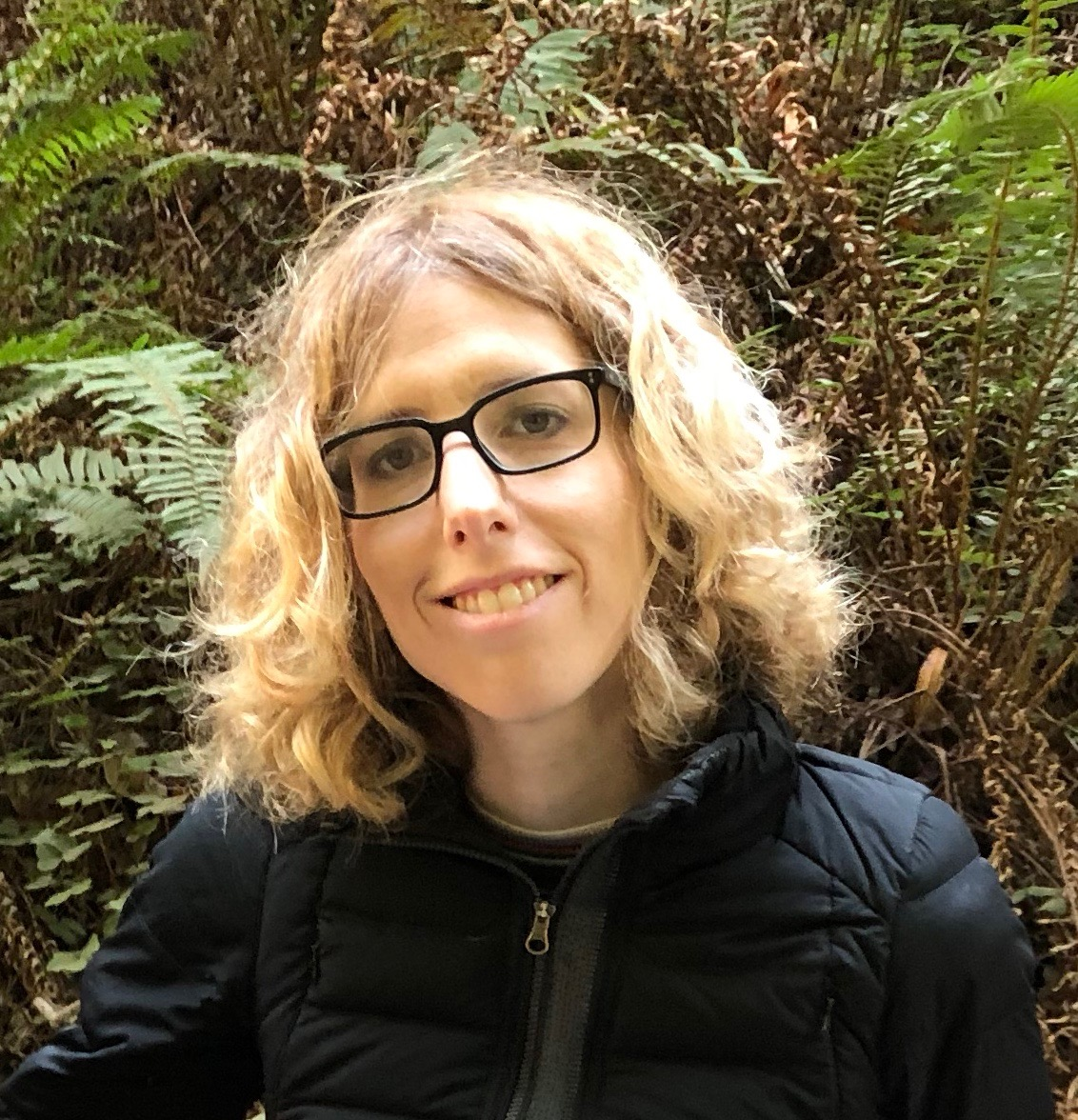
Katherine Heller
Katherine is a research scientist in Responsible AI at Google Research, and a member of Context in AI Research (CAIR) team. She works on Machine Learning (ML) research in Healthcare, Vision, Language, and Creativity, focusing on incorporating values for Transparency, Inclusivity, Fairness, and Robustness in our research. Prior to joining Google, she was Statistical Science faculty at Duke University, where she developed a sepsis detection system now in use at Duke University Hospital, and a nationally released iOS app which tries to complete the picture of peoples' Multiple Sclerosis course between clinic visits.
Katherine received a BS in CS and Applied Math from SUNY Stony Brook, an MS in CS from Columbia University, and a PhD in Machine Learning from the Gatsby Computational Neuroscience Unity at UCL. She was then a postdoc on an EPSRC fellowship in Engineering at the University of Cambridge, and an NSF postoc fellow in Brain and Cognitive Sciences at MIT.
Research Areas
Authored Publications
Sort By
A Toolbox for Surfacing Health Equity Harms and Biases in Large Language Models
Heather Cole-Lewis
Nenad Tomašev
Liam McCoy
Leo Anthony Celi
Alanna Walton
Akeiylah DeWitt
Philip Mansfield
Sushant Prakash
Joelle Barral
Ivor Horn
Karan Singhal
Nature Medicine (2024)
Preview abstract
Large language models (LLMs) hold promise to serve complex health information needs but also have the potential to introduce harm and exacerbate health disparities. Reliably evaluating equity-related model failures is a critical step toward developing systems that promote health equity. We present resources and methodologies for surfacing biases with potential to precipitate equity-related harms in long-form, LLM-generated answers to medical questions and conduct a large-scale empirical case study with the Med-PaLM 2 LLM. Our contributions include a multifactorial framework for human assessment of LLM-generated answers for biases and EquityMedQA, a collection of seven datasets enriched for adversarial queries. Both our human assessment framework and our dataset design process are grounded in an iterative participatory approach and review of Med-PaLM 2 answers. Through our empirical study, we find that our approach surfaces biases that may be missed by narrower evaluation approaches. Our experience underscores the importance of using diverse assessment methodologies and involving raters of varying backgrounds and expertise. While our approach is not sufficient to holistically assess whether the deployment of an artificial intelligence (AI) system promotes equitable health outcomes, we hope that it can be leveraged and built upon toward a shared goal of LLMs that promote accessible and equitable healthcare.
View details
The Case for Globalizing Fairness: A Mixed Methods Study on the Perceptions of Colonialism, AI and Health in Africa
Iskandar Haykel
Aisha Walcott-Bryant
Sanmi Koyejo
Preview abstract
With growing machine learning (ML) and large language model applications in healthcare, there have been calls for fairness in ML to understand and mitigate ethical concerns these systems may pose. Fairness has implications for health in Africa, which already has inequitable power imbalances between the Global North and South. This paper seeks to explore fairness for global health, with Africa as a case study.
We conduct a scoping review to propose fairness attributes for consideration in the African context and delineate where they may come into play in different ML-enabled medical modalities. We then conduct qualitative research studies with 625 general population study participants in 5 countries in Africa and 28 experts in ML, Health, and/or policy focussed on Africa to obtain feedback on the proposed attributes. We delve specifically into understanding the interplay between AI, health and colonialism.
Our findings demonstrate that among experts there is a general mistrust that technologies that are solely developed by former colonizers can benefit Africans, and that associated resource constraints due to pre-existing economic and infrastructure inequities can be linked to colonialism. General population survey responses found about an average of 40% of people associate an undercurrent of colonialism to AI and this was most dominant amongst participants from South Africa. However the majority of the general population participants surveyed did not think there was a direct link between AI and colonialism.Colonial history, country of origin, National income level were specific axes of disparities that participants felt would cause an AI tool to be biased
This work serves as a basis for policy development around Artificial Intelligence for health in Africa and can be expanded to other regions.
View details
Batch Calibration: Rethinking Calibration For In-Context Learning And Prompt Engineering
Lev Proleev
Diana Mincu
International Conference on Learning Representations (ICLR) (2024)
Preview abstract
Prompting and in-context learning (ICL) have become efficient learning paradigms for large language models (LLMs). However, LLMs suffer from prompt brittleness and various bias factors in the prompt, including but not limited to the formatting, the choice verbalizers, and the ICL examples. To address this problem that results in unexpected performance degradation, calibration methods have been developed to mitigate the effects of these biases while recovering LLM performance. In this work, we first conduct a systematic analysis of the existing calibration methods, where we both provide a unified view and reveal the failure cases. Inspired by these analyses, we propose Batch Calibration (BC), a simple yet intuitive method that controls the contextual bias from the batched input, unifies various prior approaches, and effectively addresses the aforementioned issues. BC is zero-shot, inference-only, and incurs negligible additional costs. In the few-shot setup, we further extend BC to allow it to learn the contextual bias from labeled data. We validate the effectiveness of BC with PaLM 2-(S, M, L) and CLIP models and demonstrate state-of-the-art performance over previous calibration baselines across more than 10 natural language understanding and image classification tasks.
View details
TRINDs: Assessing the Diagnostic Capabilities of Large Language Models for Tropical and Infectious Diseases
Nenad Tomašev
Chintan Ghate
Steve Adudans
Oluwatosin Akande
Sylvanus Aitkins
Geoffrey Siwo
Lynda Osadebe
Eric Ndombi
Preview abstract
Neglected tropical diseases (NTDs) and infectious diseases disproportionately affect the poorest regions of the world. While large language models (LLMs) have shown promise for medical question answering, there is limited work focused on tropical and infectious disease-specific explorations. We introduce TRINDs, a dataset of 52 tropical and infectious diseases with demographic and semantic clinical and consumer augmentations. We evaluate various context and counterfactual locations to understand their influence on LLM performance. Results show that LLMs perform best when provided with contextual information such as demographics, location, and symptoms. We also develop TRINDs-LM, a tool that enables users to enter symptoms and contextual information to receive a most likely diagnosis. In addition to the LLM evaluations, we also conducted a human expert baseline study to assess the accuracy of human experts in diagnosing tropical and infectious diseases with 7 medical and public health experts. This work demonstrates methods for creating and evaluating datasets for testing and optimizing LLMs, and the use of a tool that could improve digital diagnosis and tracking of NTDs.
View details
Nteasee: A qualitative study of expert and general population perspectives on deploying AI for health in African countries
Iskandar Haykel
Kerrie Kauer
Florence Ofori
Tousif Ahmad
Preview abstract
Background: Artificial Intelligence for health has the potential to significantly change and improve healthcare. However in most African countries identifying culturally and contextually attuned approaches for deploying these solutions is not well understood. To bridge this gap, we conduct a qualitative study to investigate the best practices, fairness indicators and potential biases to mitigate when deploying AI for health in African countries, as well as explore opportunities where artificial intelligence could make a positive impact in health.
Methods: We used a mixed methods approach combining in-depth interviews (IDIs) and surveys. We conduct 1.5-2 hour long IDIs with 50 experts in health, policy and AI across 17 countries, and through an inductive approach we conduct a qualitative thematic analysis on expert IDI responses. We administer a blinded 30-minute survey with thought-cases to 672 general population participants across 5 countries in Africa (Ghana, South Africa, Rwanda, Kenya and Nigeria), and analyze responses on quantitative scales, statistically comparing responses by country, age, gender, and level of familiarity with AI. We thematically summarize open-ended responses from surveys.
Results and Conclusion: Our results find generally positive attitudes, high levels of trust, accompanied by moderate levels of concern among general population participants for AI usage for health in Africa. This contrasts with expert responses, where major themes revolved around trust/mistrust, AI ethics concerns, and systemic barriers to overcome, among others. This work presents the first-of-its-kind qualitative research study of the potential of AI for health in Africa with perspectives from both experts and the general population. We hope that this work guides policy makers and drives home the need for education and the inclusion of general population perspectives in decision-making around AI usage.
View details
The Case for Globalizing Fairness: A Mixed Methods Study on the Perceptions of Colonialism, AI and Health in Africa
Iskandar Haykel
Aisha Walcott-Bryant
Sanmi Koyejo
Preview abstract
With growing machine learning (ML) and large language model applications in healthcare, there have been calls for fairness in ML to understand and mitigate ethical concerns these systems may pose. Fairness has implications for health in Africa, which already has inequitable power imbalances between the Global North and South. This paper seeks to explore fairness for global health, with Africa as a case study.
We conduct a scoping review to propose fairness attributes for consideration in the African context and delineate where they may come into play in different ML-enabled medical modalities. We then conduct qualitative research studies with 625 general population study participants in 5 countries in Africa and 28 experts in ML, Health, and/or policy focussed on Africa to obtain feedback on the proposed attributes. We delve specifically into understanding the interplay between AI, health and colonialism.
Our findings demonstrate that among experts there is a general mistrust that technologies that are solely developed by former colonizers can benefit Africans, and that associated resource constraints due to pre-existing economic and infrastructure inequities can be linked to colonialism. General population survey responses found about an average of 40% of people associate an undercurrent of colonialism to AI and this was most dominant amongst participants from South Africa. However the majority of the general population participants surveyed did not think there was a direct link between AI and colonialism.Colonial history, country of origin, National income level were specific axes of disparities that participants felt would cause an AI tool to be biased
This work serves as a basis for policy development around Artificial Intelligence for health in Africa and can be expanded to other regions.
View details
Preview abstract
As machine learning (ML) systems see far-reaching applications in healthcare, there have been calls for fairness in machine learning to understand and mitigate ethical concerns these systems may pose. Fairness has thus far mostly been defined from a Western lens, and has implications for global health in Africa, which already has inequitable power imbalances between the Global North and South. This paper seeks to explore fairness for global health, with Africa as a case study. We propose fairness attributes for consideration in the African context and delineate where they may come into play in different ML-enabled medical modalities. This serves as a basis and call for action for furthering research into fairness in global health.
View details
Preview abstract
As machine learning (ML) systems see far-reaching applications in healthcare, there have been calls for fairness in machine learning to understand and mitigate ethical concerns these systems may pose. Fairness has thus far mostly been defined from a Western lens, and has implications for global health in Africa, which already has inequitable power imbalances between the Global North and South. This paper seeks to explore fairness for global health, with Africa as a case study. We propose fairness attributes for consideration in the African context and delineate where they may come into play in different ML-enabled medical modalities. This serves as a basis and call for action for furthering research into fairness in global health.
View details
Healthsheet: development of a transparency artifact for health datasets
Diana Mincu
Lauren Wilcox
Razvan Adrian Amironesei
Nyalleng Moorosi
ACM FAccT Conference 2022, ACM (2022)
Preview abstract
Machine learning (ML) approaches have demonstrated promising results in a wide range of healthcare applications. Data plays a crucial role in developing ML-based healthcare systems that directly affect people’s lives. Many of the ethical issues surrounding the use of ML in healthcare stem from structural inequalities underlying the way we collect, use, and handle data. Developing guidelines to improve documentation practices regarding the creation, use, and maintenance of ML healthcare datasets is therefore of critical importance. In this work, we introduce Healthsheet, a contextualized adaptation of the original datasheet questionnaire for health-specific applications. Through a series of semi-structured interviews, we adapt the datasheets for healthcare data documentation. As part of the Healthsheet development process and to understand the obstacles researchers face in creating datasheets, we worked with three publicly-available healthcare datasets as our case studies, each with different types of structured data: Electronic health Records (EHR), clinical trial study data, and smartphone-based performance outcome measures. Our findings from the interviewee study and case studies show 1) that datasheets should be contextualized for healthcare, 2) that despite incentives to adopt accountability practices such as datasheets, there is a lack of consistency in the broader use of these practices 3) how the ML for health community views datasheets and particularly Healthsheets as diagnostic tool to surface the limitations and strength of datasets and 4) the relative importance of different fields in the datasheet to healthcare concerns.
View details
Preview abstract
In order to build trust that a machine learned model is appropriate and responsible within a systems context involving technical and
human components, a broad range of factors typically need to be considered. However in practice model evaluations frequently focus
on only a narrow range of expected predictive behaviours. This paper examines the critical evaluation gap between the idealized
breadth of concerns and the observed narrow focus of actual evaluations. In doing so, we demonstrate which values are centered—and
which are marginalized—within the machine learning community. Through an empirical study of machine learning papers from
recent high profile conferences, we demonstrate the discipline’s general focus on a small set of evaluation methods. By considering the
mathematical formulations of evaluation metrics and the test datasets over which they are calculated, we draw attention to which
properties of models are centered in the field. This analysis also reveals an important gap: the properties of models which are frequently
neglected or sidelined during evaluation. By studying the structure of this gap, we demonstrate the machine learning discipline’s
implicit assumption of a range of commitments which have normative impacts; these include commitments to consequentialism,
abstractability from context, the quantifiability of impacts, the irrelevance of non-predictive features, and the equivalence of different
failure modes. Shedding light on these assumptions and commitments enables us to question their appropriateness for different ML
system contexts, and points the way towards more diverse and contextualized evaluation methodologies which can be used to more
robustly examine the trustworthiness of ML models.
View details