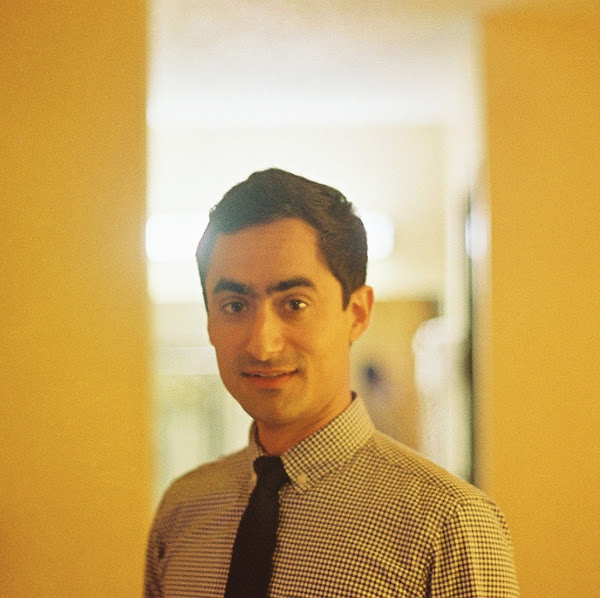
Afshin Rostamizadeh
Afshin is a research scientist at Google Research NY, where he specializes in designing and applying machine learning algorithms. He received his BS in Electrical Engineering and Computer Science from UC Berkeley, his PhD in Computer Science from the Courant Institute at NYU with advisor Mehryar Mohri and was a post-doc at UC Berkeley in Peter Bartlett's group.
He has worked on problems such as learning from non-iid samples, learning from biased samples, learning from data with missing features and automatic kernel selection for kernelized algorithms such as SVM.
Authored Publications
Sort By
DistillSpec: Improving speculative decoding via knowledge distillation
Yongchao Zhou
Kaifeng Lyu
Aditya Menon
Jean-François Kagy
International Conference on Learning Representations (ICLR) (2024)
Preview abstract
Speculative decoding proves highly effective in expediting Large Language Model inference by employing a smaller draft model for token generation and a larger model for parallel token verification. Nonetheless, identifying an accurate and compact draft model aligned with the target model presents challenges. To address this, we propose leveraging white-box knowledge distillation, significantly improving draft model alignment with the larger target model, thereby enhancing speculative decoding. Our findings underscore the pivotal role of on-policy data generation and a suitable divergence function tailored to the task and decoding scheme for successful distillation. In practice, our refined distillation approach yields 20\% speedup over standard speculative decoding across five distinct tasks, using both greedy decoding and temperature sampling. Furthermore, we extend the concept of lossless speculative decoding to incorporate a lenience factor in the rejection sampling step, offering fine-grained control over the trade-off between quality and latency in lossy decoding. Finally, adopting a strategy of "distilling for performance first and distillation for speculative decoding second" enables a remarkable 8x reduction in latency with minimal performance compromise, compared to no distillation and speculative decoding baseline.
View details
Preview abstract
In real-world systems, models are frequently updated as more data becomes available, and in addition to achieving high accuracy, the goal is to also maintain a low difference in predictions compared to the base model (i.e. predictive churn). If model retraining results in vastly different behavior, then it could cause negative effects in downstream systems, especially if this churn can be avoided with limited impact on model accuracy. In this paper, we show an equivalence between training with distillation using the base model as the teacher and training with an explicit constraint on the predictive churn. We then show that distillation performs strongly for low churn training against a number of recent baselines on a wide range of datasets and model architectures, including fully-connected networks, convolutional networks, and transformers.
View details
Preview abstract
Search engines including Google are beginning to support local-dining queries such as ``At which nearby restaurants can I order the Indonesian salad \textit{gado-gado}?''.
Given the low coverage of online menus worldwide, and only 30\% even having a website, this remains a challenge.
Here we leverage the power of the crowd: online users who are willing to answer questions about dish availability at restaurants visited.
While motivated users are happy to contribute knowledge for free, they are much less likely to respond to ``silly'' or embarrassing questions (e.g., ``Does \textit{Pizza Hut} serve pizza?'' or ``Does \textit{Mike's Vegan Restaurant} serve hamburgers?'')
In this paper, we study the problem of \textit{Vexation-Aware Active Learning}, where judiciously selected questions are targeted towards improving restaurant-dish model prediction, subject to a limit on the percentage of ``unsure'' answers or ``dismissals'' (e.g., swiping the app closed) used to measure vexation.
We formalize the problem as an integer linear program and solve it efficiently using a distributed solution that scales linearly with the number of candidate questions.
Since our algorithm relies on precise estimation of the unsure-dismiss rate (UDR), we give a regression model that provides accurate results compared to baselines including collaborative filtering.
Finally, we demonstrate in a live system that our proposed vexation-aware strategy performs competitively against classical (margin-based) active learning approaches while not exceeding UDR bounds.
View details
Preview abstract
Federated learning is typically approached as a distributed optimization problem, where the goal is to minimize a global loss function by distributing computation across many client devices that possess local data and specify different parts of the global objective. We present an alternative perspective and formulate federated learning as inference of the global posterior distribution over model parameters. While exact inference is often intractable, this perspective provides a consistent way to search for global optima in federated settings. Further, starting with the analysis of federated quadratic objectives, we develop a computation- and communication-efficient approximate posterior inference algorithm---\emph{federated posterior averaging} (\FedPA). Our algorithm uses MCMC for approximate inference of local posteriors on the clients and efficiently communicates their statistics to the server, where the latter uses them to iteratively refine the global estimate of the posterior mode. Finally, we show that \FedPA generalizes federated averaging (\FedAvg), can similarly benefit from adaptive optimizers, and yields state-of-the-art results on four realistic and challenging benchmarks, converging faster, to better optima.
View details
Batch Active Learning at Scale
Anand Rajagopalan
Gui Citovsky
Laz Karydas
NeurIPS 2021
Preview abstract
The ability to train complex and highly effective models often requires an abundance of training data, which can easily become a bottleneck in cost, time, and computational resources. Batch active learning, which adaptively issues batched queries to a labeling oracle, is a common approach for addressing this problem. The practical benefits of batch sampling come with the downside of less adaptivity and the risk of sampling redundant examples within a batch -- a risk that grows with the batch size. In this work, we analyze an efficient active learning algorithm, which focuses on the large batch setting. In particular, we show that our sampling method, which combines notions of uncertainty and diversity, easily scales to batch sizes (100K-1M) several orders of magnitude larger than used in previous studies and provides significant improvements in model training efficiency compared to recent baselines.
View details
Preview abstract
Consider a setting where we wish to automate an expensive task with a machine
learning algorithm using a limited labeling resource. In such settings, examples
routed for labeling are often out of scope for the machine learning algorithm. For
example, in a spam detection setting, human reviewers not only provide labeled
data but are such high-quality detectors of spam that examples routed to them no
longer require machine evaluation. A consequence is that distribution of examples
routed to the machine is intimately tied to the process generating labels. We
introduce a formalization of this setting, and give an algorithm that simultaneously
learns a model and decides when to request a label by leveraging ideas from both
the abstention and active learning literatures. We prove an upper bound on the
algorithm’s label complexity and a matching lower bound for any algorithm in this
setting. We conduct a thorough set of experiments including an ablation study to
test different components of our algorithm. We demonstrate the effectiveness of an
efficient version of our algorithm over margin sampling on a variety of datasets.
View details
Understanding the Effects of Batching in Online Active Learning
Proceedings of the Twenty Third International Conference on Artificial Intelligence and Statistics (2020)
Preview abstract
Online active learning (AL) algorithms often assume immediate access to a label once a query has been made. However, due to practical constraints, the labels of these queried examples are generally only available in ``batches''. In this work, we present a novel analysis for a generic class of batch online AL algorithms and reveal that the effects of batching are in fact mild and only result in an additional term in the label complexity that is linear in the batch size. To our knowledge, this provides the first theoretical justification for such algorithms and we show how they can be applied to batch variants of three canonical online AL algorithms: IWAL, ORIWAL, and DHM. We also conduct an empirical study that corroborates the novel theoretical insights.
View details
A System for Massively Parallel Hyperparameter Tuning
Liam Li
Kevin Jamieson
Ekaterina Gonina
Jonathan Ben-tzur
Moritz Hardt
Benjamin Recht
Ameet Talwalkar
Third Conference on Systems and Machine Learning (2020) (to appear)
Preview abstract
Modern learning models are characterized by large hyperparameter spaces and long training times; this coupled
with the rise of parallel computing and productionization of machine learning motivate developing production-
quality hyperparameter optimization functionality for a distributed computing setting. We address this challenge
with a simple and robust hyperparameter optimization algorithm ASHA, which exploits parallelism and aggressive
early-stopping to tackle large-scale hyperparameter optimization problems. Our extensive empirical results show
that ASHA outperforms state-of-the-art hyperparameter optimization methods; scales linearly with the number of
workers in distributed settings; and is suitable for massive parallelism, converging to a high quality configuration
in half the time taken by Vizier (Google’s internal hyperparameter optimization service) in an experiment with
500 workers. We end with a discussion of the systems considerations we encountered and our associated solutions
when implementing ASHA in SystemX, a production-quality service for hyperparameter tuning.
View details
An Analysis of SVD for Deep Rotation Estimation
Jake Levinson
Arthur Chen
Angjoo Kanazawa
Advances in Neural Information Processing Systems (NeurIPS) 2020
Preview abstract
Symmetric orthogonalization via SVD, and closely related procedures, are well-known techniques for projecting matrices onto O(n) or SO(n). These tools have long been used for applications in computer vision, for example optimal 3D alignment problems solved by orthogonal Procrustes, rotation averaging, or Essential matrix decomposition. Despite its utility in different settings, SVD orthogonalization as a procedure for producing rotation matrices is typically overlooked in deep learning models, where the preferences tend toward classic representations like unit quaternions, Euler angles, and axis-angle, or more recently-introduced methods. Despite the importance of 3D rotations in computer vision and robotics, a single universally effective representation is still missing. Here, we explore the viability of SVD orthogonalization for 3D rotations in neural networks. We present a theoretical analysis of SVD as used for projection onto the rotation group. Our extensive quantitative analysis shows simply replacing existing representations with the SVD orthogonalization procedure obtains state of the art performance in many deep learning applications covering both supervised and unsupervised training.
View details
Categorical Feature Compression via Submodular Optimization
Lin Chen
International Conference on Machine Learning (2019), pp. 515-523
Preview abstract
In modern machine learning tasks, the presence of categorical features with extremely large vocabularies is a reality. This becomes a bottleneck when using an ML model, which generally grows at least linearly with the vocabulary size, affecting the memory, training and inference costs, as well as overfitting risk. In this work, we seek to compress the vocabulary by maximizing the mutual information between the compressed categorical feature and the target binary labels. We note the relationship of this problem to that of quantization in a discrete memoryless channel, where there exists a quadratic-time algorithm to solve the problem. Unfortunately, such an algorithm does not scale to data sets with massive vocabularies and, in this paper, we develop a distributed quasi-linear O(n log n) algorithm with provable approximation guarantees. We first observe that although entropy is a submodular function, this is not the case for mutual information between a categorical feature and label. To tackle this problem, we define a set function over a different space, which still contains the optimal solution, and prove this function is submodular. We also provide a query oracle to the submodular function that runs in amortized logarithmic time, and is easy to compute in a distributed fashion. Combining these results with a greedy algorithm allows us to achieve a (1-1/e)-approximation in quasi-linear time. Finally, we compare our proposed algorithm to several existing approaches using the large-scale Criteo learning task and demonstrate better performance in retaining mutual information and also verify the learning performance of the compressed vocabulary.
View details