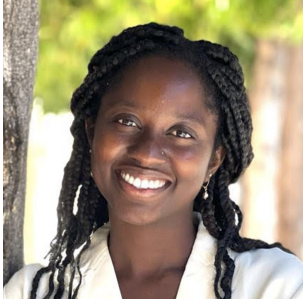
Mercy Nyamewaa Asiedu
I am a senior research scientist at Google Research, and my research interests lie in developing robust machine learning algorithms and Large Language Models for health. Before Google, I was a Schmidt Science Fellow at MIT, where I worked on different machine learning areas in medical imaging and clinical NLP. I obtained my PhD in Biomedical Engineering from Duke University with my thesis focused on developing devices and algorithms for cervical cancer screening. I obtained my BSc. in Biomedical Engineering and Business from the University of Rochester.
Research Areas
Authored Publications
Sort By
Google
Unprecedented Insights into Maternal Sleep: A Large-scale Longitudinal Analysis of Real-world Wearable Device Data Before, During, and After Pregnancy
Nichole Young-Lin
Conor Heneghan
Logan Schneider
Logan Niehaus
Ariel Haney
Karla Gleichauf
Jacqueline Shreibati
Belen Lafon
Lancet eBioMedicine (2025)
AfriMed-QA: A Pan-African Multi-Specialty Medical Question-Answering Benchmark Dataset
Tobi Olatunji
Abraham Toluwase Owodunni
Charles Nimo
Jennifer Orisakwe
Henok Biadglign Ademtew
Chris Fourie
Foutse Yuehgoh
Stephen Moore
Mardhiyah Sanni
Emmanuel Ayodele
Timothy Faniran
Bonaventure F. P. Dossou
Fola Omofoye
Wendy Kinara
Tassallah Abdullahi
Michael Best
2025
Development and Evaluation of ML Models for Cardiotocography Interpretation
Nicole Chiou
Nichole Young-Lin
Abdoulaye Diack
Christopher Kelly
Sanmi Koyejo
NPJ Women's Health (2025)
The Case for Globalizing Fairness: A Mixed Methods Study on the Perceptions of Colonialism, AI and Health in Africa
Iskandar Haykel
Chirag Nagpal
Aisha Walcott-Bryant
Sanmi Koyejo
2024
TRINDs: Assessing the Diagnostic Capabilities of Large Language Models for Tropical and Infectious Diseases
Nenad Tomašev
Chintan Ghate
Steve Adudans
Oluwatosin Akande
Sylvanus Aitkins
Geoffrey Siwo
Lynda Osadebe
Eric Ndombi
2024
TRINDs: Assessing the Diagnostic Capabilities of Large Language Models for Tropical and Infectious Diseases
Nenad Tomašev
Chintan Ghate
Oluwatosin Akande
Geoffrey Siwo
Steve Adudans
Sylvanus Aitkins
Lynda Osadebe
Eric Ndombi
Odianosen Ehiakhamen
2024
A Toolbox for Surfacing Health Equity Harms and Biases in Large Language Models
Heather Cole-Lewis
Nenad Tomašev
Liam McCoy
Leo Anthony Celi
Alanna Walton
Chirag Nagpal
Akeiylah DeWitt
Philip Mansfield
Sushant Prakash
Joelle Barral
Ivor Horn
Karan Singhal
Nature Medicine (2024)
Nteasee: A qualitative study of expert and general population perspectives on deploying AI for health in African countries
Iskandar Haykel
Kerrie Kauer
Florence Ofori
Tousif Ahmad
2024
The Case for Globalizing Fairness: A Mixed Methods Study on the Perceptions of Colonialism, AI and Health in Africa
Iskandar Haykel
Chirag Nagpal
Aisha Walcott-Bryant
Sanmi Koyejo
2024