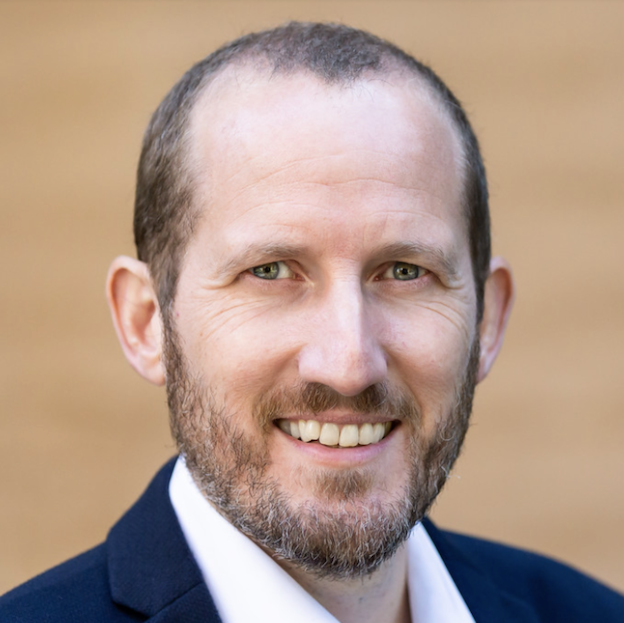
Vincent Vanhoucke
Vincent Vanhoucke is a Distinguished Engineer at Waymo. His research has spanned many areas of artificial intelligence and machine learning, from speech recognition to computer vision and robotics. At Google, he helped design and launch the first large scale production deep learning system which powered Google Voice Search, work that 10 years later earned the Best Paper Award from Signal Processing Magazine. In Google Brain, he worked on DistBelief, TensorFlow, GoogLeNet and the 'Inception' series of computer vision architectures, winning the 2014 ImageNet challenge. His Udacity lecture series has introduced over 100,000 students to deep learning. He founded the Robotics research team, now part of Google DeepMind, and won academic awards for notable works such as QT-Opt, SayCan, and RT-X. He is a founder and past president of the Robot Learning Foundation, which organizes the Conference on Robot Learning. He holds a doctorate from Stanford University, a diplôme d'ingénieur from the École Centrale Paris, and is a Fellow of the IEEE.
Research Areas
Authored Publications
Sort By
Google
Robotic Table Tennis: A Case Study into a High Speed Learning System
Jon Abelian
Saminda Abeyruwan
Michael Ahn
Justin Boyd
Erwin Johan Coumans
Omar Escareno
Wenbo Gao
Navdeep Jaitly
Juhana Kangaspunta
Satoshi Kataoka
Gus Kouretas
Yuheng Kuang
Corey Lynch
Thinh Nguyen
Ken Oslund
Barney J. Reed
Anish Shankar
Avi Singh
Grace Vesom
Peng Xu
Robotics: Science and Systems (2023)
Do As I Can, Not As I Say: Grounding Language in Robotic Affordances
Alexander Herzog
Alexander Toshkov Toshev
Andy Zeng
Anthony Brohan
Brian Andrew Ichter
Byron David
Chelsea Finn
Clayton Tan
Diego Reyes
Dmitry Kalashnikov
Eric Victor Jang
Jarek Liam Rettinghouse
Jornell Lacanlale Quiambao
Julian Ibarz
Karol Hausman
Kyle Alan Jeffrey
Linda Luu
Mengyuan Yan
Michael Soogil Ahn
Nicolas Sievers
Noah Brown
Omar Eduardo Escareno Cortes
Peng Xu
Peter Pastor Sampedro
Rosario Jauregui Ruano
Sally Augusta Jesmonth
Sergey Levine
Steve Xu
Yao Lu
Yevgen Chebotar
Yuheng Kuang
Conference on Robot Learning (CoRL) (2022)
Google Scanned Objects: A High-Quality Dataset of 3D Scanned Household Items
Anthony G. Francis
Brandon Kinman
Laura Downs
Nathan Koenig
Ryan M. Hickman
Thomas B. McHugh
(2022)
Socratic Models: Composing Zero-Shot Multimodal Reasoning with Language
Andy Zeng
Brian Ichter
Stefan Welker
Aveek Purohit
Michael Ryoo
Pete Florence
arXiv (2022)
Learning to Fold Real Garments with One Arm: A Case Study in Cloud-Based Robotics Research
Ryan Hoque
Kaushik Shivakumar
Shrey Aeron
Gabriel Deza
Aditya Ganapathi
Andy Zeng
Ken Goldberg
IEEE International Conference on Intelligent Robots and Systems (IROS) (2022) (to appear)
Mechanical Search on Shelves using LAX-RAY: Lateral Access X-RAY
Huang Huang
Marcus Dominguez-Kuhne
Vishal Satish
Michael Danielczuk
Kate Sanders
Jeff Ichnowski
Andrew Lee
Ken Goldberg
IEEE/RSJ International Conference on Intelligent Robots and Systems (IROS) (2021)
Differentiable Mapping Networks: Learning Structured Map Representations for Sparse Visual Localization
Peter Karkus
Rico Jonschkowski
International Conference on Robotics and Automation (ICRA) (2020)
X-Ray: Mechanical Search for an Occluded Object by Minimizing Support of Learned Occupancy Distributions
Michael Danielczuk
Ken Goldberg
International Conference on Intelligent Robots and Systems (IROS) (2020)
QT-Opt: Scalable Deep Reinforcement Learning for Vision-Based Robotic Manipulation
Dmitry Kalashnikov
Peter Pastor Sampedro
Julian Ibarz
Alexander Herzog
Eric Jang
Deirdre Quillen
Ethan Holly
Mrinal Kalakrishnan
Sergey Levine
CORL (2018)