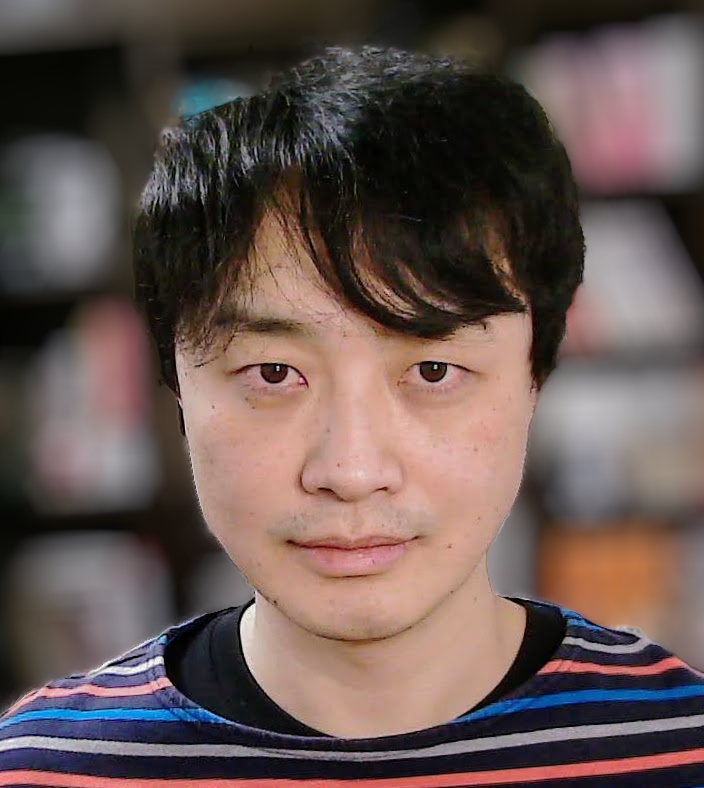
Heiga Zen
Heiga Zen received his AE from Suzuka National College of Technology, Suzuka, Japan, in 1999, and PhD from the Nagoya Institute of Technology, Nagoya, Japan, in 2006. He was an Intern/Co-Op researcher at the IBM T.J. Watson Research Center, Yorktown Heights, NY (2004--2005), and a Research Engineer at Toshiba Research Europe Ltd. Cambridge Research Laboratory, Cambridge, UK (2008--2011). At Google, he was with the Speech team from July 2011 to July 2018, then joined the Brain team from August 2018. From June 2023, he is a Principal Scientist at Google DeepMind, Japan. His research interests include speech technology and machine learning. He was one of the original authors and the first maintainer of the HMM-based speech synthesis system (HTS). He is a fellow of ISCA and IEEE.
Authored Publications
Sort By
Google
Translatotron 3: Speech to Speech Translation with Monolingual Data
Alon Levkovitch
Yifan Ding
Chulayuth Asawaroengchai
2024
Extracting Representative Subset from Massive Raw Texts for Training Pre-trained Neural Language Models
Jun Suzuki
Information Processing & Management Conference, 60 (2023) (to appear)
LibriTTS-R: Restoration of a Large-Scale Multi-Speaker TTS Corpus
Yifan Ding
Kohei Yatabe
Nobuyuki Morioka
Yu Zhang
Wei Han
Interspeech 2023 (2023)
Miipher: A Robust Speech Restoration Model Integrating Self-Supervised Speech Representation and Linguistic Features
Yifan Ding
Kohei Yatabe
Nobuyuki Morioka
Yu Zhang
Wei Han
WASPAA 2023 (2023) (to appear)
Twenty-Five Years of Evolution in Speech and Language Processing
Preview
Michael Picheny
Dilek Hakkani-Tur
IEEE Signal Processing Magazine, 40 (2023), pp. 27-39
Virtuoso: Massive Multilingual Speech-Text Joint Semi-Supervised Learning for Text-to-Speech
Takaaki Saeki
Zhehuai Chen
Nobuyuki Morioka
Yu Zhang
ICASSP (2023)
SpecGrad: Diffusion Probabilistic Model based Neural Vocoder with Adaptive Noise Spectral Shaping
Kohei Yatabe
Nanxin Chen
Proc. Interspeech (2022) (to appear)
MAESTRO: Matched Speech Text Representations through Modality Matching
Pedro Jose Moreno Mengibar
Yu Zhang
Zhehuai Chen
interspeech 2022 (2022) (to appear)
WaveFit: An Iterative and Non-autoregressive Neural Vocoder based on Fixed-Point Iteration
Kohei Yatabe
Proc. IEEE Spoken Language Technology Workshop (SLT) (2022) (to appear)