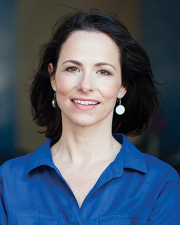
Fernanda Viegas
Authored Publications
Sort By
Preview abstract
AI is powerful and has the potential to deliver many benefits to the Nigerian economy. As such, the government needs to play an important role in partnering with industry and the community to ensure its deployment is safe, fair, and produces positive outcomes. Given the early stage of AI development in Nigeria, we believe it’s important to make sure that policy makers have a clear and consistent understanding of the current state of AI in Nigeria-- the state of current laws and regulations as it applies to AI, current applications of AI, and the challenges AI presents on a policy level. We also present areas where the government, in collaboration with wider civil society and AI practitioners, can play a crucial role in advancing AI in Nigeria. We hope this paper can help in evolving the discussion to address policy ideas and implementation of AI in Nigeria.
View details
Human-Centered Tools for Coping with Imperfect Algorithms during Medical Decision-Making
Jason Hipp
Daniel Smilkov
Martin Stumpe
Conference on Human Factors in Computing Systems (2019)
Preview abstract
Machine learning (ML) is increasingly being used in image retrieval systems for medical decision making. One application of ML is to retrieve visually similar medical images from past patients (e.g. tissue from biopsies) to reference when making a medical decision with a new patient. However, no algorithm can perfectly capture an expert's ideal notion of similarity for every case: an image that is algorithmically determined to be similar may not be medically relevant to a doctor's specific diagnostic needs. In this paper, we identified the needs of pathologists when searching for similar images retrieved using a deep learning algorithm, and developed tools that empower users to cope with the search algorithm on-the-fly, communicating what types of similarity are most important at different moments in time. In two evaluations with pathologists, we found that these refinement tools increased the diagnostic utility of images found and increased user trust in the algorithm. The tools were preferred over a traditional interface, without a loss in diagnostic accuracy. We also observed that users adopted new strategies when using refinement tools, re-purposing them to test and understand the underlying algorithm and to disambiguate ML errors from their own errors. Taken together, these findings inform future human-ML collaborative systems for expert decision-making.
View details
XRAI: Better Attributions Through Regions
International Conference on Computer Vision 2019 (ICCV) (2019) (to appear)
Preview abstract
Saliency methods can aid understanding of deep neural networks. Recent years have witnessed many improvements to saliency methods, as well as new ways for evaluating them. In this paper, we 1) present a novel region-based attribution method, XRAI, that builds upon integrated gradients (Sundararajan et al. 2017), 2) introduce evaluation methods for empirically assessing the quality of image-based saliency maps (Performance Information Curves (PICs)), and 3) contribute an axiom-based sanity check for attribution methods. Through empirical experiments and example results, we show that XRAI produces better results than other saliency methods for common models and the ImageNet dataset.
View details
TensorFlow.js: Machine Learning for the Web and Beyond
Daniel Smilkov
Nikhil Thorat
Yannick Assogba
Ann Yuan
Nick Kreeger
Ping Yu
Kangyi Zhang
Eric Nielsen
Stan Bileschi
Charles Nicholson
Sandeep N. Gupta
Sarah Sirajuddin
D. Sculley
Rajat Monga
SysML, Palo Alto, CA, USA (2019)
Preview abstract
TensorFlow.js is a library for building and executing machine learning algorithms in JavaScript. TensorFlow.js models run in a web browser and in the Node.js environment. The library is part of the TensorFlow ecosystem, providing a set of APIs that are compatible with those in Python, allowing models to be ported between the Python and JavaScript ecosystems. TensorFlow.js has empowered a new set of developers from the extensive JavaScript community to build and deploy machine learning models and enabled new classes of on-device computation. This paper describes the design, API, and implementation of TensorFlow.js, and highlights some of the impactful use cases.
View details
Preview abstract
A key challenge in developing and deploying Machine Learning (ML) systems is understanding their performance across a wide range of inputs. To address this challenge, we created the What-If Tool, an open-source application that allows practitioners to probe, visualize, and analyze ML systems, with minimal coding. The What-If Tool lets practitioners test performance in hypothetical situations, analyze the importance of different data features, and visualize model behavior across multiple models and subsets of input data. It also lets practitioners measure systems according to multiple ML fairness metrics. We describe the design of the tool, and report on real-life usage at different organizations.
View details
Interpretability Beyond Feature Attribution: Quantitative Testing with Concept Activation Vectors (TCAV)
Justin Gilmer
ICML (2018)
Preview abstract
The interpretation of deep learning models is a challenge due to their size, complexity, and often opaque internal state. In addition, many systems, such as image classifiers, operate on low-level features rather than high-level concepts. To address these challenges, we introduce Concept Activation Vectors (CAVs), which provide an interpretation of a neural net's internal state in terms of human-friendly concepts. The key idea is to view the high-dimensional internal state of a neural net as an aid, not an obstacle. We show how to use CAVs as part of a technique, Testing with CAVs (TCAV), that uses directional derivatives to quantify the degree to which a user-defined concept is important to a classification result--for example, how sensitive a prediction of “zebra” is to the presence of stripes. Using the domain of image classification as a testing ground, we describe how CAVs may be used to explore hypotheses and generate insights for a standard image classification network as well as a medical application.
View details
Visualizing Dataflow Graphs of Deep Learning Models in TensorFlow
Kanit Wongsuphasawat
Daniel Smilkov
Dandelion Mane
Doug Fritz
Dilip Krishnan
IEEE Transaction on Visualization and Computer Graphics (2017)
Preview abstract
We present a design study of the TensorFlow Graph Visualizer, part of the TensorFlow machine intelligence platform. This
tool helps users understand complex machine learning architectures by visualizing their underlying dataflow graphs. The tool works
by applying a series of graph transformations that enable standard layout techniques to produce a legible interactive diagram. To
declutter the graph, we decouple non-critical nodes from the layout. To provide an overview, we build a clustered graph using the
hierarchical structure annotated in the source code. To support exploration of nested structure on demand, we perform edge bundling
to enable stable and responsive cluster expansion. Finally, we detect and highlight repeated structures to emphasize a model’s
modular composition. To demonstrate the utility of the visualizer, we describe example usage scenarios and report user feedback.
Overall, users find the visualizer useful for understanding, debugging, and sharing the structures of their models.
View details
Google's Multilingual Neural Machine Translation System: Enabling Zero-Shot Translation
Mike Schuster
Maxim Krikun
Nikhil Thorat
Macduff Hughes
Google (2016)
Preview abstract
We propose a simple, elegant solution to use a single Neural Machine Translation (NMT) model to translate between multiple languages. Our solution requires no change in the model architecture from our base system but instead introduces an artificial token at the beginning of the input sentence to specify the required target language. The rest of the model, which includes encoder, decoder and attention, remains unchanged and is shared across all languages. Using a shared wordpiece vocabulary, our approach enables Multilingual NMT using a single model without any increase in parameters, which is significantly simpler than previous proposals for Multilingual NMT. Our method often improves the translation quality of all involved language pairs, even while keeping the total number of model parameters constant. On the WMT'14 benchmarks, a single multilingual model achieves comparable performance for English->French and surpasses state-of-the-art results for English->German. Similarly, a single multilingual model surpasses state-of-the-art results for French->English and German->English on WMT'14 and WMT'15 benchmarks respectively. On production corpora, multilingual models of up to twelve language pairs allow for better translation of many individual pairs. In addition to improving the translation quality of language pairs that the model was trained with, our models can also learn to perform implicit bridging between language pairs never seen explicitly during training, showing that transfer learning and zero-shot translation is possible for neural translation. Finally, we show analyses that hints at a universal interlingua representation in our models and show some interesting examples when mixing languages.
View details
TensorFlow: Large-Scale Machine Learning on Heterogeneous Distributed Systems
Ashish Agarwal
Eugene Brevdo
Craig Citro
Matthieu Devin
Ian Goodfellow
Andrew Harp
Geoffrey Irving
Yangqing Jia
Rafal Jozefowicz
Lukasz Kaiser
Manjunath Kudlur
Dan Mané
Rajat Monga
Chris Olah
Mike Schuster
Jonathon Shlens
Benoit Steiner
Ilya Sutskever
Kunal Talwar
Paul Tucker
Vijay Vasudevan
Pete Warden
Yuan Yu
Xiaoqiang Zheng
tensorflow.org (2015)
Preview abstract
TensorFlow is an interface for expressing machine learning
algorithms, and an implementation for executing such algorithms.
A computation expressed using TensorFlow can be
executed with little or no change on a wide variety of heterogeneous
systems, ranging from mobile devices such as phones
and tablets up to large-scale distributed systems of hundreds
of machines and thousands of computational devices such as
GPU cards. The system is flexible and can be used to express
a wide variety of algorithms, including training and inference
algorithms for deep neural network models, and it has been
used for conducting research and for deploying machine learning
systems into production across more than a dozen areas of
computer science and other fields, including speech recognition,
computer vision, robotics, information retrieval, natural
language processing, geographic information extraction, and
computational drug discovery. This paper describes the TensorFlow
interface and an implementation of that interface that
we have built at Google. The TensorFlow API and a reference
implementation were released as an open-source package under
the Apache 2.0 license in November, 2015 and are available at
www.tensorflow.org.
View details
Google+ Ripples: A Native Visualization of Information Flow
Preview
Jack Hebert
Geoffrey Borggaard
Alison Cichowlas
Jonathan Feinberg
Christopher Wren
Proceedings of the 22nd International World Wide Web Conference (2013), pp. 1389-1398