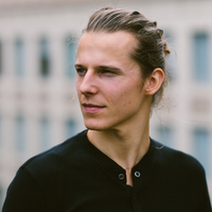
Ellery Wulczyn
Research Areas
Authored Publications
Sort By
Health equity assessment of machine learning performance (HEAL): a framework and dermatology AI model case study
Terry Spitz
Malcolm Chelliah
Heather Cole-Lewis
Stephanie Farquhar
Qinghan Xue
Jenna Lester
Cían Hughes
Patricia Strachan
Fraser Tan
Peggy Bui
Craig Mermel
Lily Peng
Sunny Virmani
Ivor Horn
Cameron Chen
The Lancet eClinicalMedicine (2024)
Preview abstract
Background
Artificial intelligence (AI) has repeatedly been shown to encode historical inequities in healthcare. We aimed to develop a framework to quantitatively assess the performance equity of health AI technologies and to illustrate its utility via a case study.
Methods
Here, we propose a methodology to assess whether health AI technologies prioritise performance for patient populations experiencing worse outcomes, that is complementary to existing fairness metrics. We developed the Health Equity Assessment of machine Learning performance (HEAL) framework designed to quantitatively assess the performance equity of health AI technologies via a four-step interdisciplinary process to understand and quantify domain-specific criteria, and the resulting HEAL metric. As an illustrative case study (analysis conducted between October 2022 and January 2023), we applied the HEAL framework to a dermatology AI model. A set of 5420 teledermatology cases (store-and-forward cases from patients of 20 years or older, submitted from primary care providers in the USA and skin cancer clinics in Australia), enriched for diversity in age, sex and race/ethnicity, was used to retrospectively evaluate the AI model's HEAL metric, defined as the likelihood that the AI model performs better for subpopulations with worse average health outcomes as compared to others. The likelihood that AI performance was anticorrelated to pre-existing health outcomes was estimated using bootstrap methods as the probability that the negated Spearman's rank correlation coefficient (i.e., “R”) was greater than zero. Positive values of R suggest that subpopulations with poorer health outcomes have better AI model performance. Thus, the HEAL metric, defined as p (R >0), measures how likely the AI technology is to prioritise performance for subpopulations with worse average health outcomes as compared to others (presented as a percentage below). Health outcomes were quantified as disability-adjusted life years (DALYs) when grouping by sex and age, and years of life lost (YLLs) when grouping by race/ethnicity. AI performance was measured as top-3 agreement with the reference diagnosis from a panel of 3 dermatologists per case.
Findings
Across all dermatologic conditions, the HEAL metric was 80.5% for prioritizing AI performance of racial/ethnic subpopulations based on YLLs, and 92.1% and 0.0% respectively for prioritizing AI performance of sex and age subpopulations based on DALYs. Certain dermatologic conditions were significantly associated with greater AI model performance compared to a reference category of less common conditions. For skin cancer conditions, the HEAL metric was 73.8% for prioritizing AI performance of age subpopulations based on DALYs.
Interpretation
Analysis using the proposed HEAL framework showed that the dermatology AI model prioritised performance for race/ethnicity, sex (all conditions) and age (cancer conditions) subpopulations with respect to pre-existing health disparities. More work is needed to investigate ways of promoting equitable AI performance across age for non-cancer conditions and to better understand how AI models can contribute towards improving equity in health outcomes.
View details
Towards Physician-Level Medical Question Answering with Large Language Models
Karan Singhal
Juro Gottweis
Le Hou
Kevin Clark
Heather Cole-Lewis
Amy Wang
Sami Lachgar
Philip Mansfield
Sushant Prakash
Bradley Green
Ewa Dominowska
Nenad Tomašev
Renee Wong
Sara Mahdavi
Joelle Barral
Arxiv (2023) (to appear)
Preview abstract
Recent artificial intelligence (AI) systems have reached milestones in "grand challenges" ranging from Go to protein-folding. The capability to retrieve medical knowledge, reason over it, and answer medical questions comparably to physicians has long been viewed as one such grand challenge.
Large language models (LLMs) have catalyzed significant progress in medical question answering; Med-PaLM was the first model to exceed a "passing" score in US Medical Licensing Examination (USMLE) style questions with a score of 67.2% on the MedQA dataset. However, this and other prior work suggested significant room for improvement, especially when models' answers were compared to clinicians' answers. Here we present Med-PaLM 2, which bridges these gaps by leveraging a combination of base LLM improvements (PaLM 2), medical domain finetuning, and prompting strategies including a novel ensemble refinement approach.
Med-PaLM 2 scored up to 86.5% on the MedQA dataset, improving upon Med-PaLM by over 19% and setting a new state-of-the-art. We also observed performance approaching or exceeding state-of-the-art across MedMCQA, PubMedQA, and MMLU clinical topics datasets.
We performed detailed human evaluations on long-form questions along multiple axes relevant to clinical applications. In pairwise comparative ranking of 1066 consumer medical questions, physicians preferred Med-PaLM 2 answers to those produced by physicians on eight of nine axes pertaining to clinical utility (p < 0.001). We also observed significant improvements compared to Med-PaLM on every evaluation axis (p < 0.001) on newly introduced datasets of 240 long-form "adversarial" questions to probe LLM limitations.
While further studies are necessary to validate the efficacy of these models in real-world settings, these results highlight rapid progress towards physician-level performance in medical question answering.
View details
Domain-specific optimization and diverse evaluation of self-supervised models for histopathology
Jeremy Lai
Faruk Ahmed
Supriya Vijay
Jessica Loo
Saurabh Vyawahare
Saloni Agarwal
Fayaz Jamil
Cameron Chen
arXiv (2023)
Preview abstract
Task-specific deep learning models in histopathology offer promising opportunities for improving diagnosis, clinical research, and precision medicine. However, development of such models is often limited by availability of high-quality data. Foundation models in histopathology that learn general representations across a wide range of tissue types, diagnoses, and magnifications offer the potential to reduce the data, compute, and technical expertise necessary to develop task-specific deep learning models with the required level of model performance. In this work, we describe the development and evaluation of foundation models for histopathology via self-supervised learning (SSL). We first establish a diverse set of benchmark tasks involving 17 unique tissue types and 12 unique cancer types and spanning different optimal magnifications and task types. Next, we use this benchmark to explore and evaluate histopathology-specific SSL methods followed by further evaluation on held out patch-level and weakly supervised tasks. We found that standard SSL methods thoughtfully applied to histopathology images are performant across our benchmark tasks and that domain-specific methodological improvements can further increase performance. Our findings reinforce the value of using domain-specific SSL methods in pathology, and establish a set of high quality foundation models to enable further research across diverse applications.
View details
Predicting lymph node metastasis from primary tumor histology and clinicopathologic factors in colorectal cancer using deep learning
Fraser Tan
Isabelle Flament-Auvigne
Trissia Brown
Markus Plass
Robert Reihs
Heimo Mueller
Kurt Zatloukal
Pema Richeson
Lily Peng
Craig Mermel
Cameron Chen
Saurabh Gombar
Thomas Montine
Jeanne Shen
Nature Communications Medicine, 3 (2023), pp. 59
Preview abstract
Background: Presence of lymph node metastasis (LNM) influences prognosis and clinical decision-making in colorectal cancer. However, detection of LNM is variable and depends on a number of external factors. Deep learning has shown success in computational pathology, but has struggled to boost performance when combined with known predictors.
Methods: Machine-learned features are created by clustering deep learning embeddings of small patches of tumor in colorectal cancer via k-means, and then selecting the top clusters that add predictive value to a logistic regression model when combined with known baseline clinicopathological variables. We then analyze performance of logistic regression models trained with and without these machine-learned features in combination with the baseline variables.
Results: The machine-learned extracted features provide independent signal for the presence of LNM (AUROC: 0.638, 95% CI: [0.590, 0.683]). Furthermore, the machine-learned features add predictive value to the set of 6 clinicopathologic variables in an external validation set (likelihood ratio test, p < 0.00032; AUROC: 0.740, 95% CI: [0.701, 0.780]). A model incorporating these features can also further risk-stratify patients with and without identified metastasis (p < 0.001 for both stage II and stage III).
Conclusion: This work demonstrates an effective approach to combine deep learning with established clinicopathologic factors in order to identify independently informative features associated with LNM. Further work building on these specific results may have important impact in prognostication and therapeutic decision making for LNM. Additionally, this general computational approach may prove useful in other contexts.
View details
Pathologist Validation of a Machine Learning–Derived Feature for Colon Cancer Risk Stratification
Vincenzo L’Imperio
Markus Plass
Heimo Müller
Nicolò Tamini
Luca Gianotti
Nicola Zucchini
Robert Reihs
Lily Peng
Cameron Chen
Marialuisa Lavitrano
David F. Steiner
Kurt Zatloukal
Fabio Pagni
JAMA Network Open (2023)
Preview abstract
Importance: Identifying new prognostic features in colon cancer has the potential to refine histopathologic review and inform patient care. Although prognostic artificial intelligence systems have recently demonstrated significant risk stratification for several cancer types, studies have not yet shown that the machine learning–derived features associated with these prognostic artificial intelligence systems are both interpretable and usable by pathologists.
Objective: To evaluate whether pathologist scoring of a histopathologic feature previously identified by machine learning is associated with survival among patients with colon cancer.
Design, Setting, and Participants: This prognostic study used deidentified, archived colorectal cancer cases from January 2013 to December 2015 from the University of Milano-Bicocca. All available histologic slides from 258 consecutive colon adenocarcinoma cases were reviewed from December 2021 to February 2022 by 2 pathologists, who conducted semiquantitative scoring for tumor adipose feature (TAF), which was previously identified via a prognostic deep learning model developed with an independent colorectal cancer cohort.
Main Outcomes and Measures: Prognostic value of TAF for overall survival and disease-specific survival as measured by univariable and multivariable regression analyses. Interpathologist agreement in TAF scoring was also evaluated.
Results: A total of 258 colon adenocarcinoma histopathologic cases from 258 patients (138 men [53%]; median age, 67 years [IQR, 65-81 years]) with stage II (n = 119) or stage III (n = 139) cancer were included. Tumor adipose feature was identified in 120 cases (widespread in 63 cases, multifocal in 31, and unifocal in 26). For overall survival analysis after adjustment for tumor stage, TAF was independently prognostic in 2 ways: TAF as a binary feature (presence vs absence: hazard ratio [HR] for presence of TAF, 1.55 [95% CI, 1.07-2.25]; P = .02) and TAF as a semiquantitative categorical feature (HR for widespread TAF, 1.87 [95% CI, 1.23-2.85]; P = .004). Interpathologist agreement for widespread TAF vs lower categories (absent, unifocal, or multifocal) was 90%, corresponding to a κ metric at this threshold of 0.69 (95% CI, 0.58-0.80).
Conclusions and Relevance: In this prognostic study, pathologists were able to learn and reproducibly score for TAF, providing significant risk stratification on this independent data set. Although additional work is warranted to understand the biological significance of this feature and to establish broadly reproducible TAF scoring, this work represents the first validation to date of human expert learning from machine learning in pathology. Specifically, this validation demonstrates that a computationally identified histologic feature can represent a human-identifiable, prognostic feature with the potential for integration into pathology practice.
View details
Robust and data-efficient generalization of self-supervised machine learning for diagnostic imaging
Laura Anne Culp
Jan Freyberg
Basil Mustafa
Sebastien Baur
Simon Kornblith
Ting Chen
Patricia MacWilliams
Sara Mahdavi
Megan Zoë Walker
Aaron Loh
Cameron Chen
Scott Mayer McKinney
Jim Winkens
Zach William Beaver
Fiona Keleher Ryan
Mozziyar Etemadi
Umesh Telang
Lily Hao Yi Peng
Geoffrey Everest Hinton
Neil Houlsby
Mohammad Norouzi
Nature Biomedical Engineering (2023)
Preview abstract
Machine-learning models for medical tasks can match or surpass the performance of clinical experts. However, in settings differing from those of the training dataset, the performance of a model can deteriorate substantially. Here we report a representation-learning strategy for machine-learning models applied to medical-imaging tasks that mitigates such ‘out of distribution’ performance problem and that improves model robustness and training efficiency. The strategy, which we named REMEDIS (for ‘Robust and Efficient Medical Imaging with Self-supervision’), combines large-scale supervised transfer learning on natural images and intermediate contrastive self-supervised learning on medical images and requires minimal task-specific customization. We show the utility of REMEDIS in a range of diagnostic-imaging tasks covering six imaging domains and 15 test datasets, and by simulating three realistic out-of-distribution scenarios. REMEDIS improved in-distribution diagnostic accuracies up to 11.5% with respect to strong supervised baseline models, and in out-of-distribution settings required only 1–33% of the data for retraining to match the performance of supervised models retrained using all available data. REMEDIS may accelerate the development lifecycle of machine-learning models for medical imaging.
View details
Deep learning models for histologic grading of breast cancer and association with disease prognosis
Trissia Brown
Isabelle Flament
Fraser Tan
Yuannan Cai
Kunal Nagpal
Emad Rakha
David J. Dabbs
Niels Olson
James H. Wren
Elaine E. Thompson
Erik Seetao
Carrie Robinson
Melissa Miao
Fabien Beckers
Lily Hao Yi Peng
Craig Mermel
Cameron Chen
npj Breast Cancer (2022)
Preview abstract
Histologic grading of breast cancer involves review and scoring of three well-established morphologic features: mitotic count, nuclear pleomorphism, and tubule formation. Taken together, these features form the basis of the Nottingham Grading System which is used to inform breast cancer characterization and prognosis. In this study, we developed deep learning models to perform histologic scoring of all three components using digitized hematoxylin and eosin-stained slides containing invasive breast carcinoma. We then evaluated the prognostic potential of these models using an external test set and progression free interval as the primary outcome. The individual component models performed at or above published benchmarks for algorithm-based grading approaches and achieved high concordance rates in comparison to pathologist grading. Prognostic performance of histologic scoring provided by the deep learning-based grading was on par with that of pathologists performing review of matched slides. Additionally, by providing scores for each component feature, the deep-learning based approach provided the potential to identify the grading components contributing most to prognostic value. This may enable optimized prognostic models as well as opportunities to improve access to consistent grading and better understand the links between histologic features and clinical outcomes in breast cancer.
View details
Predicting prostate cancer specific-mortality with artificial intelligence-based Gleason grading
Kunal Nagpal
Matthew Symonds
Melissa Moran
Markus Plass
Robert Reihs
Farah Nader
Fraser Tan
Yuannan Cai
Trissia Brown
Isabelle Flament
Mahul Amin
Martin Stumpe
Heimo Muller
Peter Regitnig
Andreas Holzinger
Lily Hao Yi Peng
Cameron Chen
Kurt Zatloukal
Craig Mermel
Communications Medicine (2021)
Preview abstract
Background. Gleason grading of prostate cancer is an important prognostic factor, but suffers from poor reproducibility, particularly among non-subspecialist pathologists. Although artificial intelligence (A.I.) tools have demonstrated Gleason grading on-par with expert pathologists, it remains an open question whether and to what extent A.I. grading translates to better prognostication.
Methods. In this study, we developed a system to predict prostate cancer-specific mortality via A.I.-based Gleason grading and subsequently evaluated its ability to risk-stratify patients on an independent retrospective cohort of 2807 prostatectomy cases from a single European center with 5–25 years of follow-up (median: 13, interquartile range 9–17).
Results. Here, we show that the A.I.’s risk scores produced a C-index of 0.84 (95% CI 0.80–0.87) for prostate cancer-specific mortality. Upon discretizing these risk scores into risk groups analogous to pathologist Grade Groups (GG), the A.I. has a C-index of 0.82 (95% CI 0.78–0.85). On the subset of cases with a GG provided in the original pathology report (n = 1517), the A.I.’s C-indices are 0.87 and 0.85 for continuous and discrete grading, respectively, compared to 0.79 (95% CI 0.71–0.86) for GG obtained from the reports. These represent improvements of 0.08 (95% CI 0.01–0.15) and 0.07 (95% CI 0.00–0.14), respectively.
Conclusions. Our results suggest that A.I.-based Gleason grading can lead to effective risk stratification, and warrants further evaluation for improving disease management.
View details
Validation and Clinical Applicability of Whole-Volume Automated Segmentation of Optical Coherence Tomography in Retinal Disease Using Deep Learning
Marc Wilson
Reena Chopra
Megan Zoë Wilson
Charlotte Cooper
Patricia MacWilliams
Daniela Florea
Cían Hughes
Hagar Khalid
Sandra Vermeirsch
Luke Nicholson
Pearse Keane
Konstantinos Balaskas
Christopher Kelly
JAMA Ophthalmology (2021)
Preview abstract
Importance Quantitative volumetric measures of retinal disease in optical coherence tomography (OCT) scans are infeasible to perform owing to the time required for manual grading. Expert-level deep learning systems for automatic OCT segmentation have recently been developed. However, the potential clinical applicability of these systems is largely unknown.
Objective To evaluate a deep learning model for whole-volume segmentation of 4 clinically important pathological features and assess clinical applicability.
Design, Setting, Participants This diagnostic study used OCT data from 173 patients with a total of 15 558 B-scans, treated at Moorfields Eye Hospital. The data set included 2 common OCT devices and 2 macular conditions: wet age-related macular degeneration (107 scans) and diabetic macular edema (66 scans), covering the full range of severity, and from 3 points during treatment. Two expert graders performed pixel-level segmentations of intraretinal fluid, subretinal fluid, subretinal hyperreflective material, and pigment epithelial detachment, including all B-scans in each OCT volume, taking as long as 50 hours per scan. Quantitative evaluation of whole-volume model segmentations was performed. Qualitative evaluation of clinical applicability by 3 retinal experts was also conducted. Data were collected from June 1, 2012, to January 31, 2017, for set 1 and from January 1 to December 31, 2017, for set 2; graded between November 2018 and January 2020; and analyzed from February 2020 to November 2020.
Main Outcomes and Measures Rating and stack ranking for clinical applicability by retinal specialists, model-grader agreement for voxelwise segmentations, and total volume evaluated using Dice similarity coefficients, Bland-Altman plots, and intraclass correlation coefficients.
Results Among the 173 patients included in the analysis (92 [53%] women), qualitative assessment found that automated whole-volume segmentation ranked better than or comparable to at least 1 expert grader in 127 scans (73%; 95% CI, 66%-79%). A neutral or positive rating was given to 135 model segmentations (78%; 95% CI, 71%-84%) and 309 expert gradings (2 per scan) (89%; 95% CI, 86%-92%). The model was rated neutrally or positively in 86% to 92% of diabetic macular edema scans and 53% to 87% of age-related macular degeneration scans. Intraclass correlations ranged from 0.33 (95% CI, 0.08-0.96) to 0.96 (95% CI, 0.90-0.99). Dice similarity coefficients ranged from 0.43 (95% CI, 0.29-0.66) to 0.78 (95% CI, 0.57-0.85).
Conclusions and Relevance This deep learning–based segmentation tool provided clinically useful measures of retinal disease that would otherwise be infeasible to obtain. Qualitative evaluation was additionally important to reveal clinical applicability for both care management and research.
View details
Interpretable Survival Prediction for Colorectal Cancer using Deep Learning
Melissa Moran
Markus Plass
Robert Reihs
Fraser Tan
Isabelle Flament
Trissia Brown
Peter Regitnig
Cameron Chen
Apaar Sadhwani
Bob MacDonald
Benny Ayalew
Lily Hao Yi Peng
Heimo Mueller
Zhaoyang Xu
Martin Stumpe
Kurt Zatloukal
Craig Mermel
npj Digital Medicine (2021)
Preview abstract
Deriving interpretable prognostic features from deep-learning-based prognostic histopathology models remains a challenge. In this study, we developed a deep learning system (DLS) for predicting disease-specific survival for stage II and III colorectal cancer using 3652 cases (27,300 slides). When evaluated on two validation datasets containing 1239 cases (9340 slides) and 738 cases (7140 slides), respectively, the DLS achieved a 5-year disease-specific survival AUC of 0.70 (95% CI: 0.66–0.73) and 0.69 (95% CI: 0.64–0.72), and added significant predictive value to a set of nine clinicopathologic features. To interpret the DLS, we explored the ability of different human-interpretable features to explain the variance in DLS scores. We observed that clinicopathologic features such as T-category, N-category, and grade explained a small fraction of the variance in DLS scores (R2 = 18% in both validation sets). Next, we generated human-interpretable histologic features by clustering embeddings from a deep-learning-based image-similarity model and showed that they explained the majority of the variance (R2 of 73–80%). Furthermore, the clustering-derived feature most strongly associated with high DLS scores was also highly prognostic in isolation. With a distinct visual appearance (poorly differentiated tumor cell clusters adjacent to adipose tissue), this feature was identified by annotators with 87.0–95.5% accuracy. Our approach can be used to explain predictions from a prognostic deep learning model and uncover potentially-novel prognostic features that can be reliably identified by people for future validation studies.
View details