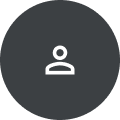
Awa Dieng
I am a Research Associate on the Google Brain team working on Machine Learning, Causality, and Algorithmic Fairness.
Personal website: awadieng.me
Research Areas
Authored Publications
Sort By
Google
The Case for Globalizing Fairness: A Mixed Methods Study on the Perceptions of Colonialism, AI and Health in Africa
Iskandar Haykel
Chirag Nagpal
Aisha Walcott-Bryant
Sanmi Koyejo
2024
TRINDs: Assessing the Diagnostic Capabilities of Large Language Models for Tropical and Infectious Diseases
Nenad Tomašev
Chintan Ghate
Steve Adudans
Oluwatosin Akande
Sylvanus Aitkins
Geoffrey Siwo
Lynda Osadebe
Eric Ndombi
2024
TRINDs: Assessing the Diagnostic Capabilities of Large Language Models for Tropical and Infectious Diseases
Nenad Tomašev
Chintan Ghate
Oluwatosin Akande
Geoffrey Siwo
Steve Adudans
Sylvanus Aitkins
Lynda Osadebe
Eric Ndombi
Odianosen Ehiakhamen
2024
A Toolbox for Surfacing Health Equity Harms and Biases in Large Language Models
Heather Cole-Lewis
Nenad Tomašev
Liam McCoy
Leo Anthony Celi
Alanna Walton
Chirag Nagpal
Akeiylah DeWitt
Philip Mansfield
Sushant Prakash
Joelle Barral
Ivor Horn
Karan Singhal
Nature Medicine (2024)
Nteasee: A qualitative study of expert and general population perspectives on deploying AI for health in African countries
Iskandar Haykel
Kerrie Kauer
Florence Ofori
Tousif Ahmad
2024
The Case for Globalizing Fairness: A Mixed Methods Study on the Perceptions of Colonialism, AI and Health in Africa
Iskandar Haykel
Chirag Nagpal
Aisha Walcott-Bryant
Sanmi Koyejo
2024
Diagnosing failures of fairness transfer across distribution shift in real-world medical settings
Jessica Schrouff
Sanmi Koyejo
Eva Schnider
Krista Opsahl-Ong
Alex Brown
Diana Mincu
Christina Chen
Silvia Chiappa
Proceedings of Neural Information Processing Systems 2022 (2022)
Causal inference methods for combining randomized trials and observational studies: a review
Benedicte Colnet
Imke Mayer
Guanhua Chen
Ruohong Li
Gaël Varoquaux
Jean-Philippe Vert
Julie Josse
Shu Yang
arXiv (2020)