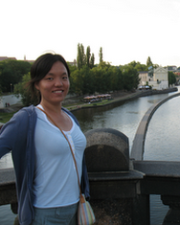
Pi-Chuan Chang
Pi-Chuan is the technical lead for the open source project DeepVariant at Google Health. She began working on DeepVariant before its first open source release in December 2017, and has led multiple releases over the years. At Google, she has led machine learning projects with public launches in various product areas, such YouTube and Search. Pi-Chuan holds a CS PhD from Stanford, specializing in natural language processing and machine translation. Pi-Chuan also has a BS and MS from National Taiwan University, where she worked on better language modeling for Chinese speech recognition systems.
Authored Publications
Sort By
Towards Generalist Biomedical AI
Danny Driess
Andrew Carroll
Chuck Lau
Ryutaro Tanno
Ira Ktena
Anil Palepu
Basil Mustafa
Aakanksha Chowdhery
Simon Kornblith
Philip Mansfield
Sushant Prakash
Renee Wong
Sunny Virmani
Sara Mahdavi
Bradley Green
Ewa Dominowska
Joelle Barral
Karan Singhal
Pete Florence
NEJM AI (2024)
Preview abstract
BACKGROUND: Medicine is inherently multimodal, requiring the simultaneous interpretation and integration of insights between many data modalities spanning text, imaging, genomics, and more. Generalist biomedical artificial intelligence systems that flexibly encode, integrate, and interpret these data might better enable impactful applications ranging from scientific discovery to care delivery.
METHODS: To catalyze development of these models, we curated MultiMedBench, a new multimodal biomedical benchmark. MultiMedBench encompasses 14 diverse tasks, such as medical question answering, mammography and dermatology image interpretation, radiology report generation and summarization, and genomic variant calling. We then introduced Med-PaLM Multimodal (Med-PaLM M), our proof of concept for a generalist biomedical AI system that flexibly encodes and interprets biomedical data including clinical language, imaging, and genomics with the same set of model weights. To further probe the capabilities and limitations of Med-PaLM M, we conducted a radiologist evaluation of model-generated (and human) chest x-ray reports.
RESULTS: We observed encouraging performance across model scales. Med-PaLM M reached performance competitive with or exceeding the state of the art on all MultiMedBench tasks, often surpassing specialist models by a wide margin. In a side-by-side ranking on 246 retrospective chest x-rays, clinicians expressed a pairwise preference for Med-PaLM Multimodal reports over those produced by radiologists in up to 40.50% of cases, suggesting potential clinical utility.
CONCLUSIONS: Although considerable work is needed to validate these models in real-world cases and understand if cross-modality generalization is possible, our results represent a milestone toward the development of generalist biomedical artificial intelligence systems.
View details
Accurate human genome analysis with Element Avidity sequencing
Andrew Carroll
Bryan Lajoie
Daniel Cook
Kelly N. Blease
Kishwar Shafin
Lucas Brambrink
Maria Nattestad
Semyon Kruglyak
bioRxiv (2023)
Preview abstract
We investigate the new sequencing technology Avidity from Element Biosciences. We show that Avidity whole genome sequencing matches mapping and variant calling accuracy with Illumina at high coverages (30x-50x) and is noticeably more accurate at lower coverages (20x-30x). We quantify base error rates of Element reads, finding lower error rates, especially in homopolymer and tandem repeat regions. We use Element’s ability to generate paired end sequencing with longer insert sizes than typical short–read sequencing. We show that longer insert sizes result in even higher accuracy, with long insert Element sequencing giving noticeably more accurate genome analyses at all coverages.
View details
Local read haplotagging enables accurate long-read small variant calling
Daniel Cook
Maria Nattestad
John E. Gorzynski
Sneha D. Goenka
Euan Ashley
Miten Jain
Karen Miga
Benedict Paten
Andrew Carroll
Kishwar Shafin
bioRxiv (2023)
Preview abstract
Long-read sequencing technology has enabled variant detection in difficult-to-map regions of the genome and enabled rapid genetic diagnosis in clinical settings. Rapidly evolving third-
generation sequencing like Pacific Biosciences (PacBio) and Oxford nanopore technologies (ONT) are introducing newer platforms and data types. It has been demonstrated that variant calling methods based on deep neural networks can use local haplotyping information with long-reads to improve the genotyping accuracy. However, using local haplotype information creates an overhead as variant calling needs to be performed multiple times which ultimately makes it difficult to extend to new data types and platforms as they get introduced. In this work, we have developed a local haplotype approximate method that enables state-of-the-art variant calling performance with multiple sequencing platforms including PacBio revio platfrom, ONT R10.4 simplex and duplex data. This addition of local haplotype approximation makes DeepVariant a universal variant calling solution for all long-read sequencing platforms.
View details
A draft human pangenome reference
Wen-Wei Liao
Mobin Asri
Jana Ebler
Daniel Doerr
Marina Haukness
Shuangjia Lu
Julian K. Lucas
Jean Monlong
Haley J. Abel
Silvia Buonaiuto
Xian Chang
Haoyu Cheng
Justin Chu
Vincenza Colonna
Jordan M. Eizenga
Xiaowen Feng
Christian Fischer
Robert S. Fulton
Shilpa Garg
Cristian Groza
Andrea Guarracino
William T. Harvey
Simon Heumos
Kerstin Howe
Miten Jain
Tsung-Yu Lu
Charles Markello
Fergal J. Martin
Matthew W. Mitchell
Katherine M. Munson
Moses Njagi Mwaniki
Adam M. Novak
Hugh E. Olsen
Trevor Pesout
David Porubsky
Pjotr Prins
Jonas A. Sibbesen
Jouni Sirén
Chad Tomlinson
Flavia Villani
Mitchell R. Vollger
Lucinda L Antonacci-Fulton
Gunjan Baid
Carl A. Baker
Anastasiya Belyaeva
Konstantinos Billis
Andrew Carroll
Sarah Cody
Daniel Cook
Robert M. Cook-Deegan
Omar E. Cornejo
Mark Diekhans
Peter Ebert
Susan Fairley
Olivier Fedrigo
Adam L. Felsenfeld
Giulio Formenti
Adam Frankish
Yan Gao
Nanibaa’ A. Garrison
Carlos Garcia Giron
Richard E. Green
Leanne Haggerty
Kendra Hoekzema
Thibaut Hourlier
Hanlee P. Ji
Eimear E. Kenny
Barbara A. Koenig
Jan O. Korbel
Jennifer Kordosky
Sergey Koren
HoJoon Lee
Alexandra P. Lewis
Hugo Magalhães
Santiago Marco-Sola
Pierre Marijon
Ann McCartney
Jennifer McDaniel
Jacquelyn Mountcastle
Maria Nattestad
Sergey Nurk
Nathan D. Olson
Alice B. Popejoy
Daniela Puiu
Mikko Rautiainen
Allison A. Regier
Arang Rhie
Samuel Sacco
Ashley D. Sanders
Valerie A. Schneider
Baergen I. Schultz
Kishwar Shafin
Michael W. Smith
Heidi J. Sofia
Ahmad N. Abou Tayoun
Francoise Thibauld-Nissen
Francesa Floriana Tricomi
Justin Wagner
Brian Walenz
Jonathan M. D. Wood
Aleksey V. Zimin
Guillaume Borque
Mark J. P. Chaisson
Paul Flicek
Adam M. Phillippy
Justin Zook
Evan E. Eichler
David Haussler
Ting Wang
Erich D. Jarvis
Karen H. Miga
Glenn Hickey
Erik Garrison
Tobias Marschall
Ira M. Hall
Heng Li
Benedict Paten
Nature (2023)
Preview abstract
Here the Human Pangenome Reference Consortium presents a first draft of the human pangenome reference. The pangenome contains 47 phased, diploid assemblies from a cohort of genetically diverse individuals. These assemblies cover more than 99% of the expected sequence in each genome and are more than 99% accurate at the structural and base pair levels. Based on alignments of the assemblies, we generate a draft pangenome that captures known variants and haplotypes and reveals new alleles at structurally complex loci. We also add 119 million base pairs of euchromatic polymorphic sequences and 1,115 gene duplications relative to the existing reference GRCh38. Roughly 90 million of the additional base pairs are derived from structural variation. Using our draft pangenome to analyse short-read data reduced small variant discovery errors by 34% and increased the number of structural variants detected per haplotype by 104% compared with GRCh38-based workflows, which enabled the typing of the vast majority of structural variant alleles per sample.
View details
Improving variant calling using population data and deep learning
Nae-Chyun Chen
Sidharth Goel
Andrew Carroll
BMC Bioinformatics (2023)
Preview abstract
Large-scale population variant data is often used to filter and aid interpretation of variant calls in a single sample. These approaches do not incorporate population information directly into the process of variant calling, and are often limited to filtering which trades recall for precision. In this study, we develop population-aware DeepVariant models with a new channel encoding allele frequencies from the 1000 Genomes Project. This model reduces variant calling errors, improving both precision and recall in single samples, and reduces rare homozygous and pathogenic clinvar calls cohort-wide. We assess the use of population-specific or diverse reference panels, finding the greatest accuracy with diverse panels, suggesting that large, diverse panels are preferable to individual populations, even when the population matches sample ancestry. Finally, we show that this benefit generalizes to samples with different ancestry from the training data even when the ancestry is also excluded from the reference panel.
View details
A deep-learning-based RNA-seq germline variant caller
Aarti Venkat
Andrew Carroll
Daniel Cook
Dennis Yelizarov
Francisco De La Vega
Yannick Pouliot
Bioinformatics Advances (2023)
Preview abstract
RNA-seq is a widely used technology for quantifying and studying gene expression. Many other applications have been developed for RNA-seq as well such as identifying quantitative trait loci, or identifying gene fusion events. However, germline variant calling has not been widely used because RNA-seq data tend to have high error rates and require special processing by variant callers. Here, we introduce a DeepVariant RNA-seq model capable of producing highly accurate variant calls from RNA-sequencing data. Our model outperforms existing approaches such as Platypus and GATK. We examine factors that influence accuracy, how our model addresses RNA editing events, and how additional thresholding can be used to allow for our models' use in a production pipeline.
View details
Improving variant calling using population data and deep learning
Andrew Carroll
Nae-Chyun Chen
Sidharth Goel
BMC Bioinformatics (2023)
Preview abstract
Large-scale population variant data is often used to filter and aid interpretation of variant calls in a single sample. These approaches do not incorporate population information directly into the process of variant calling, and are often limited to filtering which trades recall for precision. In this study, we develop population-aware DeepVariant models with a new channel encoding allele frequencies from the 1000 Genomes Project. This model reduces variant calling errors, improving both precision and recall in single samples, and reduces rare homozygous and pathogenic clinvar calls cohort-wide. We assess the use of population-specific or diverse reference panels, finding the greatest accuracy with diverse panels, suggesting that large, diverse panels are preferable to individual populations, even when the population matches sample ancestry. Finally, we show that this benefit generalizes to samples with different ancestry from the training data even when the ancestry is also excluded from the reference panel.
View details
Knowledge distillation for fast and accurate DNA sequence correction
Anastasiya Belyaeva
Joel Shor
Daniel Cook
Kishwar Shafin
Daniel Liu
Armin Töpfer
Aaron Wenger
William J. Rowell
Howard Yang
Andrew Carroll
Maria Nattestad
Learning Meaningful Representations of Life (LMRL) Workshop NeurIPS 2022
Preview abstract
Accurate genome sequencing can improve our understanding of biology and the genetic basis of disease. The standard approach for generating DNA sequences from PacBio instruments relies on HMM-based models. Here, we introduce Distilled DeepConsensus - a distilled transformer–encoder model for sequence correction, which improves upon the HMM-based methods with runtime constraints in mind. Distilled DeepConsensus is 1.3x faster and 1.5x smaller than its larger counterpart while improving the yield of high quality reads (Q30) over the HMM-based method by 1.69x (vs. 1.73x for larger model). With improved accuracy of genomic sequences, Distilled DeepConsensus improves downstream applications of genomic sequence analysis such as reducing variant calling errors by 39% (34% for larger model) and improving genome assembly quality by 3.8% (4.2% for larger model). We show that the representations learned by Distilled DeepConsensus are similar between faster and slower models.
View details
Preview abstract
Traditional methods that use a linear reference genome for analyses of whole genome sequencing data have been found to be inadequate for detection of structural variants, rare variation and variants that originate in high-complexity or repetitive regions of the human genome. Genome graphs help to systematically embed genetic variation from a population of samples into one reference structure. Though genome graphs have helped to reduce this mapping bias, there are still performance improvements that can be made. Here we present a workflow that uses population and pedigree genetic information to reduce reference bias and improve variant detection sensitivity as well as to generate a small list of candidate variants that are causal to rare genetic disorders at the genome scale.
View details
Ultra-Rapid Nanopore Whole Genome Genetic Diagnosis of Dilated Cardiomyopathy in an Adolescent With Cardiogenic Shock
John E. Gorzynski
Sneha D. Goenka
Kishwar Shafin
Dianna G. Fisk
Elizabeth Spiteri
Fritz J. Sedlazeck
Miten Jain
Jean Monlong
Trevor Pesout
Jonathan A Bernstein
Andrew Carroll
Kyla Dunn
Benedict Paten
Euan Ashley
Circulation: Genomic and Precision Medicine (2022)
Preview abstract
Rapid genetic diagnosis has the potential to guide clinical treatment in critically ill patients leading to improved prognosis and decreased health care costs. 1 Until recently, the turnaround time for whole genome diagnostic testing precluded its integration into critical care decision making (typical rapid whole genome sequencing clinical testing returns results in 5–7 days). Here, we describe a case of a teenager presenting with cardiogenic shock in whom a genetic diagnosis was made in under 12 hours using a new ultra-rapid long read whole genome sequencing assay and workflow. 2, 3 A 13-year-old male previously in good health presented to his primary care provider with a nocturnal dry cough, decreased appetite, intermittent chest pain, and fatigue. Thoracic radiographs showed cardiomegaly leading to echocardiography, which revealed a dilated left ventricle with an ejection fraction of 29%.
View details