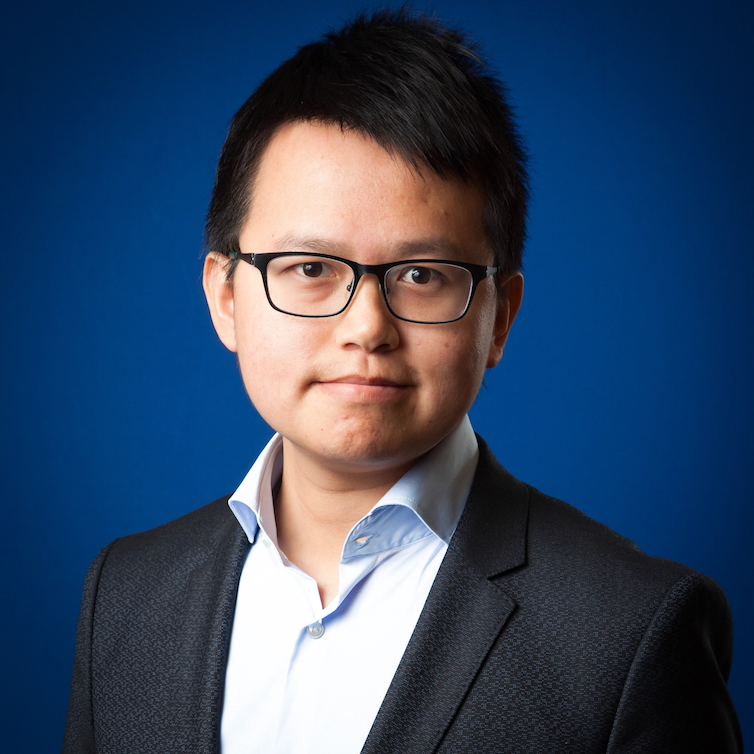
Heng-Tze Cheng
Heng-Tze Cheng is a Technical Lead Manager and Senior Staff Software Engineer on the Google Brain team, part of Google Research & AI. Heng-Tze currently leads a research team focusing on Neural Sequence Modeling research for Task-oriented Dialogues, Personalized Semantic Search, and Recommender Systems productionized across Google, such as Google Duplex Assistant, YouTube, and more. Heng-Tze also founded and led the Wide & Deep Learning project in TensorFlow, and has worked on large-scale machine learning platforms that are widely used for retrieval, ranking, and recommender systems.
Prior to joining Google in 2014, Heng-Tze received his Ph.D. from Carnegie Mellon University in 2013 and B.S. from National Taiwan University in 2008. His research interests range across machine learning, information retrieval, user behavior modeling, and human activity recognition.
Authored Publications
Sort By
Google
HyperPrompt: Prompt-based Task-Conditioning of Transformers
Cosmo Du
Steven Zheng
Vamsi Aribandi
Yi Tay
Yun He
Zhao Chen
Zhe Zhao
ICML (2022)
LaMDA: Language Models for Dialog Applications
Aaron Daniel Cohen
Alena Butryna
Alicia Jin
Apoorv Kulshreshtha
Ben Zevenbergen
Chung-ching Chang
Cosmo Du
Daniel De Freitas Adiwardana
Dehao Chen
Dmitry (Dima) Lepikhin
Erin Hoffman-John
Igor Krivokon
James Qin
Jamie Hall
Joe Fenton
Johnny Soraker
Kathy Meier-Hellstern
Maarten Paul Bosma
Marc Joseph Pickett
Marcelo Amorim Menegali
Marian Croak
Maxim Krikun
Noam Shazeer
Rachel Bernstein
Ravi Rajakumar
Ray Kurzweil
Romal Thoppilan
Steven Zheng
Taylor Bos
Toju Duke
Tulsee Doshi
Vincent Y. Zhao
Will Rusch
Yuanzhong Xu
Zhifeng Chen
arXiv (2022)
Mondegreen: A Post-Processing Solution to Speech Recognition Error Correction for Voice Search Queries
Ajit Apte
Ambarish Jash
Amol H Wankhede
Ankit Kumar
Ayooluwakunmi Jeje
Dima Kuzmin
Ellie Ka In Chio
Harry Fung
Jon Effrat
Nitin Jindal
Pei Cao
Senqiang Zhou
Sukhdeep S. Sodhi
Tameen Khan
Tarush Bali
KDD (2021)
Zero-Shot Transfer Learning for Query-Item Cold Start in Search Retrieval and Recommendations
Ankit Kumar
Cosmo Du
Dima Kuzmin
Ellie Chio
John Roberts Anderson
Li Zhang
Nitin Jindal
Pei Cao
Ritesh Agarwal
Tao Wu
Wen Li
CIKM (2020)
SlateQ: A Tractable Decomposition for Reinforcement Learning with Recommendation Sets
Vihan Jain
Jing Wang
Sanmit Narvekar
Ritesh Agarwal
Rui Wu
Proceedings of the Twenty-eighth International Joint Conference on Artificial Intelligence (IJCAI-19), Macau, China (2019), pp. 2592-2599
Reinforcement Learning for Slate-based Recommender Systems: A Tractable Decomposition and Practical Methodology
Vihan Jain
Jing Wang
Sanmit Narvekar
Ritesh Agarwal
Rui Wu
Morgane Lustman
Vince Gatto
Paul Covington
Jim McFadden
arXiv (2019)
TFX: A TensorFlow-Based Production-Scale Machine Learning Platform
Akshay Naresh Modi
Chiu Yuen Koo
Chuan Yu Foo
Clemens Mewald
Denis M. Baylor
Jarek Wilkiewicz
Levent Koc
Lukasz Lew
Martin A. Zinkevich
Mustafa Ispir
Neoklis Polyzotis
Steven Whang
Sudip Roy
Sukriti Ramesh
Vihan Jain
Xin Zhang
Zakaria Haque
KDD 2017
TensorFlow Estimators: Managing Simplicity vs. Flexibility in High-Level Machine Learning Frameworks
Cassandra Xia
Clemens Mewald
D. Sculley
George Roumpos
Illia Polosukhin
Jamie Alexander Smith
Jianwei Xie
Lichan Hong
Mustafa Ispir
Philip Daniel Tucker
Yuan Tang
Zakaria Haque
Proceedings of the 23th ACM SIGKDD International Conference on Knowledge Discovery and Data Mining, Halifax, Canada (2017)
Wide & Deep Learning for Recommender Systems
Levent Koc
Tal Shaked
Glen Anderson
Wei Chai
Mustafa Ispir
Rohan Anil
Zakaria Haque
Lichan Hong
Vihan Jain
Xiaobing Liu
Hemal Shah
arXiv:1606.07792 (2016)