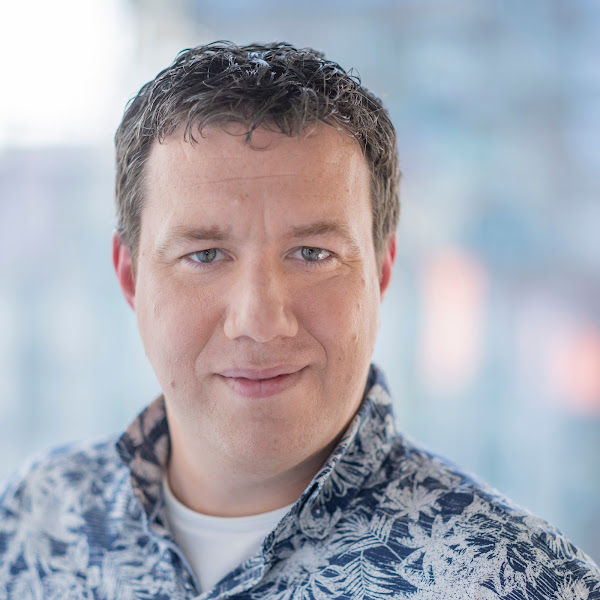
Daan van Esch
I work on internationalization for language technology at Google, harnessing machine learning and scalable infrastructure to bring support for new languages to products like Gboard and the Assistant. Our world has a wealth of linguistic diversity and it's a fascinating research challenge to build technology across so many different languages.
Authored Publications
Sort By
Now You See Me, Now You Don't: 'Poverty of the Stimulus' Problems and Arbitrary Correspondences in End-to-End Speech Models
Proceedings of the Second Workshop on Computation and Written Language (CAWL) 2024
Preview abstract
End-to-end models for speech recognition and speech synthesis have many benefits, but we argue they also face a unique set of challenges not encountered in conventional multi-stage hybrid systems, which relied on the explicit injection of linguistic knowledge through resources such as phonemic dictionaries and verbalization grammars. These challenges include handling words with unusual grapheme-to-phoneme correspondences, converting between written forms like ‘12’ and spoken forms such as ‘twelve’, and contextual disambiguation of homophones or homographs. We describe the mitigation strategies that have been used for these problems in end-to-end systems, either implicitly or explicitly, and call out that the most commonly used mitigation techniques are likely incompatible with newly emerging approaches that use minimal amounts of supervised audio training data. We review best-of-both-world approaches that allow the use of end-to-end models combined with traditional linguistic resources, which we show are increasingly straightforward to create at scale, and close with an optimistic outlook for bringing speech technologies to many more languages by combining these strands of research.
View details
Connecting Language Technologies with Rich, Diverse Data Sources Covering Thousands of Languages
Sebastian Ruder
Julia Kreutzer
Clara Rivera
Ishank Saxena
Proceedings of the 2024 Joint International Conference on Computational Linguistics, Language Resources and Evaluation (LREC-COLING 2024)
Preview abstract
Contrary to common belief, there are rich and diverse data sources available for many thousands of languages, which can be used to develop technologies for these languages. In this paper, we provide an overview of some of the major online data sources, the types of data that they provide access to, potential applications of this data, and the number of languages that they cover. Even this covers only a small fraction of the data that exists; for example, printed books are published in many languages but few online aggregators exist.
View details
Multimodal Modeling for Spoken Language Identification
Shikhar Bharadwaj
Sriram (Sri) Ganapathy
Sid Dalmia
Wei Han
Yu Zhang
Proceedings of 2024 IEEE International Conference on Acoustics, Speech and Signal Processing (ICASSP 2024)(2024)
Preview abstract
Spoken language identification refers to the task of automatically predicting the spoken language in a given utterance. Conventionally, it is modeled as a speech-based language identification task. Prior techniques have been constrained to a single modality; however in the case of video data there is a wealth of other metadata that may be beneficial for this task. In this work, we propose MuSeLI, a Multimodal Spoken Language Identification method, which delves into the use of various metadata sources to enhance language identification. Our study reveals that metadata such as video title, description and geographic location provide substantial information to identify the spoken language of the multimedia recording. We conduct experiments using two diverse public datasets of YouTube videos, and obtain state-of-the-art results on the language identification task. We additionally conduct an ablation study that describes the distinct contribution of each modality for language recognition.
View details
LinguaMeta: Unified Metadata for Thousands of Languages
Uche Okonkwo
Emily Drummond
Proceedings of the 2024 Joint International Conference on Computational Linguistics, Language Resources and Evaluation (LREC-COLING 2024)
Preview abstract
We introduce LinguaMeta, a unified resource for language metadata for thousands of languages, including language codes, names, number of speakers, writing systems, countries, official status, coordinates, and language varieties. The resources are drawn from various existing repositories and supplemented with our own research. Each data point is tagged for its origin, allowing us to easily trace back to and improve existing resources with more up-to-date and complete metadata. The resource is intended for use by researchers and organizations who aim to extend technology to thousands of languages.
View details
Handling Compounding in Mobile Keyboard Input
Andreas Christian Kabel
Keith B. Hall
David Rybach
arXiv cs.CL(2022)
Preview abstract
This paper proposes a framework to improve the typing experience of mobile users in morphologically rich languages. Smartphone keyboards typically support features such as input decoding, corrections and predictions that all rely on language models. For latency reasons, these operations happen on device, so the models are of limited size and cannot easily cover all the words needed by users for their daily tasks, especially in morphologically rich languages. In particular, the compounding nature of Germanic languages makes their vocabulary virtually infinite. Similarly, heavily inflecting and agglutinative languages (e.g. Slavic, Turkic or Finno-Ugric languages) tend to have much larger vocabularies than morphologically simpler languages, such as English or Mandarin. We propose to model such languages with automatically selected subword units annotated with what we call binding types, allowing the decoder to know when to bind subword units into words. We show that this method brings around 20% word error rate reduction in a variety of compounding languages. This is more than twice the improvement we previously obtained with a more basic approach, also described in the paper.
View details
Managing Transcription Data for Automatic Speech Recognition with Elpis
Ben Foley
Nay San
The Open Handbook of Linguistic Data Management, The MIT Press(2022)
Preview abstract
This chapter provides a ‘mid-level’ introduction to speech recognition technologies, with particular reference to Elpis (Foley et al., 2018), a tool designed for people with minimal computational experience to take advantage of modern speech recognition technologies in their language documentation transcription workflow. Elpis is intended to be used even in situations where there might not be the large quantities of previously-transcribed recordings typically required for training speech recognition systems. Even in language documentation contexts where people may only have one or two hours of transcribed recordings, using speech recognition can be beneficial to the process of transcription by providing an initial estimate which can be more quickly refined than typed from scratch.
View details
Preview abstract
Almost none of the 2,000+ languages spoken in Africa have widely available automatic speech recognition systems, and the required data is also only available for a few languages. We have experimented with two techniques which may provide pathways to large vocabulary speech recognition for African languages: multilingual modeling and self-supervised learning. We gathered available open source data and collected data for 15 languages, and trained experimental models using these techniques. Our results show that pooling the small amounts of data available in multilingual end-to-end models, and pre-training on unsupervised data can help improve speech recognition quality for many African languages.
View details
Quality at a Glance: An Audit of Web-Crawled Multilingual Datasets
Julia Kreutzer
Lisa Wang
Ahsan Wahab
Nasanbayar Ulzii-Orshikh
Allahsera Auguste Tapo
Nishant Subramani
Artem Sokolov
Claytone Sikasote
Monang Setyawan
Supheakmungkol Sarin
Sokhar Samb
Benoît Sagot
Clara E. Rivera
Annette Rios
Isabel Papadimitriou
Salomey Osei
Pedro Javier Ortiz Suárez
Iroro Fred Ọ̀nọ̀mẹ̀ Orife
Kelechi Ogueji
Rubungo Andre Niyongabo
Toan Nguyen
Mathias Müller
André Müller
Shamsuddeen Hassan Muhammad
Nanda Muhammad
Ayanda Mnyakeni
Jamshidbek Mirzakhalov
Tapiwanashe Matangira
Colin Leong
Nze Lawson
Yacine Jernite
Mathias Jenny
Bonaventure F. P. Dossou
Sakhile Dlamini
Nisansa de Silva
Sakine Çabuk Ballı
Stella Biderman
Alessia Battisti
Ahmed Baruwa
Pallavi Baljekar
Israel Abebe Azime
Ayodele Awokoya
Duygu Ataman
Orevaoghene Ahia
Oghenefego Ahia
Sweta Agrawal
Mofetoluwa Adeyemi
TACL(2022)
Preview abstract
With the success of large-scale pre-training and multilingual modeling in Natural Language Processing (NLP), recent years have seen a proliferation of large, web-mined text datasets covering hundreds of languages. However, to date there has been no systematic analysis of the quality of these publicly available datasets, or whether the datasets actually contain content in the languages they claim to represent. In this work, we manually audit the quality of 205 language-specific corpora released with five major public datasets (CCAligned, ParaCrawl, WikiMatrix, OSCAR, mC4), and audit the correctness of language codes in a sixth (JW300). We find that lower-resource corpora have systematic issues: at least 15 corpora are completely erroneous, and a significant fraction contains less than 50% sentences of acceptable quality. Similarly, we find 82 corpora that are mislabeled or use nonstandard/ambiguous language codes. We demonstrate that these issues are easy to detect even for non-speakers of the languages in question, and supplement the human judgements with automatic analyses. Inspired by our analysis, we recommend techniques to evaluate and improve multilingual corpora and discuss the risks that come with low-quality data releases.
View details
Accented Speech Recognition: Benchmarking, Pre-training, and Diverse Data
Zhehuai Chen
Chung-Cheng Chiu
Pavel Golik
Wei Han
Levi King
Suzan Schwartz
(2022)
Preview abstract
Building inclusive speech recognition systems is a crucial step towards developing technologies that speakers of all language varieties can use. Therefore, ASR systems must work for everybody independently of the way they speak. To accomplish this goal, there should be available data sets representing language varieties, and also an understanding of model configuration that is the most helpful in achieving robust understanding of all types of speech. However, there are not enough data sets for accented speech, and for the ones that are already available, more training approaches need to be explored to improve the quality of accented speech recognition. In this paper, we discuss recent progress towards developing more inclusive ASR systems, namely, the importance of building new data sets representing linguistic diversity, and exploring novel training approaches to improve performance for all users. We address recent directions within benchmarking ASR systems for accented speech, measure the effects of wav2vec 2.0 pre-training on accented speech recognition, and highlight corpora relevant for diverse ASR evaluations.
View details
XTREME-S: Evaluating Cross-lingual Speech Representations
Clara E. Rivera
Mihir Sanjay Kale
Sebastian Ruder
Simran Khanuja
Ye Jia
Yu Zhang
Proc. Interspeech 2022
Preview abstract
We introduce \xtremes, a new benchmark to evaluate universal cross-lingual speech representations in many languages. XTREME-S covers four task families: speech recognition, classification, retrieval and speech-to-text translation. Covering 102 languages from 10+ language families, 3 different domains and 4 task families, XTREME-S aims to simplify multilingual speech representation evaluation, as well as catalyze research in ``universal'' speech representation learning. This paper describes the new benchmark and establishes the first speech-only and speech-text baselines using XLS-R and mSLAM on all downstream tasks. We motivate the design choices and detail how to use the benchmark. The code and pre-processing scripts will be made publicly available.\footnote{\small\url{https://huggingface.co/datasets/google/xtreme_s}}
View details