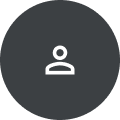
Ceslee Montgomery
Research Areas
Authored Publications
Sort By
ImageInWords: Unlocking Hyper-Detailed Image Descriptions
Andrew Bunner
Ranjay Krishna
(2024)
Preview abstract
Despite the longstanding adage "an image is worth a thousand words," creating accurate and hyper-detailed image descriptions for training Vision-Language models remains challenging.
Current datasets typically have web-scraped descriptions that are short, low-granularity, and often contain details unrelated to the visual content. As a result, models trained on such data generate descriptions replete with missing information, visual inconsistencies, and hallucinations. To address these issues, we introduce ImageInWords (IIW), a carefully designed human-in-the-loop annotation framework for curating hyper-detailed image descriptions and a new dataset resulting from this process.
We validate the framework through evaluations focused on the quality of the dataset and its utility for fine-tuning with considerations for readability, comprehensiveness, specificity, hallucinations, and human-likeness. Our dataset significantly improves across these dimensions compared to recently released datasets (+66%) and GPT-4V outputs (+48%). Furthermore, models fine-tuned with IIW data excel by +31% against prior work along the same human evaluation dimensions. Given our fine-tuned models, we also evaluate text-to-image generation and vision-language reasoning. Our model's descriptions can generate images closest to the original, as judged by both automated and human metrics. We also find our model produces more compositionally rich descriptions, outperforming the best baseline by up to 6% on ARO, SVO-Probes, and Winoground datasets.
View details
PaLI-X: On Scaling up a Multilingual Vision and Language Model
Josip Djolonga
Piotr Padlewski
Basil Mustafa
Carlos Riquelme
Sebastian Goodman
Yi Tay
Siamak Shakeri
Daniel Salz
Michael Tschannen
Mandar Joshi
Filip Pavetić
Gang Li
Anurag Arnab
Yuanzhong Xu
Keran Rong
Neil Houlsby
Computer Vision and Pattern Recognition Conference (CVPR) (2024)
Preview abstract
We explore the boundaries of scaling up a multilingual vision and language model, both in terms of size of the components and the breadth of its training task mixture. Our model achieves new levels of performance on a wide-range of varied and complex tasks, including multiple image-based captioning and question-answering tasks, image-based document understanding and few-shot (in-context) learning, as well as object detection, video question answering, and video captioning. Our model advances the state-of-the-art on most vision-and-language benchmarks considered (20+ of them). Finally, we observe emerging capabilities, such as complex counting and multilingual object detection, tasks that are not explicitly in the training mix.
View details
Imagen Editor and EditBench: Advancing and Evaluating Text-Guided Image Inpainting
Su Wang
Chitwan Saharia
Shai Noy
Stefano Pellegrini
Sarah Laszlo
Mohammad Norouzi
Peter Anderson
William Chan
CVPR (2023)
Preview abstract
Text-guided image editing can have a transformative impact in supporting creative applications. A key challenge is to generate edits that are faithful to the input text prompt, while consistent with the input image. We present Imagen Editor, a cascaded diffusion model, built by fine-tuning Imagen on text-guided image inpainting. Imagen Editor's edits are faithful to the text prompts, which is accomplished by incorporating object detectors for proposing inpainting masks during training. In addition, text-guided image inpainting captures fine details in the input image by conditioning the cascaded pipeline on the original high resolution image. To improve qualitative and quantitative evaluation, we introduce EditBench, a systematic benchmark for text-guided image inpainting. EditBench evaluates inpainting edits on natural and generated images exploring objects, attributes, and scenes. Through extensive human evaluation on EditBench, we find that object-masking during training leads to across-the-board improvements in text-image alignment -- such that Imagen Editor is preferred over DALL-E 2 and Stable Diffusion -- and, as a cohort, these models are better at object-rendering than text-rendering, and handle material/color/size attributes better than count/shape attributes.
View details
Less is More: Generating Grounded Navigation Instructions from Landmarks
Su Wang
Jordi Orbay
Vighnesh Birodkar
Izzeddin Gur
Peter Anderson
CVPR (2022) (to appear)
Preview abstract
We study the automatic generation of navigation instructions from 360-degree images captured on indoor routes. Existing generators suffer from poor visual grounding, causing them to rely on language priors and hallucinate objects. Our MARKY-MT5 system addresses this by focusing on visual landmarks; it comprises a first stage landmark detector and a second stage generator -- a multimodal, multilingual, multitask encoder-decoder. To train it, we bootstrap grounded landmark annotations on top of the Room-across-Room (RxR) dataset. Using text parsers, weak supervision from RxR's pose traces, and a multilingual image-text encoder trained on 1.8b images, we identify 1.1m English, Hindi and Telugu landmark descriptions and ground them to specific regions in panoramas. On Room-to-Room, human wayfinders obtain success rates (SR) of 71% following MARKY-MT5's instructions, just shy of their 75% SR following human instructions -- and well above SRs with other generators. Evaluations on RxR's longer, diverse paths obtain 61-64% SRs on three languages. Generating such high-quality navigation instructions in novel environments is a step towards conversational navigation tools and could facilitate larger-scale training of instruction-following agents.
View details