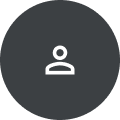
Quang Anh Duong
Authored Publications
Sort By
Google
LLM-based Lossless Text Simplification and its Effect on User Comprehension and Cognitive Load
Theo Guidroz
Diego Ardila
Jimmy Li
Adam Mansour
Paul Jhun
Nina Gonzalez
Xiang Ji
Mike Sanchez
Miguel Ángel Garrido
Divyansh Choudhary
Jay Hartford
Georgina Xu
Henry Serrano
Yifan Wang
Jeff Shaffer
Eric (Yifan) Cao
Sho Fujiwara
Peggy Bui
arXiv (2025)
Searching for Dermatology Information Online using Images vs Text: a Randomized Study
Jay Hartford
Natalie Salaets
Kimberley Raiford
Jay Nayar
Dounia Berrada
Harsh Kharbanda
Lou Wang
Peggy Bui
medRxiv (2024)
PathAlign: A vision–language model for whole slide images in histopathology
Lin Yang
Shawn Xu
Abbi Ward
Niels Olson
Arash Mohtashamian
Shravya Shetty
Daniel Golden
Yun Li
(2024)
Searching for Dermatology Information Online using Images vs Text: a Randomized Study
Jay Hartford
Natalie Salaets
Kimberley Raiford
Jay Nayar
Dounia Berrada
Harsh Kharbanda
Lou Wang
Peggy Bui
medRxiv (2024)
Iterative quality control strategies for expert medical image labeling
Sonia Phene
Abigail Huang
Rebecca Ackermann
Olga Kanzheleva
Caitlin Taggart
Proceedings of the AAAI Conference on Human Computation and Crowdsourcing (2021)