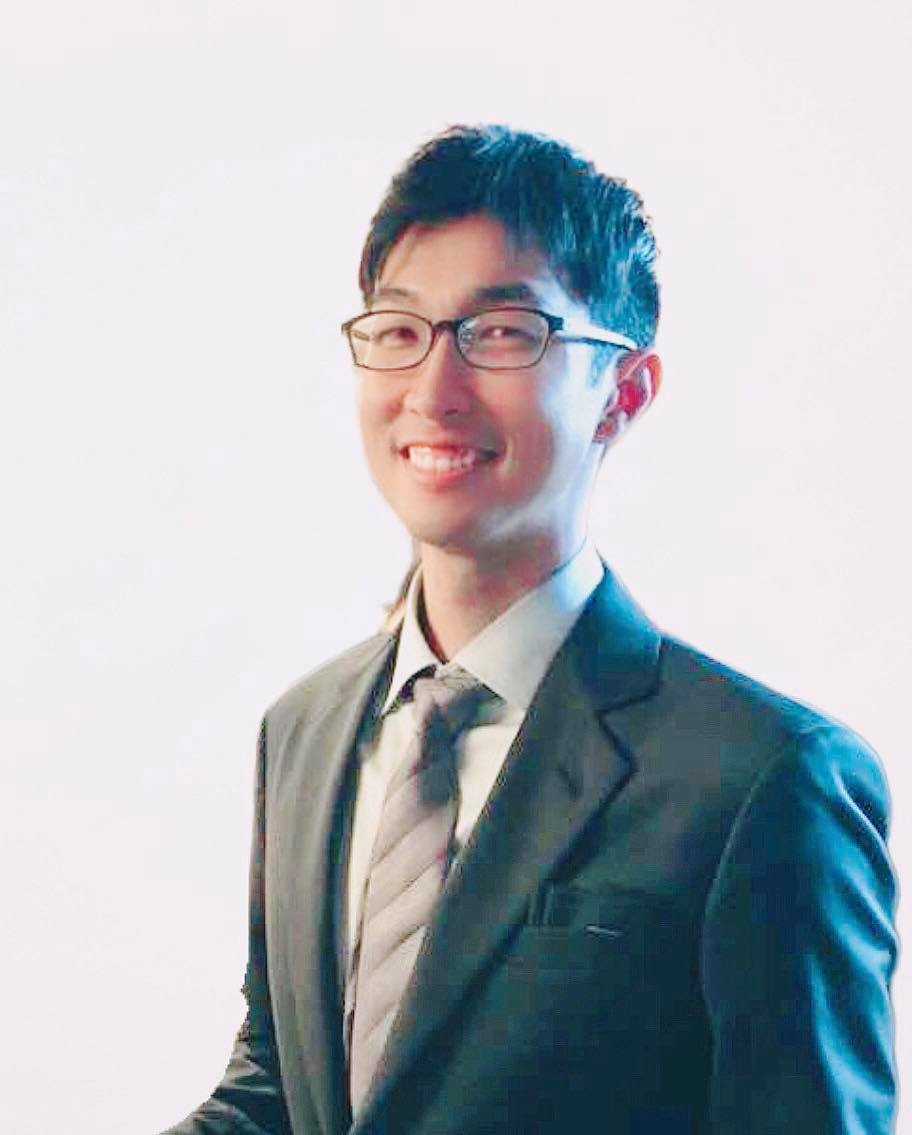
Shuo-yiin Chang
Authored Publications
Sort By
Google
Text Injection for Capitalization and Turn-taking Prediction In ASR Models
Weiran Wang
Zhong Meng
Interspeech 2023 (2023)
Unified End-to-End Speech Recognition and Endpointing for Fast and Efficient Speech Systems
Chao Zhang
IEEE Spoken Language Technology Workshop (2022)
E2E Segmenter: Joint Segmenting and Decoding for Long-Form ASR
David Rybach
Cal Peyser
Zhiyun Lu
Interspeech 2022 (2022) (to appear)
An Efficient Streaming Non-Recurrent On-Device End-to-End Model with Improvements to Rare-Word Modeling
Rami Botros
Ruoming Pang
David Johannes Rybach
James Qin
Quoc-Nam Le-The
Anmol Gulati
Cal Peyser
Chung-Cheng Chiu
Emmanuel Guzman
Jiahui Yu
Qiao Liang
Wei Li
Yonghui Wu
Yu Zhang
Interspeech (2021) (to appear)
FastEmit: Low-latency Streaming ASR with Sequence-level Emission Regularization
Jiahui Yu
Chung-Cheng Chiu
Wei Han
Anmol Gulati
Yonghui Wu
Ruoming Pang
ICASSP 2021
Towards fast and accurate streaming end-to-end ASR
Ruoming Pang
Yonghui Wu
Proc. ICASSP (2020)
A Streaming On-Device End-to-End Model Surpassing Server-Side Conventional Model Quality and Latency
Ruoming Pang
Antoine Bruguier
Wei Li
Raziel Alvarez
Zhifeng Chen
Chung-Cheng Chiu
David Garcia
Kevin Hu
Minho Jin
Qiao Liang
Cal Peyser
David Rybach
(June) Yuan Shangguan
Yash Sheth
Mirkó Visontai
Yonghui Wu
Yu Zhang
Ding Zhao
ICASSP (2020)
Low Latency Speech Recognition using End-to-End Prefetching
David Johannes Rybach
Wei Li
Interspeech 2020 (to appear)