Climate and sustainability
Leveraging machine learning (ML) and artificial intelligence (AI) to address climate change and help build a sustainable future for all.
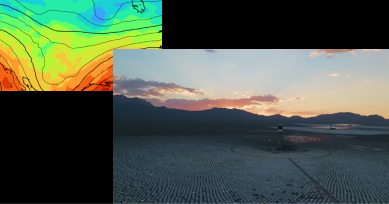
Leveraging machine learning (ML) and artificial intelligence (AI) to address climate change and help build a sustainable future for all.
About the team
Climate change is an urgent threat to humanity with far-reaching societal and economic consequences. Our climate and sustainability teams harness the power of AI to address this challenge, as part of Google's long-standing commitment to climate action.
AI has expanded the type of information we can apply to deep computing power and unlocked an increasing number of methods for interpreting information and creating groundbreaking innovations. It enables us to identify previously impossible solutions to climate challenges — from reducing greenhouse gas emissions in cities to building models that improve our ability to predict and respond to climate-driven natural disasters.
Google Research is leading multiple climate and sustainability efforts in various stages of development, and we continue to explore innovations to accelerate progress in this space. As part of our mission to build a safer, more sustainable future for all, we collaborate closely with cities, governments, startups and aid organizations.
Our Climate and Sustainability team is global, with researchers, partners and projects spanning the US, Europe, Africa, Latin America and Asia. Learn more below about how we are using AI to improve the lives of billions of people worldwide.
Team focus summaries
Featured publications
Highlighted work
-
Green LightOptimizing traffic lights to reduce vehicle emissions in cities, helping to mitigate climate change and improve urban mobility.
-
Project ContrailsLeveraging computer vision to build a cost-effective and scalable method for helping to mitigate aviation’s climate impact.
-
Flood Forecasting initiativeUsing AI models to predict when floods will occur and help make critical flood forecasting information accessible to all.
-
Startups for Sustainable DevelopmentMentoring and technological support for impact startups working towards the Sustainable Development Goals.
-
Wildfire detection and predictionUsing AI to provide accurate wildfire information to affected communities and fire authorities
-
Fuel-efficient routingProviding users with the most fuel-efficient route to their destination based on factors such as engine type and traffic conditions.