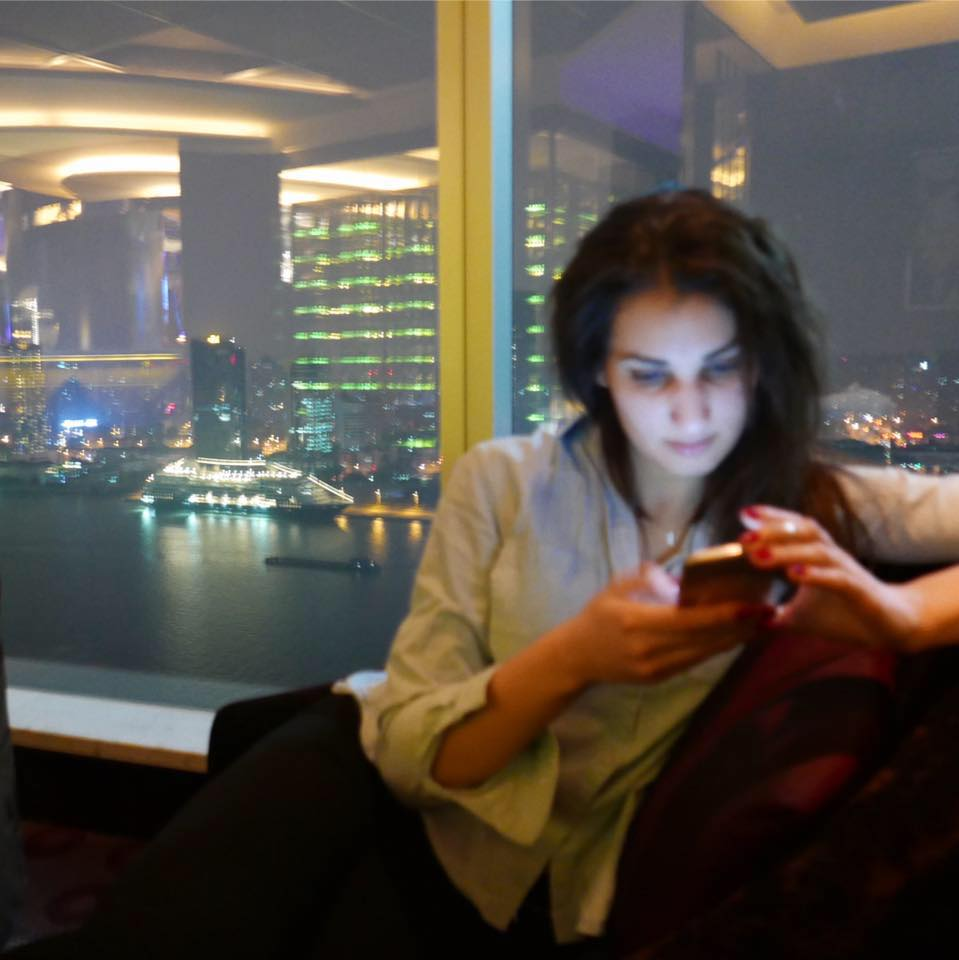
Deborah Cohen
Deborah Cohen received the B.Sc. degree in electrical engineering (summa cum laude) in 2010 and the Ph.D. degree in electrical engineering (signal processing) in 2016 from the Technion - Israel Institute of Technology, Haifa, in 2010. Since 2010, she has been a Project Supervisor with the Signal and Image Processing Lab, the High Speed Digital Systems Lab, the Communications Lab and the Signal Acquisition, Modeling and Processing Lab (SAMPL), at the Electrical Engineering Department, Technion. In 2011, Ms. Cohen was awarded the Meyer Foundation Excellence prize. She received the Sandor Szego Award and the Vivian Konigsberg Award for Excellence in Teaching from 2012 to 2016, the David and Tova Freud and Ruth Freud-Brendel Memorial Scholarship in 2014 and the Muriel and David Jacknow Award for Excellence in Teaching in 2015. Since 2014, Ms. Cohen is an Azrieli Fellow. She is currently a research scientist in the Clair team in Google Israel. Her research interests include theoretical aspects of signal processing, compressed sensing, reinforcement learning and machine learning for dialogues.
Research Areas
Authored Publications
Sort By
Google
AI Increases Global Access to Reliable Flood Forecasts
Asher Metzger
Dana Weitzner
Frederik Kratzert
Guy Shalev
Martin Gauch
Sella Nevo
Shlomo Shenzis
Tadele Yednkachw Tekalign
Vusumuzi Dube
arXiv (2023)
Dynamic Composition for Conversational Domain Exploration
Eran Ofek
Sagie Israel Pudinsky
Asaf Revach
Shimi Salant
The Web Conference, ACM (2020), 872–883
Sparse imitation learning for text based games with combinatorial action spaces
Chen Tessler
Tom Zahavy
Daniel J. Mankowitz
Shie Mannor
The Multidisciplinary Conference on Reinforcement Learning and Decision Making (RLDM) (2019)