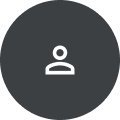
Sayali Kulkarni
Authored Publications
Google Publications
Other Publications
Sort By
CoMSum: Dataset and Neural Model for Contextual Multi-Document Summarization
Sheide Chammas
Wan Zhu
International Conference on Document Analysis and Recognition, International Conference on Document Analysis and Recognition (2021)
Preview abstract
Summarization is the task of compressing source document(s) into coherent and succinct passages. Query-based (contextual) multi-document summarization (qMDS) is a variant that targets summaries to specific informational needs with queries providing additional contexts. Progress in qMDS has been hampered by limited availability of corresponding types of datasets. In this work, we make two contributions. First, we develop an automatic approach for creating both extractive and abstractive qMDS examples from existing language resources. We use this approach to create \qmds, a qMDS dataset for public use. Secondly, to validate the utility of \qmds, we propose a neural model for extractive summarization that exploits the hierarchical nature of the input from multiple documents. It also infuses queries into the modeling to extract query-specific summaries. The experimental results show that modeling the queries and the multiple documents hierarchically improve the performance of qMDS on this datasets. This is consitent with our intuition and supports using \qmds for developing learning methods for qMDS.
View details
Dynamic Composition for Conversational Domain Exploration
Eran Ofek
Sagie Israel Pudinsky
Asaf Revach
Shimi Salant
The Web Conference, ACM (2020), 872–883
Preview abstract
We study conversational domain exploration (CODEX), where the user’s goal is to enrich her knowledge of a given domain by conversing with an informative bot. Such conversations should be well grounded in high-quality domain knowledge as well as engaging and open-ended. A CODEX bot should be proactive and introduce relevant information even if not directly asked for by the user. The bot should also appropriately pivot the conversation to undiscovered regions of the domain. To address these dialogue characteristics, we introduce a novel approach termed dynamic composition that decouples candidate content generation from the flexible composition of bot responses. This allows the bot to control the source, correctness and quality of the offered content, while achieving flexibility via a dialogue manager that selects the most appropriate contents in a compositional manner. We implemented a CODEX bot based on dynamic composition and integrated it into the Google Assistant. As an example domain, the bot conversed about the NBA basketball league in a seamless experience, such that users were not aware whether they were conversing with the vanilla system or the one augmented with our CODEX bot. Results are positive and offer insights into what makes for a good conversation. To the best of our knowledge, this is the first real user experiment of open-ended dialogues as part of a commercial
assistant system.
View details
Preview abstract
Wildlife monitoring is an essential part of nature conservation. Traditionally this has been done by human experts working in the field limiting the reach and extent. With the advances in AI and computer vision over the past decade, it can be put to an effective use for this purpose. There is gigantic amount of data collected from camera trap images over the years, tagged by experts. It can be used to build models to automatically tag new images efficiently. We outline the data collection effort from various conservation partners to unify the same for training AI-based classification models using deep neural networks. Evaluation of widely-used models trained on 2.9M images across 465 fine grained species enable us to achieve an accuracy of 83.92\%. We then outline work in progress to improve these models by addressing the common challenges and how we can effectively use inherent characteristics of camera trap data thus reducing the load on human experts and contributing to nature conservation effort by effectively monitoring the wildlife.
View details
Learning Dense Representations for Entity Retrieval
Larry Lansing
Diego Garcia-Olano
CoNLL (2019)
Preview abstract
We show that it is feasible to perform entity linking by training a dual encoder (two-tower) model that encodes mentions and entities in the same dense vector space, where candidate entities are retrieved by approximate nearest neighbor search. Unlike prior work, this setup does not rely on an alias table followed by a re-ranker, and is thus the first fully learned entity retrieval model. We show that our dual encoder, trained using only anchor-text links in Wikipedia, outperforms discrete alias table and BM25 baselines, and is competitive with the best comparable results on the standard TACKBP-2010 dataset. In addition, it can retrieve candidates extremely fast, and generalizes well to a new dataset derived from Wikinews. On the modeling side, we demonstrate the dramatic value of an unsupervised negative mining algorithm for this task.
View details
Seq2Slate: Re-ranking and Slate Optimization with RNNs
Irwan Bello
Sagar Jain
Alan Mackey
arXiv (2019)
Preview abstract
Ranking is a central task in machine learning and information retrieval. In this task, it is especially important to present the user with a slate of items that is appealing as a whole. This in turn requires taking into account interactions between items, since intuitively, placing an item on the slate affects the decision of which other items should be placed alongside it. In this work, we propose a sequence-to-sequence model for ranking called seq2slate. At each step, the model predicts the next "best" item to place on the slate given the items already selected. The sequential nature of the model allows complex dependencies between the items to be captured directly in a flexible and scalable way. We show how to learn the model end-to-end from weak supervision in the form of easily obtained click-through data. We further demonstrate the usefulness of our approach in experiments on standard ranking benchmarks as well as in a real-world recommendation system.
View details
No Results Found