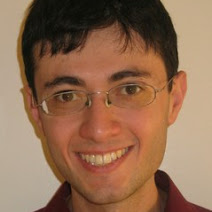
Avinatan Hassidim
Authored Publications
Sort By
Preview abstract
Computing efficient traffic signal plans is often based on the amount of traffic in an intersection, its distribution over the various intersection movements and hours as well as on performance metrics such as traffic delay. In their simple and typical form plans are fixed in the same hour over weekdays. This allows low operation costs without the necessity for traffic detection and monitoring tools. A critical factor on the potential efficiency of such plans is the similarity of traffic patterns over the days along each of the intersection movements. We refer to such similarity as the traffic stability of the intersection and define simple metrics to measure it based on traffic volume and traffic delay. In this paper, we propose an automatic probe data based method, for city-wide estimation of traffic stability. We discuss how such measures can be used for signal planning such as in selecting plan resolution or as an indication as which intersections can benefit from dynamic but expensive traffic detection tools. We also identify events of major changes in traffic characteristics of an intersection. We demonstrate the framework by using real traffic statistics to study the traffic stability in the city of Haifa along its 162 intersections. We study the impact of the time of day on the stability, detect major changes in traffic and find intersections with high and low stability.
View details
Alignment of brain embeddings and artificial contextual embeddings in natural language points to common geometric patterns
Ariel Goldstein
Avigail Grinstein-Dabush
Haocheng Wang
Zhuoqiao Hong
Bobbi Aubrey
Samuel A. Nastase
Zaid Zada
Eric Ham
Harshvardhan Gazula
Eliav Buchnik
Werner Doyle
Sasha Devore
Patricia Dugan
Roi Reichart
Daniel Friedman
Orrin Devinsky
Adeen Flinker
Uri Hasson
Nature Communications (2024)
Preview abstract
Contextual embeddings, derived from deep language models (DLMs), provide
a continuous vectorial representation of language. This embedding space
differs fundamentally from the symbolic representations posited by traditional
psycholinguistics. We hypothesize that language areas in the human brain,
similar to DLMs, rely on a continuous embedding space to represent language.
To test this hypothesis, we densely record the neural activity patterns in the
inferior frontal gyrus (IFG) of three participants using dense intracranial arrays
while they listened to a 30-minute podcast. From these fine-grained spatiotemporal neural recordings, we derive a continuous vectorial representation
for each word (i.e., a brain embedding) in each patient. We demonstrate that
brain embeddings in the IFG and the DLM contextual embedding space have
common geometric patterns using stringent zero-shot mapping. The common
geometric patterns allow us to predict the brain embedding of a given left-out
word in IFG based solely on its geometrical relationship to other nonoverlapping words in the podcast. Furthermore, we show that contextual
embeddings better capture the geometry of IFG embeddings than static word
embeddings. The continuous brain embedding space exposes a vector-based
neural code for natural language processing in the human brain.
View details
Factually Consistent Summarization via Reinforcement Learning with Textual Entailment Feedback
Paul Roit
Johan Ferret
Geoffrey Cideron
Matthieu Geist
Sertan Girgin
Léonard Hussenot
Nikola Momchev
Piotr Stanczyk
Nino Vieillard
Olivier Pietquin
Proceedings of the 61st Annual Meeting of the Association for Computational Linguistics, Association for Computational Linguistics(2023), 6252–6272
Preview abstract
Despite the seeming success of contemporary grounded text generation systems, they often tend to generate factually inconsistent text with respect to their input. This phenomenon is emphasized in tasks like summarization, in which the generated summaries should be corroborated by their source article. In this work we leverage recent progress on textual entailment models to directly address this problem for abstractive summarization systems. We use reinforcement learning with reference-free, textual-entailment rewards to optimize for factual consistency and explore the ensuing trade-offs, as improved consistency may come at the cost of less informative or more extractive summaries. Our results, according to both automatic metrics and human evaluation, show that our method considerably improves the faithfulness, salience and conciseness of the generated summaries.
View details
A Neural Encoder for Earthquake Rate Forecasting
Oleg Zlydenko
Brendan Meade
Alexandra Sharon Molchanov
Sella Nevo
Yohai bar Sinai
Scientific Reports(2023)
Preview abstract
Forecasting the timing of earthquakes is a long-standing challenge. Moreover, it is still debated how to formulate this problem in a useful manner, or to compare the predictive power of different models.
Here, we develop a versatile neural encoder of earthquake catalogs, and apply it to the fundamental problem of earthquake rate prediction, in the spatio-temporal point process framework. The epidemic
type aftershock sequence model (ETAS) effectively learns a small number of parameters to constrain assumed functional forms for the space and time relationships of earthquake sequences (e.g., Omori-Utsu law). Here we introduce learned spatial and temporal embeddings for point process earthquake forecast models that capture complex correlation structures. We demonstrate the generality of this neural representation as compared with ETAS model using train-test data splits and how it enables the incorporation of additional geophysical information. In rate prediction tasks, the generalized model shows > 4% improvement in information gain per earthquake and the simultaneous learning of anisotropic spatial structures analogous to fault traces. The trained network can be also used to perform short-term prediction tasks, showing similar improvement while providing a 1,000-fold reduction in run-time.
View details
AI Increases Global Access to Reliable Flood Forecasts
Asher Metzger
Dana Weitzner
Frederik Kratzert
Guy Shalev
Martin Gauch
Sella Nevo
Shlomo Shenzis
Tadele Yednkachw Tekalign
Vusumuzi Dube
arXiv(2023)
Preview abstract
Floods are one of the most common natural disasters, with a disproportionate impact in developing countries that often lack dense streamflow gauge networks. Accurate and timely warnings are critical for mitigating flood risks, but hydrological simulation models typically must be calibrated to long data records in each watershed. Here we show that AI-based forecasting achieves reliability in predicting extreme riverine events in ungauged watersheds at up to a 5-day lead time that is similar to or better than the reliability of nowcasts (0-day lead time) from a current state of the art global modeling system (the Copernicus Emergency Management Service Global Flood Awareness System). Additionally, we achieve accuracies over 5-year return period events that are similar to or better than current accuracies over 1-year return period events. This means that AI can provide flood warnings earlier and over larger and more impactful events in ungauged basins. The model developed in this paper was incorporated into an operational early warning system that produces publicly available (free and open) forecasts in real time in over 80 countries. This work highlights a need for increasing the availability of hydrological data to continue to improve global access to reliable flood warnings.
View details
Caravan - A global community dataset for large-sample hydrology
Frederik Kratzert
Nans Addor
Tyler Erickson
Martin Gauch
Lukas Gudmundsson
Daniel Klotz
Sella Nevo
Guy Shalev
Scientific Data, 10(2023), pp. 61
Preview abstract
High-quality datasets are essential to support hydrological science and modeling. Several CAMELS (Catchment Attributes and Meteorology for Large-sample Studies) datasets exist for specific countries or regions, however these datasets lack standardization, which makes global studies difficult. This paper introduces a dataset called Caravan (a series of CAMELS) that standardizes and aggregates seven existing large-sample hydrology datasets. Caravan includes meteorological forcing data, streamflow data, and static catchment attributes (e.g., geophysical, sociological, climatological) for 6830 catchments. Most importantly, Caravan is both a dataset and open-source software that allows members of the hydrology community to extend the dataset to new locations by extracting forcing data and catchment attributes in the cloud. Our vision is for Caravan to democratize the creation and use of globally-standardized large-sample hydrology datasets. Caravan is a truly global open-source community resource.
View details
Structured Understanding of Assessment and Plans in Clinical Documentation
Doron Yaya-Stupp
Ronnie Barequet
I-Ching Lee
Eyal Oren
Eran Ofek
Alvin Rajkomar
medRxiv(2022)
Preview abstract
Physicians record their detailed thought-processes about diagnoses and treatments as unstructured text in a section of a clinical note called the assessment and plan. This information is more clinically rich than structured billing codes assigned for an encounter but harder to reliably extract given the complexity of clinical language and documentation habits. We describe and release a dataset containing annotations of 579 admission and progress notes from the publicly available and de-identified MIMIC-III ICU dataset with over 30,000 labels identifying active problems, their assessment, and the category of associated action items (e.g. medication, lab test). We also propose deep-learning based models that approach human performance, with a F1 score of 0.88. We found that by employing weak supervision and domain specific data-augmentation, we could improve generalization across departments and reduce the number of human labeled notes without sacrificing performance.
View details
Flood forecasting with machine learning models in an operational framework
Asher Metzger
Chen Barshai
Dana Weitzner
Frederik Kratzert
Gregory Begelman
Guy Shalev
Hila Noga
Moriah Royz
Niv Giladi
Ronnie Maor
Sella Nevo
Yotam Gigi
HESS(2022)
Preview abstract
Google’s operational flood forecasting system was developed to provide accurate real-time flood warnings to agencies and the public, with a focus on riverine floods in large, gauged rivers. It became operational in 2018 and has since expanded geographically. This forecasting system consists of four subsystems: data validation, stage forecasting, inundation modeling, and alert distribution. Machine learning is used for two of the subsystems. Stage forecasting is modeled with the Long Short-Term Memory (LSTM) networks and the Linear models. Flood inundation is computed with the Thresholding and the Manifold models, where the former computes inundation extent and the latter computes both inundation extent and depth. The Manifold model, presented here for the first time, provides a machine-learning alternative to hydraulic modeling of flood inundation. When evaluated on historical data, all models achieve sufficiently high-performance metrics for operational use. The LSTM showed higher skills than the Linear model, while the Thresholding and Manifold models achieved similar performance metrics for modeling inundation extent. During the 2021 monsoon season, the flood warning system was operational in India and Bangladesh, covering flood-prone regions around rivers with a total area of 287,000 km2, home to more than 350M people. More than 100M flood alerts were sent to affected populations, to relevant authorities, and to emergency organizations. Current and future work on the system includes extending coverage to additional flood-prone locations, as well as improving modeling capabilities and accuracy.
View details
Using generative AI to investigate medical imagery models and datasets
Charles Lau
Chloe Nichols
Doron Yaya-Stupp
Heather Cole-Lewis
Ilana Traynis
https://arxiv.org/(2022)
Preview abstract
AI models have shown promise in performing many medical imaging tasks. However, our ability to explain what signals these models learn from the training data is severely lacking. Explanations are needed in order to increase the trust of doctors in AI-based models, especially in domains where AI prediction capabilities surpass those of humans. Moreover, such explanations could enable novel scientific discovery by uncovering signals in the data that aren’t yet known to experts. In this paper, we present a method for automatic visual explanations that can help achieve these goals by generating hypotheses of what visual signals in the images are correlated with the task. We propose the following 4 steps: (i) Train a classifier to perform a given task to assess whether the imagery indeed contains signals relevant to the task; (ii) Train a StyleGAN-based image generator with an architecture that enables guidance by the classifier (“StylEx”); (iii) Automatically detect and extract the top visual attributes that the classifier is sensitive to. Each of these attributes can then be independently modified for a set of images to generate counterfactual visualizations of those attributes (i.e. what that image would look like with the attribute increased or decreased); (iv) Present the discovered attributes and corresponding counterfactual visualizations to a multidisciplinary panel of experts to formulate hypotheses for the underlying mechanisms with consideration to social and structural determinants of health (e.g. whether the attributes correspond to known patho-physiological or socio-cultural phenomena, or could be novel discoveries) and stimulate future research. To demonstrate the broad applicability of our approach, we demonstrate results on eight prediction tasks across three medical imaging modalities – retinal fundus photographs, external eye photographs, and chest radiographs. We showcase examples where many of the automatically-learned attributes clearly capture clinically known features (e.g., types of cataract, enlarged heart), and demonstrate automatically-learned confounders that arise from factors beyond physiological mechanisms (e.g., chest X-ray underexposure is correlated with the classifier predicting abnormality, and eye makeup is correlated with the classifier predicting low hemoglobin levels). We further show that our method reveals a number of physiologically plausible novel attributes for future investigation (e.g., differences in the fundus associated with self-reported sex, which were previously unknown). While our approach is not able to discern causal pathways, the ability to generate hypotheses from the attribute visualizations has the potential to enable researchers to better understand, improve their assessment, and extract new knowledge from AI-based models. Importantly, we highlight that attributes generated by our framework can capture phenomena beyond physiology or pathophysiology, reflecting the real world nature of healthcare delivery and socio-cultural factors, and hence multidisciplinary perspectives are critical in these investigations. Finally, we release code to enable researchers to train their own StylEx models and analyze their predictive tasks of interest, and use the methodology presented in this paper for responsible interpretation of the revealed attributes.
View details
Shared computational principles for language processing in humans and deep language models
Ariel Goldstein
Zaid Zada
Eliav Buchnik
Amy Price
Bobbi Aubrey
Samuel A. Nastase
Harshvardhan Gazula
Gina Choe
Aditi Rao
Catherine Kim
Colton Casto
Lora Fanda
Werner Doyle
Daniel Friedman
Patricia Dugan
Lucia Melloni
Roi Reichart
Sasha Devore
Adeen Flinker
Liat Hasenfratz
Omer Levy,
Kenneth A. Norman
Orrin Devinsky
Uri Hasson
Nature Neuroscience(2022)
Preview abstract
Departing from traditional linguistic models, advances in deep learning have resulted in a new type of predictive (autoregressive) deep language models (DLMs). Using a self-supervised next-word prediction task, these models generate appropriate linguistic responses in a given context. In the current study, nine participants listened to a 30-min podcast while their brain responses were recorded using electrocorticography (ECoG). We provide empirical evidence that the human brain and autoregressive DLMs share three fundamental computational principles as they process the same natural narrative: (1) both are engaged in continuous next-word prediction before word onset; (2) both match their pre-onset predictions to the incoming word to calculate post-onset surprise; (3) both rely on contextual embeddings to represent words in natural contexts. Together, our findings suggest that autoregressive DLMs provide a new and biologically feasible computational framework for studying the neural basis of language.
View details