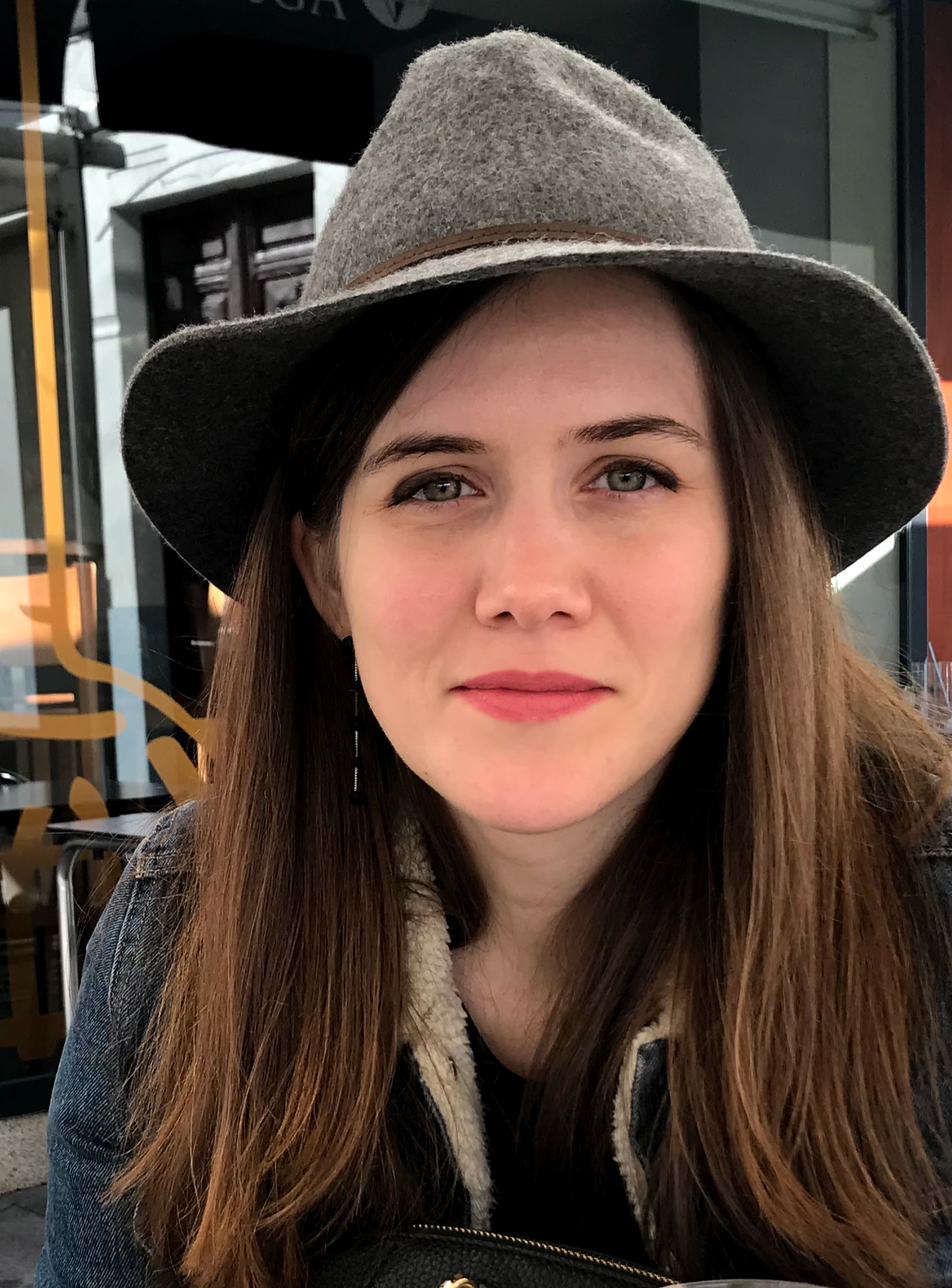
Sabela Ramos
Sabela has a PhD in High Performance Computing by the University of A Coruña (Spain) and decided to join Google while doing a postdoc at ETH (Switzerland). After spending 3 years at YouTube working on tools for developers, she moved to Google Research where she works on tools for Reinforcement Learning.
Research Areas
Authored Publications
Sort By
Factually Consistent Summarization via Reinforcement Learning with Textual Entailment Feedback
Paul Roit
Johan Ferret
Geoffrey Cideron
Matthieu Geist
Sertan Girgin
Léonard Hussenot
Nikola Momchev
Piotr Stanczyk
Nino Vieillard
Olivier Pietquin
Proceedings of the 61st Annual Meeting of the Association for Computational Linguistics, Association for Computational Linguistics(2023), 6252–6272
Preview abstract
Despite the seeming success of contemporary grounded text generation systems, they often tend to generate factually inconsistent text with respect to their input. This phenomenon is emphasized in tasks like summarization, in which the generated summaries should be corroborated by their source article. In this work we leverage recent progress on textual entailment models to directly address this problem for abstractive summarization systems. We use reinforcement learning with reference-free, textual-entailment rewards to optimize for factual consistency and explore the ensuing trade-offs, as improved consistency may come at the cost of less informative or more extractive summaries. Our results, according to both automatic metrics and human evaluation, show that our method considerably improves the faithfulness, salience and conciseness of the generated summaries.
View details
Hyperparameter Selection for Imitation Learning
Léonard Hussenot
Marcin Andrychowicz
Damien Vincent
Lukasz Piotr Stafiniak
Sertan Girgin
Nikola M Momchev
Manu Orsini
Matthieu Geist
Olivier Pietquin
ICML(2021)
Preview abstract
We address the issue of tuning hyperparameters (HPs) for imitation learning algorithms when the underlying reward function of the demonstrating expert cannot be observed at any time. The vast literature in imitation learning mostly considers this reward function to be available for HP selection, although this is not a realistic setting. Indeed, would this reward function be available, it should then directly be used for policy training and imitation would not make sense. To tackle this mostly ignored problem, we propose and study, for different representative agents and benchmarks, a number of possible proxies to the return, within an extensive empirical study. We observe that, depending on the algorithm and the environment, some methods allow good performance to be achieved without using the unknown return.
View details