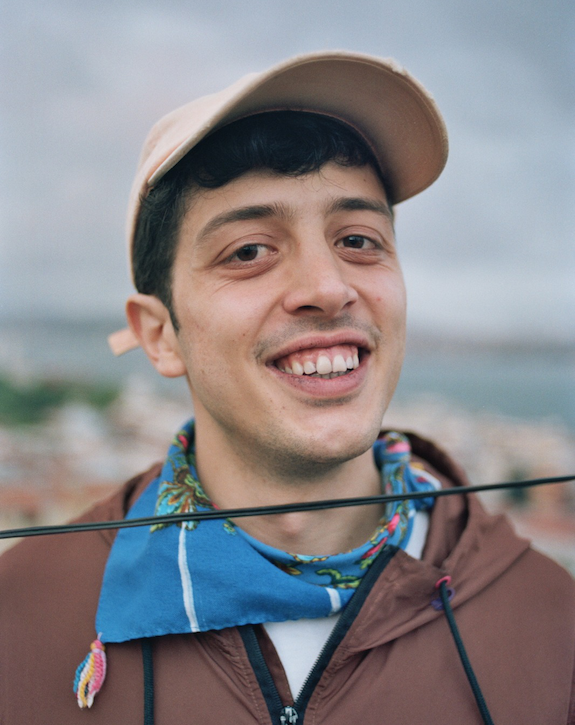
Utku Evci
Utku joined Google through the AI Residency Program in the summer of 2018 after completing his M.Sc. degree in Computer Science at NYU Courant with the Fulbright Scholarship. During his time at NYU, he worked with Levent Sagun on understanding the energy landscape of neural networks. Following his interest in network pruning, Utku wrote his M.Sc. thesis on detecting dead units in neural networks (advised by Prof. Leon Bottou). Prior the M.Sc. degree, Utku completed his undergrad degree in Koc University, Istanbul double majoring in Electrical Engineering and Computer Engineering. His prior research experience includes two summer internships at EPFL(2015), Switzerland and University of Amsterdam(2016), Netherlands.
Utku is very excited about doing research in Google. He is excited to leverage Google's computational resources to conduct research on a large scale. He believes neural network training is far from optimal in terms of speed and resources used. He is excited to work on making them faster, smaller, and more agile on learning various tasks.
His off-research interests include running, yoga, climbing and some maker projects.
His personal page and blog posts related to ML and engineering can be found here.
Research Areas
Authored Publications
Sort By
Scaling Vision Transformers to 22 Billion Parameters
Avital Oliver
Gamaleldin Elsayed
Yi Tay
Vighnesh Birodkar
Carlos Riquelme
Alexander Kolesnikov
Matthias Minderer
Lucas Beyer
Piotr Padlewski
Xiaohua Zhai
Michael Tschannen
Neil Houlsby
Mark Collier
Mathilde Caron
Josip Djolonga
Basil Mustafa
Thomas Kipf
Anurag Arnab
Filip Pavetić
Fantine Huot
Justin Gilmer
Rodolphe Jenatton
Fisher Yu
Arxiv (2023)
Preview abstract
The scaling of Transformers has driven breakthrough capabilities for language models.
At present, the largest large language models (LLMs) contain upwards of 100B parameters.
Vision Transformers (ViT) have introduced the same architecture to image and video modeling, but these have not yet been successfully scaled to nearly the same degree; the largest dense ViT contains 4B parameters. We present a recipe for highly efficient training of a 22B-parameter ViT and perform a wide variety of experiments on the resulting model. When evaluated on downstream tasks (often with a lightweight linear model on frozen features) ViT22B demonstrates increasing performance with scale. We further observe other interesting benefits of scale, including an improved tradeoff between bias and performance, an improved alignment to human visual perception in terms of shape/texture bias, and improved robustness. ViT22B demonstrates the potential for "LLM-like'' scaling in vision, and provides key steps towards getting there.
View details
Gradient Flow in Sparse Neural Networks and How Lottery Tickets Win
Cem Keskin
Yani Ioannou
AAAI Conference on Artificial Intelligence (2022)
Preview abstract
Sparse Neural Networks (NNs) can match the generalization of dense NNs using a fraction of the compute/storage for inference, and also have the potential to enable efficient training. However, naively training unstructured sparse NNs from random initialization results in significantly worse generalization, with the notable exception of Lottery Tickets (LTs) and Dynamic Sparse Training (DST). In this work, we attempt to answer: (1) why training unstructured sparse networks from random initialization performs poorly and; (2) what makes LTs and DST the exceptions? We show that sparse NNs have poor gradient flow at initialization and propose a modified initialization for unstructured connectivity. Furthermore, we find that DST methods significantly improve gradient flow during training over traditional sparse training methods. Finally, we show that LTs do not improve gradient flow, rather their success lies in re-learning the pruning solution they are derived from - however, this comes at the cost of learning novel solutions.
View details
The State of Sparse Training in Deep Reinforcement Learning
Erich Elsen
Proceedings of the 39th International Conference on Machine Learning, PMLR (2022)
Preview abstract
The use of sparse neural networks has seen a rapid growth in recent years, particularly in computer vision; their appeal stems largely due to the reduced number of parameters required to train and store, as well as in an increase in learning efficiency. Somewhat surprisingly, there have been very few efforts exploring their use in deep reinforcement learning (DRL). In this work we perform a systematic investigation into applying a number of existing sparse training techniques on a variety of DRL agents and environments. Our results highlight the overall challenge that reinforcement learning poses for sparse training methods, complemented by detailed analyses on how the various components in DRL are affected by the use of sparse networks. We conclude by suggesting some promising avenues for improving the effectiveness of general sparse training methods, as well as for advancing their use in DRL.
View details
Training Recipe for N:M Structured Sparsity with Decaying Pruning Mask
Sheng-Chun Kao
Tushar Krishna
Shivani Agrawal
Suvinay Subramanian
(2022) (to appear)
Preview abstract
Sparsity has become one of the promising methods to compress and accelerate Deep Neural Networks (DNNs). Among different categories of sparsity, structured sparsity has gained more attentions due to its efficient execution on modern accelerators. Particularly, N:M sparsity is attractive because there are already hardware accelerator architectures that can leverage few forms of N:M structured sparsity in the model to yield higher compute-efficiency. While there is a large body of work proposing various recipes for N:M structured sparsity training, compute-efficient training recipes for structured sparsity is rather a less explored territory. In this
work, we focus on N:M sparsity and extensively study and evaluate various training recipes for N:M sparsity in terms of the trade-off between model accuracy and compute training cost (FLOPs). Building upon this study, we propose two new decay-based pruning methods, namely “pruning mask decay” and “sparse structure decay”. Our evaluations indicate that these proposed methods consistently deliver SOTA model accuracy, comparable to unstructured sparsity, on a transformer-based model for translate task. The increase in the accuracy of the sparse model using the new training recipes comes at the cost of marginal increase in the total training
compute (FLOPs).
View details
Head2Toe: Utilizing Intermediate Representations for Better Transfer Learning
Mike Mozer
Proceedings of the 39th International Conference on Machine Learning, PMLR (2022)
Preview abstract
Transfer-learning methods aim to improve performance in a data-scarce target domain using a model pretrained on a source domain. A cost-efficient strategy, , involves freezing the source model and training a new classification head for the target domain. This strategy is outperformed by a more costly but state-of-the-art method--- all parameters of the source model to the target domain---possibly because fine tuning allows the model to leverage useful information from intermediate layers which is otherwise discarded. We explore the hypothesis that these intermediate layers might be directly exploited by linear probing. We propose a method, , that selects features from all layers of the source model to train a target-domain classification head. In evaluations on the Visual Task Adaptation Benchmark, Head2Toe matches performance obtained with fine tuning on average, but critically, for out-of-distribution transfer, Head2Toe outperforms fine tuning.
View details
GradMax: Growing Neural Networks using Gradient Information
Thomas Unterthiner
Bart van Merriënboer
The International Conference on Learning Representations (2022)
Preview abstract
The architecture and the parameters of neural networks are often optimized independently, which requires costly retraining of the parameters whenever the architecture is modified. In this work we instead focus on growing the architecture without requiring costly retraining. We present a method that adds new neurons during training without impacting what is already learned, while improving the training dynamics. We achieve the latter by maximizing the gradients of the new weights and find the optimal initialization efficiently by means of the singular value decomposition (SVD). We call this technique Gradient Maximizing Growth (GradMax) and demonstrate its effectiveness in variety of vision tasks and architectures.
View details
A Unified Few-Shot Classification Benchmark to Compare Transfer and Meta Learning Approaches
Sylvain Gelly
Xiaohua Zhai
Neil Houlsby
NeurIPS Datasets and Benchmarks Track (2021)
Preview abstract
Meta and transfer learning are two successful families of approaches to few-shot learning. Despite highly related goals, state-of-the-art advances in each family are measured largely in isolation of each other. As a result of diverging evaluation norms, a direct or thorough comparison of different approaches is challenging. To bridge this gap, we introduce a few-shot classification evaluation protocol named VTAB+MD with the explicit goal of facilitating sharing of insights from each community. We demonstrate its accessibility in practice by performing a cross-family study of the best transfer and meta learners which report on both a large-scale meta-learning benchmark (Meta-Dataset, MD), and a transfer learning benchmark (Visual Task Adaptation Benchmark, VTAB). We find that, on average, large-scale transfer methods (Big Transfer, BiT) outperform competing approaches on MD, even when trained only on ImageNet. In contrast, meta-learning approaches struggle to compete on VTAB when trained and validated on MD. However, BiT is not without limitations, and pushing for scale does not improve performance on highly out-of-distribution MD tasks. We hope that this work contributes to accelerating progress on few-shot learning research.
View details
Meta-Dataset: A Dataset of Datasets for Learning to Learn from Few Examples
Eleni Triantafillou
Tyler Zhu
Carles Gelada
Kelvin Xu
International Conference on Learning Representations (submission) (2020)
Preview abstract
Few-shot classification refers to learning a classifier for new classes given only a few examples. While a plethora of models have emerged to tackle this recently, we find the current procedure and datasets that are used to systematically assess progress in this setting lacking. To address this, we propose META-DATASET: a new benchmark for training and evaluating few-shot classifiers that is large-scale, consists of multiple datasets, and presents more natural and realistic tasks. The aim is to measure the ability of state-of the-art models to leverage diverse sources of data to achieve higher generalization, and to evaluate that generalization ability in a more challenging setting. We additionally measure robustness of current methods to variations in the number of available examples and the number of classes. Finally our extensive empirical evaluation leads us to identify weaknesses in Prototypical Networks and MAML, two popular few-shot classification methods, and to propose a new method, ProtoMAML, which achieves improved performance on our benchmark.
View details
Rigging The Lottery: Making All Tickets Winners
Erich Elsen
Jacob Menick
International Conference of Machine Learning (2020)
Preview abstract
Recent work (Kalchbrenner et al., 2018) has demonstrated that sparsity in theparameters of neural networks leads to more parameter and floating-point oper-ation (flop) efficient networks and that these gains also translate into inferencetime reductions. There is a large body of work (Molchanov et al., 2017; Zhu &Gupta, 2017; Louizos et al., 2017; Li et al., 2016; Guo et al., 2016) on variousways ofpruningnetworks that require dense training but yield sparse networksfor inference. This limits the size of the largest trainable model to the largesttrainable dense model. Concurrently, other work (Mocanu et al., 2018; Mostafa& Wang, 2019; Bellec et al., 2017), have introduced dynamic sparse reparameter-ization training methods that allow a network to be trained while always sparse.However, they either do not reach the accuracy of pruning, or do not have a fixedFLOP cost due to parameter re-allocation during training. This work introducesa new method that does not require parameter re-allocation for end-to-end sparsetraining that matches and even exceeds the accuracy of dense-to-sparse methods.We show that this method requires less FLOPs to achieve a given level of accu-racy than previous methods. We also provide some insights into why static sparsetraining fails to find good minima and dynamic reparameterization succeeds.
View details