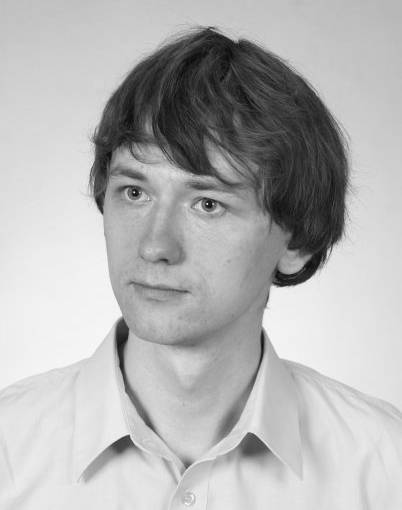
Michał Januszewski
Authored Publications
Sort By
Google
Multi-Layered Maps of Neuropil with Segmentation Guided Contrastive Learning
Sven Dorkenwald
Daniel R. Berger
Agnes L. Bodor
Forrest Collman
Casey M. Schneider-Mizell
Nuno Maçarico da Costa
Jeff W. Lichtman
Nature Methods (2023)
Automated synapse-level reconstruction of neural circuits in the larval zebrafish brain
Fabian Svara
Dominique Förster
Fumi Kubo
Marco dal Maschio
Philipp Schubert
Jörgen Kornfeld
Adrian Wanner
Winfried Denk
Herwig Baier
Nature Methods, 19 (2022), 1357–1366
Visual recognition of social signals by a tectothalamic neural circuit
Johannes M. Kappel
Dominique Förster
Katja Slangewal
Inbal Shainer
Fabian Svara
Joseph C. Donovan
Shachar Sherman
Herwig Baier
Johannes Larsch
Nature, 608 (2022), 146–152
SyConn2: dense synaptic connectivity inference for volume electron microscopy
Philipp J. Schubert
Sven Dorkenwald
Jonathan Klimesch
Fabian Svara
Andrei Mancu
Hashir Ahmad
Michale S. Fee
Joergen Kornfeld
Nature Methods, 19 (2022), 1367–1370
Denoising-based Image Compression for Connectomics
Alex Shapson-Coe
Richard L. Schalek
Johannes Ballé
Jeff W. Lichtman
bioRxiv (2021)
A connectomic study of a petascale fragment of human cerebral cortex
Alex Shapson-Coe
Daniel R. Berger
Yuelong Wu
Richard L. Schalek
Shuohong Wang
Neha Karlupia
Sven Dorkenwald
Evelina Sjostedt
Dongil Lee
Luke Bailey
Angerica Fitzmaurice
Rohin Kar
Benjamin Field
Hank Wu
Julian Wagner-Carena
David Aley
Joanna Lau
Zudi Lin
Donglai Wei
Hanspeter Pfister
Adi Peleg
Jeff W. Lichtman
bioRxiv (2021)
A connectome and analysis of the adult Drosophila central brain
Louis K Scheffer
C Shan Xu
Zhiyuan Lu
Shin-ya Takemura
Kenneth J Hayworth
Gary B Huang
Kazunori Shinomiya
Stuart Berg
Jody Clements
Philip M Hubbard
William T Katz
Lowell Umayam
Ting Zhao
David Ackerman
John Bogovic
Tom Dolafi
Dagmar Kainmueller
Takashi Kawase
Khaled A Khairy
Larry Lindsey
Nicole Neubarth
Donald J Olbris
Hideo Otsuna
Eric T Trautman
Masayoshi Ito
Alexander S Bates
Jens Goldammer
Tanya Wolff
Robert Svirskas
Philipp Schlegel
Erika Neace
Christopher J Knecht
Chelsea X Alvarado
Dennis A Bailey
Samantha Ballinger
Jolanta A Borycz
Brandon S Canino
Natasha Cheatham
Michael Cook
Marisa Dreher
Octave Duclos
Bryon Eubanks
Kelli Fairbanks
Samantha Finley
Nora Forknall
Audrey Francis
Gary Patrick Hopkins
Emily M Joyce
SungJin Kim
Nicole A Kirk
Julie Kovalyak
Shirley Lauchie
Alanna Lohff
Charli Maldonado
Emily A Manley
Sari McLin
Caroline Mooney
Miatta Ndama
Omotara Ogundeyi
Nneoma Okeoma
Christopher Ordish
Nicholas Padilla
Christopher M Patrick
Tyler Paterson
Elliott E Phillips
Emily M Phillips
Neha Rampally
Caitlin Ribeiro
Madelaine K Robertson
Jon Thomson Rymer
Sean M Ryan
Megan Sammons
Anne K Scott
Ashley L Scott
Aya Shinomiya
Claire Smith
Kelsey Smith
Natalie L Smith
Margaret A Sobeski
Alia Suleiman
Jackie Swift
Satoko Takemura
Iris Talebi
Dorota Tarnogorska
Emily Tenshaw
Temour Tokhi
John J Walsh
Tansy Yang
Jane Anne Horne
Feng Li
Ruchi Parekh
Patricia K Rivlin
Vivek Jayaraman
Marta Costa
Gregory SXE Jefferis
Kei Ito
Stephan Saalfeld
Reed George
Ian Meinertzhagen
Gerald M Rubin
Harald F Hess
Stephen M Plaza
eLife, 9 (2020)
An anatomical substrate of credit assignment in reinforcement learning
Jorgen Kornfeld
Michale S. Fee
Philipp Schubert
Winfried Denk
bioRxiv (2020)
Automated Reconstruction of a Serial-Section EM Drosophila Brain with Flood-Filling Networks and Local Realignment
Larry Lindsey
Zhihao Zheng
Alexander Shakeel Bates
István Taisz
Matthew Nichols
Feng Li
Eric Perlman
Gregory S.X.E. Jefferis
Davi Bock
bioRxiv (2019)