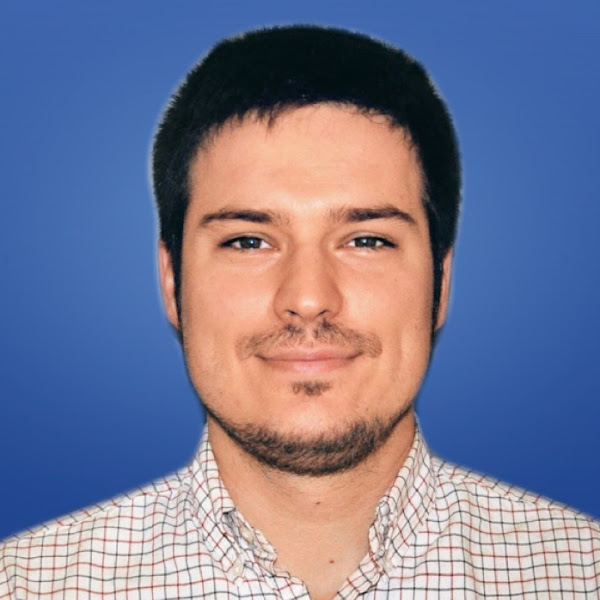
Mario Lucic
Mario Lucic is a Senior Staff Research Scientist at Google DeepMind working on the Gemini team where he is co-leading the efforts on video and audio-video understanding. Since joining Google he worked on GenAI and large-scale vision and multimodal systems. He received his PhD from ETH Zurich where he investigated efficient and theoretically grounded data selection algorithms. He is serving as the Area Chair for NeurIPS and ICLR conferences.
Research Areas
Authored Publications
Sort By
Google
PaLI-X: On Scaling up a Multilingual Vision and Language Model
Josip Djolonga
Piotr Padlewski
Basil Mustafa
Carlos Riquelme
Sebastian Goodman
Yi Tay
Siamak Shakeri
Daniel Salz
Michael Tschannen
Hexiang (Frank) Hu
Mandar Joshi
Matthias Minderer
Filip Pavetić
Gang Li
Lucas Beyer
Anurag Arnab
Yuanzhong Xu
Keran Rong
Alexander Kolesnikov
Xiaohua Zhai
Neil Houlsby
Computer Vision and Pattern Recognition Conference (CVPR) (2024)
Scaling Vision Transformers to 22 Billion Parameters
Josip Djolonga
Basil Mustafa
Piotr Padlewski
Justin Gilmer
Mathilde Caron
Rodolphe Jenatton
Lucas Beyer
Michael Tschannen
Anurag Arnab
Carlos Riquelme
Matthias Minderer
Gamaleldin Elsayed
Fisher Yu
Avital Oliver
Fantine Huot
Mark Collier
Vighnesh Birodkar
Yi Tay
Alexander Kolesnikov
Filip Pavetić
Thomas Kipf
Xiaohua Zhai
Neil Houlsby
Arxiv (2023)
RUST: Latent Neural Scene Representations from Unposed Imagery
Thomas Kipf
Klaus Greff
Conference on Computer Vision and Pattern Recognition (CVPR) (2023) (to appear)
VCT: A Video Compression Transformer
Sung Jin Hwang
NeurIPS 2022, NeurIPS 2022
Which Model to Transfer? Finding the Needle in the Growing Haystack
Cedric Renggli
André Susano Pinto
Luka Rimanic
Carlos Riquelme
Ce Zhang
Conference on Computer Vision and Pattern Recognition (2022) (to appear)
Scene Representation Transformer: Geometry-Free Novel View Synthesis Through Set-Latent Scene Representations
Henning Meyer
Urs Bergmann
Klaus Greff
Noha Radwan
Alexey Dosovitskiy
Jakob Uszkoreit
Conference on Computer Vision and Pattern Recognition (CVPR) (2022)
Object Scene Representation Transformer
Filip Pavetić
Leonidas Guibas
Klaus Greff
Thomas Kipf
Advances in Neural Information Processing Systems (2022), pp. 9512-9524
MLP-Mixer: An All-MLP Architecture for Vision
Neil Houlsby
Alexander Kolesnikov
Lucas Beyer
Xiaohua Zhai
Thomas Unterthiner
Jessica Yung
Jakob Uszkoreit
Alexey Dosovitskiy
NeurIPS 2021 (poster)
Revisiting the Calibration of Modern Neural Networks
Matthias Minderer
Josip Djolonga
Rob Romijnders
Frances Ann Hubis
Xiaohua Zhai
Neil Houlsby
Neural Information Processing Systems (2021) (to appear)