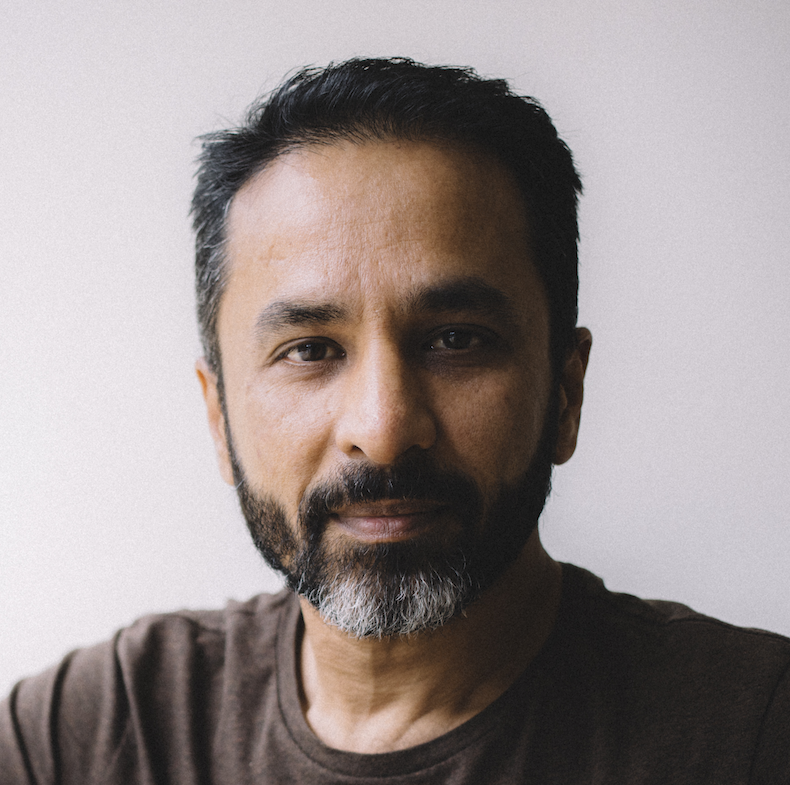
Izhak Shafran
Izhak Shafran is a researcher working on deep learning for speech and language processing.
Before joining Google, he was a faculty member at the Oregon Health & Science University (OHSU) and the Johns Hopkins University (JHU).
Before joining Google, he was a faculty member at the Oregon Health & Science University (OHSU) and the Johns Hopkins University (JHU).
Authored Publications
Sort By
Description-Driven Task-Oriented Dialog Modeling
Dian Yu
Mingqiu Wang
Preview abstract
Task-oriented dialogue (TOD) systems are required to identify key information from conversations for the completion of given tasks. Such information is conventionally specified in terms of intents and slots contained in task-specific ontology or schemata. Since these schemata are designed by system developers, the naming convention for slots and intents is not uniform across tasks, and may not convey their semantics effectively. This can lead to models memorizing arbitrary patterns in data, resulting in suboptimal performance and generalization. In this paper, we propose that schemata should be modified by replacing names or notations entirely with natural language descriptions. We show that a language description-driven system exhibits better understanding of task specifications, higher performance on state tracking, improved data efficiency, and effective zero-shot transfer to unseen tasks. Following this paradigm, we present a simple yet effective Description-Driven Dialog State Tracking (D3ST) model, which relies purely on schema descriptions and an "index-picking" mechanism. We demonstrate the superiority in quality, data efficiency and robustness of our approach as measured on the MultiWOZ (Budzianowski et al.,2018), SGD (Rastogi et al., 2020), and the recent SGD-X (Lee et al., 2021) benchmarks.
View details
Google COVID-19 Search Trends Symptoms Dataset: Anonymization Process Description
Akim Kumok
Chaitanya Kamath
Charlotte Stanton
Damien Desfontaines
Evgeniy Gabrilovich
Gerardo Flores
Gregory Alexander Wellenius
Ilya Eckstein
John S. Davis
Katie Everett
Krishna Kumar Gadepalli
Rayman Huang
Shailesh Bavadekar
Thomas Ludwig Roessler
Venky Ramachandran
Yael Mayer
Arxiv.org, N/A (2020)
Preview abstract
This report describes the aggregation and anonymization process applied to the initial version of COVID-19 Search Trends symptoms dataset, a publicly available dataset that shows aggregated, anonymized trends in Google searches for symptoms (and some related topics). The anonymization process is designed to protect the daily search activity of every user with \varepsilon-differential privacy for \varepsilon = 1.68.
View details
The Medical Scribe: Corpus Development and Model Performance Analyses
Amanda Perry
Ashley Robson Domin
Chris Co
Gang Li
Hagen Soltau
Justin Stuart Paul
Lauren Keyes
Linh Tran
Mark David Knichel
Mingqiu Wang
Nan Du
Rayman Huang
Proc. Language Resources and Evaluation, 2020
Preview abstract
There has been a growing interest in creating tools to assist clinical note generation from the audio of provider-patient encounters. Motivated by this goal and with the help of providers and experienced medical scribes, we developed an annotation scheme to extract relevant clinical concepts. Using this annotation scheme, a corpus of about 6k clinical encounters was labeled, which was used to train a state-of-the-art tagging model. We report model performance and a detailed analyses of the results.
View details
Audio De-identification: A New Entity Recognition Task
Ido Cohn
Gang Li
Tzvika Hartman
NAACL (2019)
Preview abstract
Named Entity Recognition (NER) has been mostly studied in the context of written text. Specifically, NER is an important step in de-identification (de-ID) of medical records, many of which are recorded conversations between a patient and a doctor. In such recordings, audio spans with personal information should be redacted, similar to the redaction of sensitive character spans in de-ID for written text. The application of NER in the context of audio de-identification has yet to be fully investigated. To this end, we define the task of audio de-ID, in which audio spans with entity mentions should be detected. We then present our pipeline for this task, which involves Automatic Speech Recognition (ASR), NER on the transcript text, and text-to-audio alignment. Finally, we introduce a novel metric for audio de-ID and a new evaluation benchmark consisting of a large labeled segment of the Switchboard and Fisher audio datasets and detail our pipeline's results on it.
View details
Preview abstract
Speech applications dealing with conversations require not only recognizing the spoken words, but also determining who spoke when. The task of assigning words to speakers is typically addressed by merging the outputs of two separate systems, namely, an automatic speech recognition (ASR) system and a speaker diarization (SD) system. The two systems are trained independently with different objective functions. Often the SD systems operate directly on the acoustics and are not constrained to respect word boundaries and this deficiency is overcome in an ad hoc manner. Motivated by recent advances in sequence to sequence learning, we propose a novel approach to tackle the two tasks by a joint ASR and SD system using a recurrent neural network transducer. Our approach utilizes both linguistic and acoustic cues to infer speaker roles, as opposed to typical SD subsystems, which only use acoustic cues. We evaluate the performance of our model on a large corpus of medical conversations between physicians and patients and find that our approach improves performance by about 86% word-level diarization error rate over a competitive conventional baseline.
View details
Preview abstract
Motivated by the need to solve a real-world application, we propose a novel model for extracting relationships in tasks where the label space is large but can be factored and the training data is limited. The model tackles the problem in multiple stages but is trained end-to-end using curriculum learning. Each stage realizes simple intuitions for improving the model and through ablation analysis we see the benefits of each stage. We evaluate our models on two tasks, that of extracting symptoms and medications along with their properties from clinical conversations. While LSTM-based baselines achieve a F1-score of 0.08 and 0.35 for symptoms and medications respectively, our models achieve a performance of 0.56 and 0.43 respectively.
View details
Extracting Symptoms and their Status from Clinical Conversations
Nan Du
Linh Tran
Proceedings of the 57th Annual Meeting of the Association for Computational Linguistics, Florence, Italy (2019), pp. 915-9125
Preview abstract
This paper describes novel models tailored for a new application, that of extracting the symptoms mentioned in clinical conversations along with their status. Lack of any publicly available corpus in this privacy-sensitive domain led us to develop our own corpus, consisting of about 3K conversations annotated by professional medical scribes. We propose two novel deep learning approaches to infer the symptom names and their status: (1) a new hierarchical span-attribute tagging (SAT) model, trained using curriculum learning, and (2) a variant of sequence-to-sequence model which decodes the symptoms and their status from a few speaker turns within a sliding window over the conversation. This task stems from a realistic application of assisting medical providers in capturing symptoms mentioned by patients from their clinical conversations. To reflect this application, we define multiple metrics. From inter-rater agreement, we find that the task is inherently difficult. We conduct comprehensive evaluations on several contrasting conditions and observe that the performance of the models range from an F-score of 0.5 to 0.8 depending on the condition. Our analysis not only reveals the inherent challenges of the task, but also provides useful directions to improve the models.
View details
Preview abstract
Unitary Evolution Recurrent Neural Networks (uRNNs) have three attractive properties: (a) the unitary property, (b) the complex-valued nature, and (c) their efficient linear operators [1]. The literature so far does not address - how critical is the unitary property of the model? Furthermore, uRNNs have not been evaluated on large tasks. To study these shortcomings, we propose the complex evolution Recurrent Neural Networks (ceRNNs), which is similar to uRNNs but drops the unitary property selectively. On a simple multivariate linear regression task, we illustrate that dropping the constraints improves the learning trajectory. In copy memory task, ceRNNs and uRNNs perform identically, demonstrating that their superior performance over LSTMs is due to complex-valued nature and their linear operators. In a large scale real-world speech recognition, we find that pre-pending a uRNN degrades the performance of our baseline LSTM acoustic models, while pre-pending a ceRNN improves the performance over the baseline by 0.8% absolute WER.
View details
Multichannel Signal Processing with Deep Neural Networks for Automatic Speech Recognition
Kean Chin
Chanwoo Kim
IEEE /ACM Transactions on Audio, Speech, and Language Processing, 25 (2017), pp. 965 - 979
Preview abstract
Multichannel ASR systems commonly separate speech enhancement, including localization, beamforming and postfiltering, from acoustic modeling. In this paper, we perform multichannel enhancement jointly with acoustic modeling in a deep neural network framework. Inspired by beamforming, which leverages differences in the fine time structure of the signal at different microphones to filter energy arriving from different directions, we explore modeling the raw time-domain waveform directly. We introduce a neural network architecture which performs multichannel filtering in the first layer of the network and show that this network learns to be robust to varying target speaker direction of arrival, performing as well as a model that is given oracle knowledge of the true target speaker direction.
%
Next, we show how performance can be improved by \emph{factoring} the first layer to separate the multichannel spatial filtering operation from a single channel filterbank which computes a frequency decomposition.
%
We also introduce an adaptive variant, which updates the spatial filter coefficients at each time frame based on the previous inputs.
%
Finally we demonstrate that these approaches can be implemented more efficiently in the frequency domain. Overall, we find that such multichannel neural networks give a relative word error rate improvement of more than 5\% compared to a traditional beamforming-based multichannel ASR system and more than 10\% compared to a single channel waveform model.
View details
Raw Multichannel Processing Using Deep Neural Networks
Kean Chin
Chanwoo Kim
New Era for Robust Speech Recognition: Exploiting Deep Learning, Springer (2017)
Preview abstract
Multichannel ASR systems commonly separate speech enhancement, including localization, beamforming and postfiltering, from acoustic modeling. In this chapter, we perform multi-channel enhancement jointly with acoustic modeling in a deep neural network framework. Inspired by beamforming, which leverages differences in the fine time structure of the signal at different microphones to filter energy arriving from different directions, we explore modeling the raw time-domain waveform directly. We introduce a neural network architecture which performs multichannel filtering in the first layer of the network and show that this network learns to be robust to varying target speaker direction of arrival, performing as well as a model that is given oracle knowledge of the true target speaker direction. Next, we show how performance can be improved by factoring the first layer to separate the multichannel spatial filtering operation from a single channel filterbank which computes a frequency decomposition. We also introduce an adaptive variant, which updates the spatial filter coefficients at each time frame based on the previous inputs. Finally we demonstrate that these approaches can be implemented more efficiently in the frequency domain. Overall, we find that such multichannel neural networks give a relative word error rate improvement of more than 5% compared to a traditional beamforming-based multichannel ASR system and more than 10% compared to a single channel waveform model.
View details