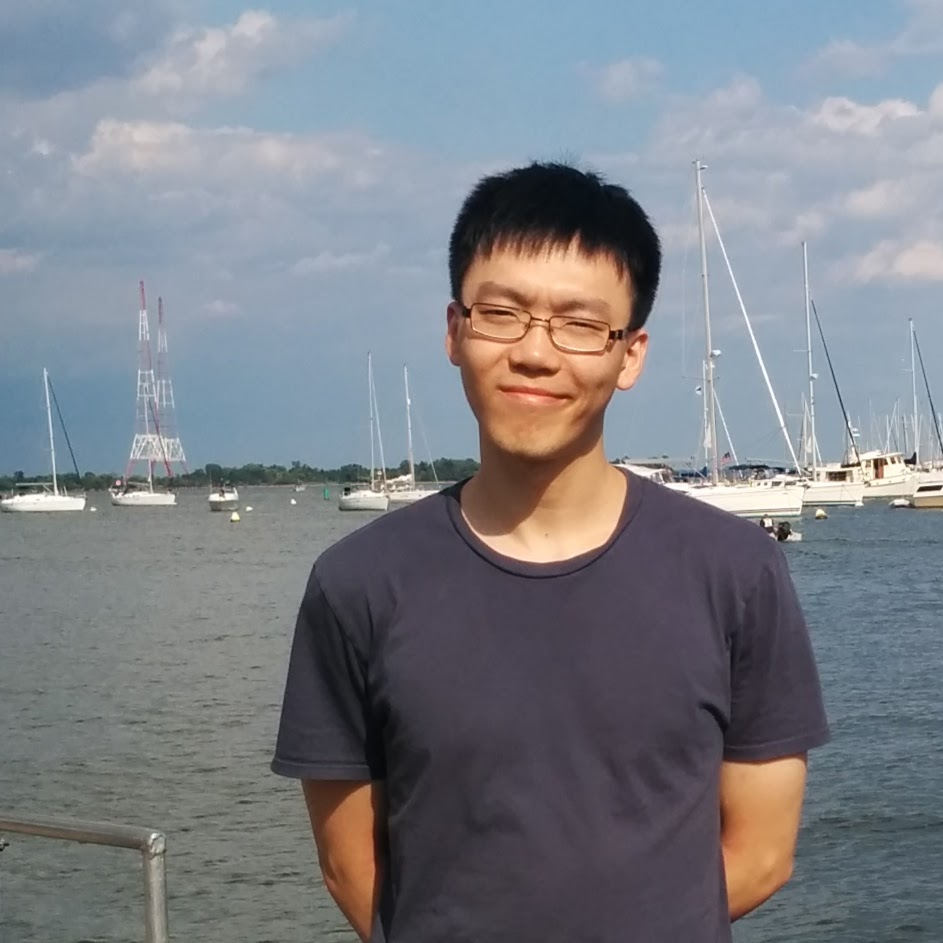
Di Wang
Di Wang is in the Market Algorithm team, which is part of the broader Algorithms, Theory, Neural networks, and AI (Athena) team under Google Research. Di finished his PhD in Computer Science at UC Berkeley where he was advised by Satish Rao. Before joining Google, Di worked as a postdoc researcher at the Simons Institute (UC Berkeley) and the Algorithms & Randomness Center at Georgia Tech. Di's research interests are in the design of efficient algorithms, especially for large-scale optimization problems and graph problems that arise broadly in applications from machine learning, data analysis and operations research. Di's work draws on a broad range of numerical and discrete tools from combinatorics, optimization and graph theory, which leads to not only stronger theoretical guarantees, but also practical improvements for computational challenges arising in practice.
Authored Publications
Sort By
Auto-bidding and Auctions in Online Advertising: A Survey
Ashwinkumar Badanidiyuru Varadaraja
Christopher Liaw
Haihao (Sean) Lu
Andres Perlroth
Georgios Piliouras
Ariel Schvartzman
Kelly Spendlove
Hanrui Zhang
Mingfei Zhao
ACM SIGecom Exchanges, 22 (2024)
Preview abstract
In this survey, we summarize recent developments in research fueled by the growing adoption of automated bidding strategies in online advertising. We explore the challenges and opportunities that have arisen as markets embrace this autobidding and cover a range of topics in this area, including bidding algorithms, equilibrium analysis and efficiency of common auction formats, and optimal auction design.
View details
Preview abstract
Motivated by the online advertising industry, we study the non-stationary stochastic budget management problem: An advertiser repeatedly participates in $T$ second-price auctions, where her value and the highest competing bid are drawn from unknown time-varying distributions, with the goal of maximizing her total utility subject to her budget constraint. In the absence of any information about the distributions, it is known that sub-linear regret cannot be achieved. We assume access to historical samples, with the goal of developing algorithms that are robust to discrepancies between the sampling distributions and the true distributions. We show that our Dual Follow-The-Regularized-Leader algorithm is robust and achieves a near-optimal $\tilde O(\sqrt{T})$-regret with just one sample per distribution, drastically improving over the best-known sample-complexity of $T$ samples per distribution.
View details
Preview abstract
Online advertising has recently become a highly competitive, complex, multi-billion-dollar industry with advertisers bidding for ad slots at large scales and high frequencies. This has resulted in the growing need for efficient ``auto-bidding'' algorithms to determine the bids for incoming queries to maximize advertisers' targets subject to their specified constraints. Our work focuses on designing efficient online algorithms for a single value-maximizing advertiser under a commonly seen constraint: Return-on-Spend (RoS). We quantify the efficiency in terms of \emph{regret} in comparison with the optimal algorithm that knows all the queries a priori.
Our main contribution is an algorithm that achieves near-optimal regret while respecting the specified RoS constraint. We are able to also incorporate our results with the pre-existing work of Balseiro, Lu, and Mirrokni~\cite{BLM20} to achieve near-optimal regret while respecting \emph{both} RoS and fixed budget constraints. Our main technical insight is in leveraging ideas from the literature on (offline) packing linear programs, in addition to the generic structural properties arising from our auction model.
View details
Minimum Cost Flows, MDPs, and $\ell_1$-Regression in Nearly Linear Time for Dense Instances
Jan van den Brand
Yin Tat Lee
Yang P. Liu
Thatchaphol Saranurak
Aaron Sidford
Zhao Song
The 53rd ACM Symposium on Theory of Computing (STOC) (2021) (to appear)
Preview abstract
In this paper we provide new randomized algorithms with improved runtimes for solving linear programs with two-sided constraints. In the special case of the minimum cost flow problem on $n$-vertex $m$-edge graphs with integer polynomially-bounded costs and capacities we obtain a randomized method which solves the problem in $\tilde{O}(m + n^{1.5})$ time. This improves upon the previous best runtime of $\tilde{O}(m \sqrt{n})$ \cite{ls14} and, in the special case of unit-capacity maximum flow, improves upon the previous best runtimes of $m^{4/3 + o(1)}$ \cite{ls20_focs,kathuria2020potential} and $\tilde{O}(m \sqrt{n})$ \cite{ls14} for sufficiently dense graphs.
In the case of $\ell_1$-regression in a matrix with $n$-columns and $m$-rows we obtain a randomized method which computes an $\epsilon$-approximate solution in $\tilde{O}(mn + n^{2.5})$ time. This yields a randomized method which computes an $\epsilon$-optimal policy of a discounted Markov Decision Process with $S$ states and, $A$ actions per state in time $\tilde{O}(S^2 A + S^{2.5})$. These methods improve upon the previous best runtimes of methods which depend polylogarithmically on problem parameters, which were $\tilde{O}(mn^{1.5})$ \cite{LeeS15} and $\tilde{O}(S^{2.5} A)$ \cite{ls14,SidfordWWY18} respectively.
To obtain this result we introduce two new algorithmic tools of possible independent interest. First, we design a new general interior point method for solving linear programs with two sided constraints which combines techniques from \cite{lsz19} and \cite{BrandLN+20} to obtain a robust stochastic method with iteration count nearly the square root of the smaller dimension. Second, to implement this method we provide dynamic data structures for efficiently maintaining approximations to variants of Lewis-weights, a fundamental importance measure for matrices which generalize leverage scores and effective resistances.
View details
Bipartite Matching in Nearly-linear Time on Moderately Dense Graphs
Jan van den Brand
Yin-Tat Lee
Danupon Nanongkai
Richard Peng
Thatchaphol Saranurak
Aaron Sidford
Zhao Song
61st Annual IEEE Symposium on Foundations of Computer Science (FOCS) (2020)
Preview abstract
We present an $\tilde O(m+n^{1.5})$-time randomized algorithm for maximum bipartite matching and related problems (e.g. transshipment, negative-weight shortest paths, and optimal transport) on $m$-edge and $n$-node graphs. For maximum bipartite matching on moderately dense graphs, i.e. $m = \Omega(n^{1.5})$, our algorithm runs in time nearly linear in the input size and constitutes the first improvement over the classic $O(m\sqrt{n})$-time [Dinic 1970; Hopcroft-Karp 1971; Karzanov 1973] and $\tilde O(n^\omega)$-time algorithms [Ibarra-Moran 1981] (where currently $\omega\approx 2.373$). On sparser graphs, i.e. when $m = n^{9/8 + \delta}$ for any constant $\delta>0$, our result improves upon the recent advances of [Madry 2013] and [Liu-Sidford 2020b, 2020a] which achieve an $\tilde O(m^{4/3})$ runtime.
We obtain this result by several techniques of independent interest. First, we provide a simple sublinear-time algorithm for detecting high-energy edges in electric flows on expanders. Second, we combine this technique with recent advances in dynamic expander decompositions to provide a data structure for efficiently maintaining the iterates of the interior point method of [v.d.Brand-Lee-Sidford-Song 2020]. Finally, we simplify and improve this method by providing new sampling-based techniques for handling loss of feasibility due to approximate linear system solvers. Combining these results yields our $\tilde O(m+n^{1.5})$-time algorithm.
View details
Preview abstract
We study the problem of computing a minimum cut in a simple, undirected graph and give a deterministic $O(m \log^2 n \log\log^2 n)$ time algorithm. This improves on both the best previously known deterministic running time of $O(m \log^{12} n)$ (Kawarabayashi and Thorup [J. ACM, 66 (2018), 4]) and the best previously known randomized running time of $O(m \log^{3} n)$ (Karger [J. ACM, 47 (2000), pp. 46--76]) for this problem, though Karger's algorithm can be further applied to weighted graphs. Moreover, our result extends to balanced directed graphs, where the balance of a directed graph captures how close the graph is to being Eulerian. Our approach is using the Kawarabayashi and Thorup graph compression technique, which repeatedly finds low conductance cuts. To find these cuts they use a diffusion-based local algorithm. We use instead a flow-based local algorithm and suitably adjust their framework to work with our flow-based subroutine. Both flow- and diffusion-based methods have a long history of being applied to finding low conductance cuts. Diffusion algorithms have several variants that are naturally local, while it is more complicated to make flow methods local. Some prior work has proven nice properties for local flow-based algorithms with respect to improving or cleaning up low conductance cuts. Our flow subroutine, however, is the first that both is local and produces low conductance cuts. Thus, it may be of independent interest.
View details
Packing LPs are Hard to Solve Accurately, Assuming Linear Equations are Hard
Preview
Rasmus Kyng
Peng Zhang
Proceedings of the 2020 ACM-SIAM Symposium on Discrete Algorithms (SODA) (2020)
Preview abstract
Local graph clustering and the closely related seed set expansion problem are primitives on graphs that are central to a wide range of analytic and learning tasks such as local clustering, community detection, nodes ranking and feature inference. Prior work on local graph clustering mostly falls into two categories with numerical and combinatorial roots respectively.
In this work, we draw inspiration from both fields and propose a family of convex optimization formulations based on the idea of diffusion with $p$-norm network flow for $p\in (1,\infty)$.
In the context of local clustering, we characterize the optimal solutions for these optimization problems and show their usefulness in finding low conductance cuts around input seed set. In particular, we achieve quadratic approximation of conductance in the case of $p=2$ similar to the Cheeger-type bounds of spectral methods, constant factor approximation when $p\rightarrow\infty$ similar to max-flow based methods, and a smooth transition for general $p$ values in between. Thus, our optimization formulation can be viewed as bridging the numerical and combinatorial approaches, and we can achieve the best of both worlds in terms of speed and noise robustness.
We show that the proposed problem can be solved in strongly local running time for $p\ge 2$ and conduct empirical evaluations on both synthetic and real-world graphs to illustrate our approach compares favorably with existing methods.
View details
Preview abstract
The problem of finding dense components of a graph is a major primitive in graph mining and data analysis. The densest subgraph problem (DSP) that asks to find a subgraph with maximum average degree forms a basic primitive in dense subgraph discovery with applications ranging from community detection to unsupervised discovery of biological network modules. The DSP is exactly solvable in polynomial time using maximum flows. Due to the high computational cost of maximum flows, Charikar's greedy approximation algorithm is usually preferred in practice due to its linear time and linear space complexity. It constitutes a key algorithmic idea in scalable solutions for large-scale dynamic graphs. However, its output density can be a factor 2 off the optimal solution.
In this paper we design Greedy++, an iterative peeling algorithm that improves upon the performance of Charikar's greedy algorithm significantly. Our iterative greedy algorithm is able to output near-optimal and optimal solutions fast by adding a few more passes to Charikar's greedy algorithm. Furthermore Greedy++ is more robust against the structural heterogeneities (e.g., skewed degree distributions) in real-world datasets. An additional property of our algorithm is that it is able to assess quickly, without computing maximum flows, whether Charikar's approximation quality on a given graph instance is closer to the worst case theoretical guarantee of 1/2 or to optimality. We also demonstrate that our method has significant efficiency advantage over the maximum flow based exact optimal algorithm. For example, our algorithm achieves ~145x speedup on average across a variety of real-world graphs while finding subgraphs of density that are at least 90% as dense as the densest subgraph.
View details