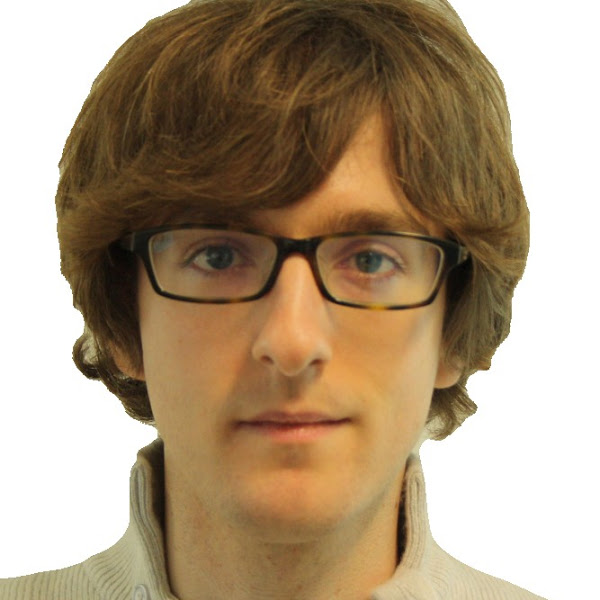
Joe Ledsam
Joe is clinician scientist in Google Japan focusing on the application of AI to health and science. Prior to joining Google Japan, Joe spent four years in DeepMind leading multiple research projects across medical imaging and electronic health records, as well as founding the DeepMind Genomics team. Joe remains an active collaborator with Google Health, DeepMind and other research groups throughout Google. He obtained his medical degree from The University of Leeds, UK, and was a research fellow at University College London during his years in clinical practice.
Research Areas
Authored Publications
Sort By
Google
Assistive AI in Lung Cancer Screening: A Retrospective Multinational Study in the United States and Japan
Atilla Kiraly
Corbin Cunningham
Ryan Najafi
Jie Yang
Chuck Lau
Diego Ardila
Scott Mayer McKinney
Rory Pilgrim
Mozziyar Etemadi
Sunny Jansen
Lily Peng
Shravya Shetty
Neeral Beladia
Krish Eswaran
Radiology: Artificial Intelligence (2024)
Artificial intelligence as a second reader for screening mammography
Etsuji Nakai
Alessandro Scoccia Pappagallo
Hiroki Kayama
Lin Yang
Shawn Xu
Christopher Kelly
Timo Kohlberger
Daniel Golden
Akib Uddin
Radiology Advances, 1(2) (2024)
Clinically Applicable Segmentation of Head and Neck Anatomy for Radiotherapy: Deep Learning Algorithm Development and Validation Study
Stanislav Nikolov
Sam Blackwell
Alexei Zverovitch
Ruheena Mendes
Michelle Livne
Jeffrey De Fauw
Yojan Patel
Clemens Meyer
Harry Askham
Bernardino Romera Paredes
Christopher Kelly
Carlton Chu
Dawn Carnell
Cheng Boon
Derek D'Souza
Syed Moinuddin
Yasmin Mcquinlan
Sarah Ireland
Kiarna Hampton
Krystle Fuller
Hugh Montgomery
Geraint Rees
Mustafa Suleyman
Trevor John Back
Cían Hughes
Olaf Ronneberger
JMIR (2021)
Google and DeepMind: Deep Learning Systems in Ophthalmology
Xinle Liu
Akinori Mitani
Terry Spitz
Derek Wu
Artificial Intelligence in Ophthalmology (2021)
Use of deep learning to develop continuous-risk models for adverse event prediction from electronic health records
Nenad Tomašev
Sebastien Baur
Anne Mottram
Xavier Glorot
Jack William Rae
Michal Zielinski
Harry Askham
Andre Saraiva
Valerio Magliulo
Clemens Meyer
Suman Venkatesh Ravuri
Ivan Protsyuk
Alistair Connell
Cían Hughes
Julien Cornebise
Hugh Montgomery
Geraint Rees
Christopher Laing
Clifton R. Baker
Thomas Osborne
Ruth Reeves
Demis Hassabis
Dominic King
Mustafa Suleyman
Trevor John Back
Christopher Nielsen
Martin Gamunu Seneviratne
Shakir Mohamad
Nature Protocols (2021)
A prospective evaluation of AI-augmented epidemiology to forecast COVID-19 in the USA and Japan
Joel Shor
Arkady Epshteyn
Ashwin Sura Ravi
Beth Luan
Chun-Liang Li
Daisuke Yoneoka
Dario Sava
Hiroaki Miyata
Hiroki Kayama
Isaac Jones
Joe Mckenna
Johan Euphrosine
Kris Popendorf
Nate Yoder
Shashank Singh
Shuhei Nomura
Thomas Tsai
npj Digital Medicine (2021)
International evaluation of an AI system for breast cancer screening
Scott Mayer McKinney
Varun Yatindra Godbole
Jonathan Godwin
Natasha Antropova
Hutan Ashrafian
Trevor John Back
Mary Chesus
Ara Darzi
Mozziyar Etemadi
Florencia Garcia-Vicente
Fiona J Gilbert
Mark D Halling-Brown
Demis Hassabis
Sunny Jansen
Christopher Kelly
Dominic King
David Melnick
Hormuz Mostofi
Lily Hao Yi Peng
Joshua Reicher
Bernardino Romera Paredes
Richard Sidebottom
Mustafa Suleyman
Kenneth C. Young
Jeffrey De Fauw
Shravya Ramesh Shetty
Nature (2020)
Predicting OCT-derived DME grades from fundus photographs using deep learning
Arunachalam Narayanaswamy
Avinash Vaidyanathan Varadarajan
Dr. Paisan Raumviboonsuk
Dr. Peranut Chotcomwongse
Jorge Cuadros
Lily Hao Yi Peng
Pearse Keane
Nature Communications (2020)
Predicting conversion to wet age-related macular degeneration using deep learning
Jason Yim
Reena Chopra
Terry Spitz
Jim Winkens
Annette Obika
Christopher Kelly
Harry Askham
Marko Lukic
Josef Huemer
Katrin Fasler
Gabriella Moraes
Clemens Meyer
Marc Wilson
Jonathan Mark Dixon
Cían Hughes
Geraint Rees
Peng Khaw
Dominic King
Demis Hassabis
Mustafa Suleyman
Trevor John Back
Pearse Keane
Jeffrey De Fauw
Nature Medicine (2020)
A clinically applicable approach to continuous prediction of future acute kidney injury
Nenad Tomašev
Xavier Glorot
Jack W Rae
Michal Zielinski
Harry Askham
Andre Saraiva
Anne Mottram
Clemens Meyer
Suman Ravuri
Ivan Protsyuk
Alistair Connell
Cían O Hughes
Julien Cornebise
Hugh Montgomery
Geraint Rees
Chris Laing
Clifton R Baker
Kelly Peterson
Ruth Reeves
Demis Hassabis
Dominic King
Mustafa Suleyman
Trevor Back
Christopher Nielson
Shakir Mohamed
Nature, 572 (2019), pp. 116-119