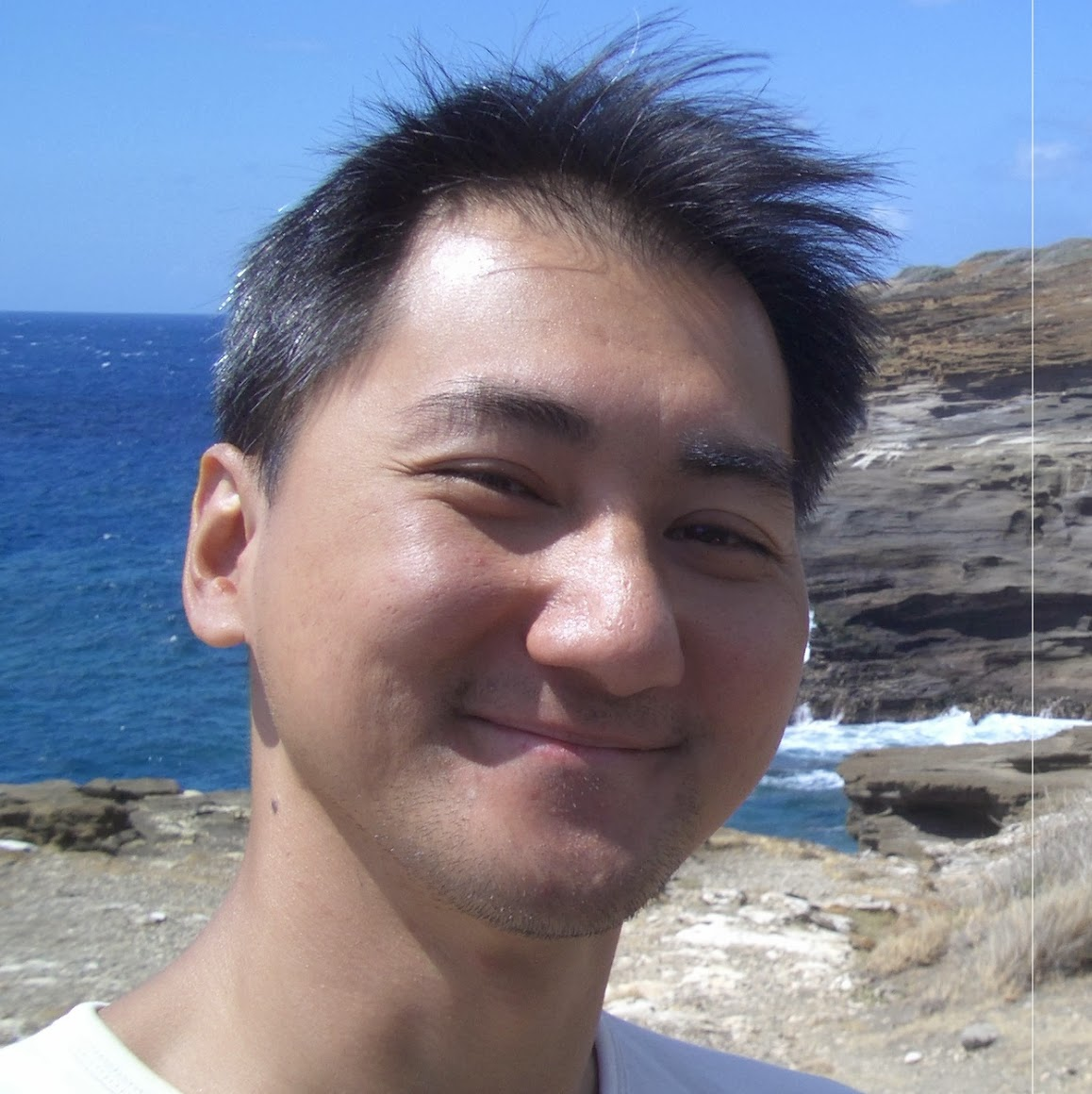
Vincent Wan
Research Areas
Authored Publications
Sort By
Google
Training Text-To-Speech Systems From Synthetic Data: A Practical Approach For Accent Transfer Tasks
Lev Finkelstein
Norman Casagrande
Ye Jia
Alexey Petelin
Jonathan Shen
Yu Zhang
Yonghui Wu
Interspeech (2022)
CHiVE: Varying Prosody in Speech Synthesis with a Linguistically Driven Dynamic Hierarchical Conditional Variational Network
Jakub Vit
Proceedings of the 36th International Conference on Machine Learning (ICML 2019), PMLR, pp. 3331-3340