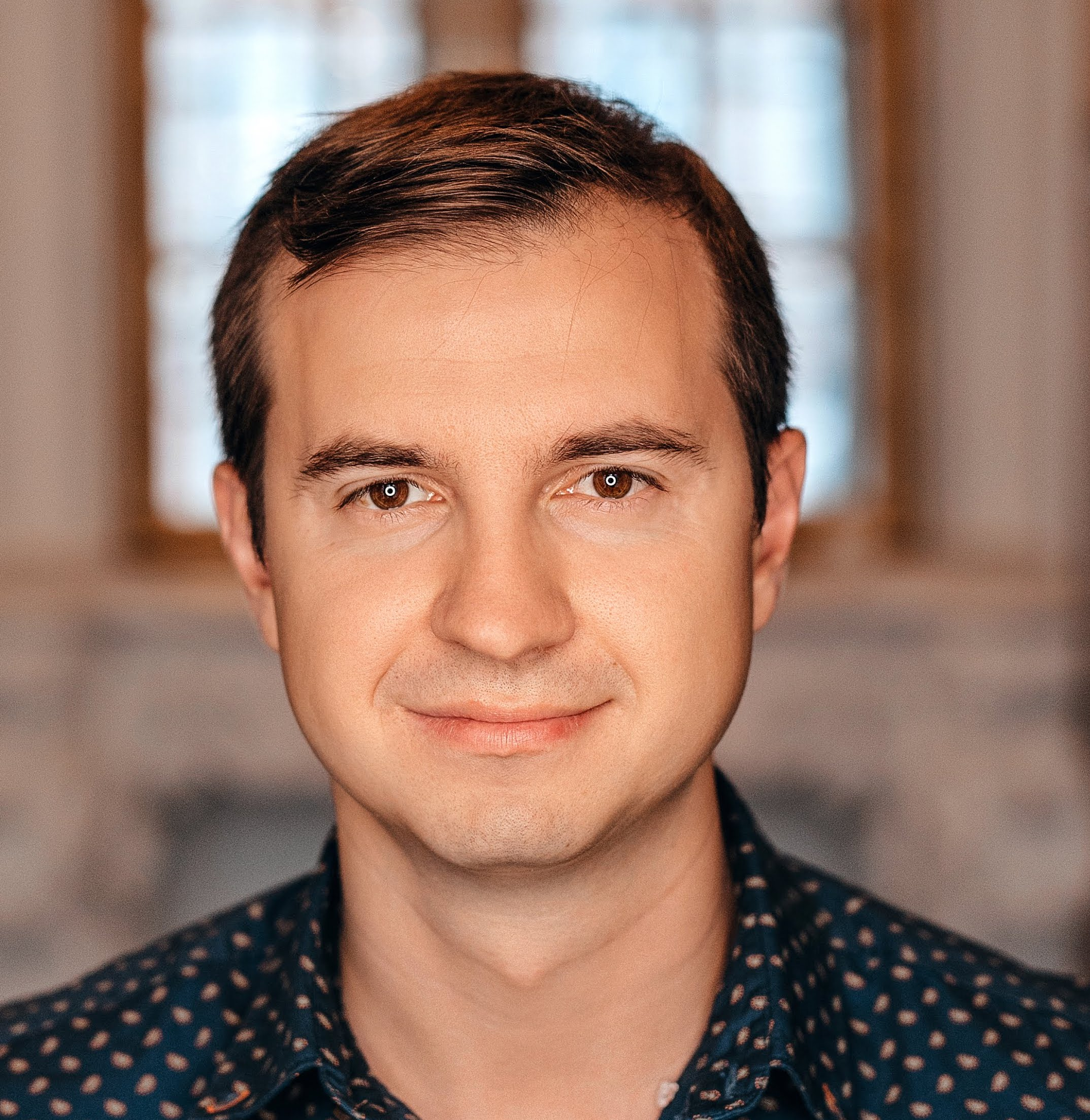
Mikhail Sirotenko
Mikhail is a Staff Software Engineer and Technical Lead Manager at Google Research where he leads a team working on computer vision and deep learning problems.
Research Areas
Authored Publications
Sort By
Google
Neptune: The Long Orbit to Benchmarking Long Video Understanding
Ramin Mehran
Rachel Hornung
Nitesh Bharadwaj Gundavarapu
Nilpa Jha
Austin Myers
Xingyi Zhou
Boqing Gong
Yukun Zhu
ArXiv (2024)
VideoPoet: A Large Language Model for Zero-Shot Video Generation
Dan Kondratyuk
Xiuye Gu
Jonathan Huang
Grant Schindler
Rachel Hornung
Vighnesh Birodkar
Jimmy Yan
Ming-Chang Chiu
Hassan Akbari
Josh Dillon
Agrim Gupta
Meera Hahn
Anja Hauth
David Hendon
Alonso Martinez
Kihyuk Sohn
Xuan Yang
Huisheng Wang
Lu Jiang
ICML (2024)
Fashionpedia: Ontology, Segmentation, and an Attribute Localization Dataset
Bharath Hariharan
Claire Cardie
Menglin Jia
Mengyun Shi
Serge Belongie
Yin Cui
(2020)