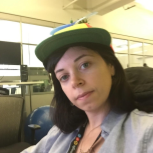
Michelle Tadmor Ramanovich
Michelle received her B.Sc from Tel Aviv University in Mathematics and later her M.S from Columbia University in Computer Science. She joined Google in 2016. At Google, she has worked on speech and translation related products and published research in areas that relate to automatic speech translation and dubbing.
Research Areas
Authored Publications
Sort By
Google
Spoken Question Answering and Speech Continuation Using Spectrogram-Powered LLM
Alon Levkovitch
Roy Hirsch
Chulayuth Asawaroengchai
Ehud Rivlin
ICLR (2024)
Translatotron 3: Speech to Speech Translation with Monolingual Data
Alon Levkovitch
Yifan Ding
Chulayuth Asawaroengchai
2024
More than Words: In-the-Wild Visually-Driven Text-to-Speech
Brendan Shillingford
Michael Eyov Hassid
Tal Remez
Ye Jia
CVPR, CVF CVPR-2022 (2022)