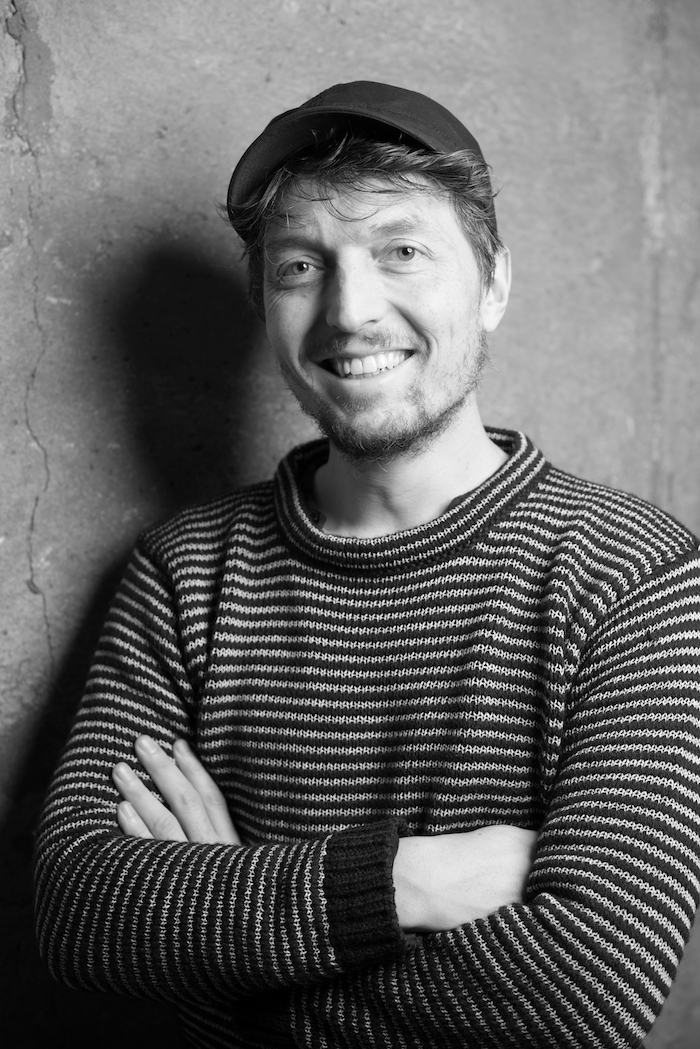
Lucas Dixon
Previously, he was Chief Scientist at Jigsaw where he founded engineering and research. He has contributed scientific advances and systems in multiple disciplines including digital security, formal logic, machine learning, and data visualization. For example he co-founded uProxy & Outline, Project Shield, DigitalAttackMap; Syria Defection Tracker, unfiltered.news, Conversation AI and Perspective API.
Before Google, Lucas completed his PhD and worked at the University of Edinburgh on the automation of mathematical reasoning and graphical languages applied to quantum information. He also helped run a non-profit working towards more rational and informed discussion and decision making, and was a co-founder of TheoryMine - a playful take on automating mathematical discovery. Outside of scientific advances, Lucas is also a martial arts instructor in Paris.
Research Areas
-
Algorithms and Theory
-
Data Management
-
Data Mining and Modeling
-
Distributed Systems and Parallel Computing
-
General Science
-
Human-Computer Interaction and Visualization
-
Information Retrieval and the Web
-
Machine Intelligence
-
Natural Language Processing
-
Networking
-
Security, Privacy and Abuse Prevention
-
Software Engineering
-
Software Systems