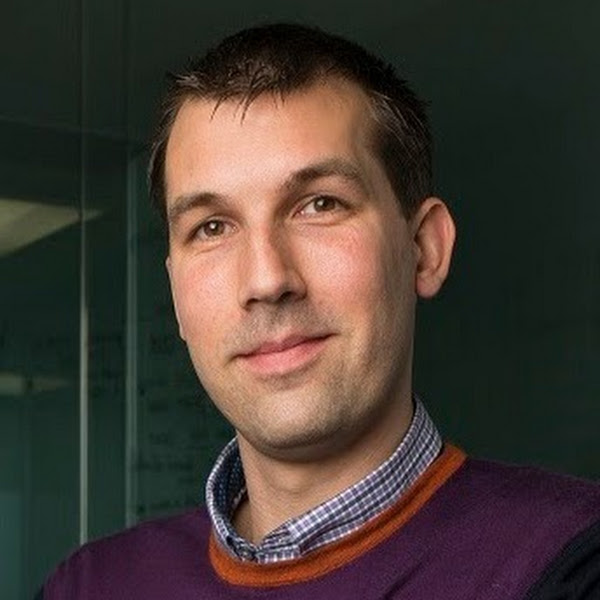
Filip Radlinski
Filip Radlinski is a research scientist at Google in London, UK. He received his PhD from Cornell University and a BSc (Hons) from the Australian National University. His research interests include conversational search and recommendation, online evaluation and machine learning.
More of his publications are listed on Google Scholar.
Authored Publications
Sort By
Google
Towards Realistic Synthetic User-Generated Content: A Scaffolding Approach to Generating Online Discussions
Barbara Ikica
Hamidreza Alvari
Mehdi Hafezi Manshadi
(2024)
Resolving Indirect Referring Expressions for Entity Selection
Silvia Pareti
Proceedings of the Annual Meetings of the Association for Computational Linguistics (ACL 2023)
Conversational Information Seeking
Hamed Zamani
Johanne R. Trippas
Jeff Dalton
Foundations and Trends® in Information Retrieval (2023), pp. 244-456
Beyond Single Items: Exploring User Preferences in Item Sets with the Conversational Playlist Curation Dataset
Arun Chaganty
Megan Leszczynski
Shu Zhang
Ravi Ganti
Proceedings of the 46th International ACM SIGIR Conference on Research and Development in Information Retrieval (2023)
Measuring the Impact of Explanation Bias: A Study of Natural Language Justifications for Recommender Systems
Andrey Petrov
Extended Abstracts of the 2023 CHI Conference on Human Factors in Computing Systems (CHI EA '23), ACM
Large Language Models are Competitive Near Cold-start Recommenders for Language- and Item-based Preferences
Scott Sanner
Proceedings of ACM Conference on Recommender Systems (RecSys ’23) (2023) (to appear)
On Natural Language User Profiles for Transparent and Scrutable Recommendation
Proceedings of the 45th International ACM SIGIR Conference on Research and Development in Information Retrieval (SIGIR '22) (2022)
Subjective Attributes in Conversational Recommendation Systems: Challenges and Opportunities
Ivan Vendrov
Proceedings of the 36th AAAI Conference on Artificial Intelligence (AAAI-22) (2022), pp. 12287-12293
Conversational Music Retrieval with Synthetic Data
Megan Eileen Leszczynski
Ravi Ganti
Shu Zhang
Arun Tejasvi Chaganty
Second Workshop on Interactive Learning for Natural Language Processing at NeurIPS 2022