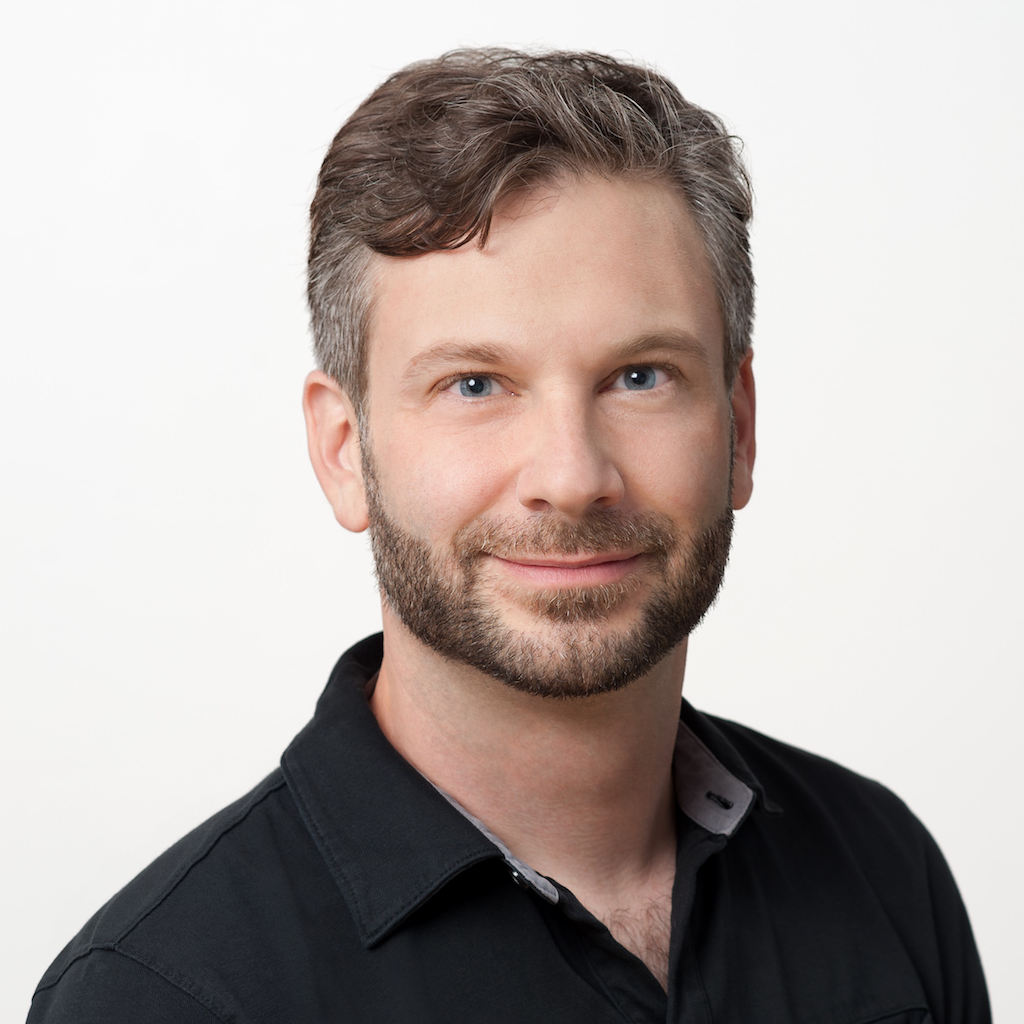
Greg Corrado
Greg Corrado is a senior research scientist interested in biological neuroscience, artificial intelligence, and scalable machine learning. He has published in fields ranging across behavioral economics, neuromorphic device physics, systems neuroscience, and deep learning. At Google he has worked for some time on brain inspired computing, and most recently has served as one of the founding members and the co-technical lead of Google's large scale deep neural networks project.
Authored Publications
Sort By
Google
Triaging mammography with artificial intelligence: an implementation study
Sarah M. Friedewald
Sunny Jansen
Fereshteh Mahvar
Timo Kohlberger
David V. Schacht
Sonya Bhole
Dipti Gupta
Scott Mayer McKinney
Stacey Caron
David Melnick
Mozziyar Etemadi
Samantha Winter
Alejandra Maciel
Luca Speroni
Martha Sevenich
Arnav Agharwal
Rubin Zhang
Gavin Duggan
Shiro Kadowaki
Atilla Kiraly
Jie Yang
Basil Mustafa
Krish Eswaran
Shravya Shetty
Breast Cancer Research and Treatment (2025)
Performance of a Deep Learning Diabetic Retinopathy Algorithm in India
Arthur Brant
Xiang Yin
Lu Yang
Jay Nayar
Divleen Jeji
Sunny Virmani
Anchintha Meenu
Naresh Babu Kannan
Florence Thng
Lily Peng
Ramasamy Kim
JAMA Network Open (2025)
Predicting Cardiovascular Disease Risk using Photoplethysmography and Deep Learning
Sebastien Baur
Christina Chen
Mariam Jabara
Babak Behsaz
Shravya Shetty
Goodarz Danaei
Diego Ardila
PLOS Global Public Health, 4(6) (2024), e0003204
An intentional approach to managing bias in embedding models
Atilla P. Kiraly
Jungyeon Park
Rory Pilgrim
Charles Lau
Heather Cole-Lewis
Shravya Shetty
Krish Eswaran
Leo Anthony Celi
The Lancet Digital Health, 6 (2024), E126-E130
Conversational AI in health: Design considerations from a Wizard-of-Oz dermatology case study with users, clinicians and a medical LLM
Brenna Li
Amy Wang
Patricia Strachan
Julie Anne Seguin
Sami Lachgar
Karyn Schroeder
Renee Wong
Extended Abstracts of the 2024 CHI Conference on Human Factors in Computing Systems, Association for Computing Machinery, pp. 10
Creating an Empirical Dermatology Dataset Through Crowdsourcing With Web Search Advertisements
Abbi Ward
Jimmy Li
Julie Wang
Sriram Lakshminarasimhan
Ashley Carrick
Jay Hartford
Pradeep Kumar S
Sunny Virmani
Renee Wong
Margaret Ann Smith
Dawn Siegel
Steven Lin
Justin Ko
JAMA Network Open (2024)
PathAlign: A vision–language model for whole slide images in histopathology
Lin Yang
Shawn Xu
Abbi Ward
Niels Olson
Arash Mohtashamian
Shravya Shetty
Daniel Golden
Yun Li
(2024)
General Geospatial Inference with a Population Dynamics Foundation Model
Chaitanya Kamath
Prithul Sarker
Joydeep Paul
Yael Mayer
Sheila de Guia
Jamie McPike
Adam Boulanger
David Schottlander
Yao Xiao
Manjit Chakravarthy Manukonda
Monica Bharel
Von Nguyen
Luke Barrington
Niv Efron
Krish Eswaran
Shravya Shetty
(2024) (to appear)
Towards Generalist Biomedical AI
Danny Driess
Andrew Carroll
Chuck Lau
Ryutaro Tanno
Ira Ktena
Basil Mustafa
Aakanksha Chowdhery
Simon Kornblith
Philip Mansfield
Sushant Prakash
Renee Wong
Sunny Virmani
Sara Mahdavi
Bradley Green
Ewa Dominowska
Joelle Barral
Karan Singhal
Pete Florence
NEJM AI (2024)
Health equity assessment of machine learning performance (HEAL): a framework and dermatology AI model case study
Terry Spitz
Malcolm Chelliah
Heather Cole-Lewis
Stephanie Farquhar
Qinghan Xue
Jenna Lester
Cían Hughes
Patricia Strachan
Fraser Tan
Peggy Bui
Craig Mermel
Lily Peng
Sunny Virmani
Ivor Horn
Cameron Chen
The Lancet eClinicalMedicine (2024)