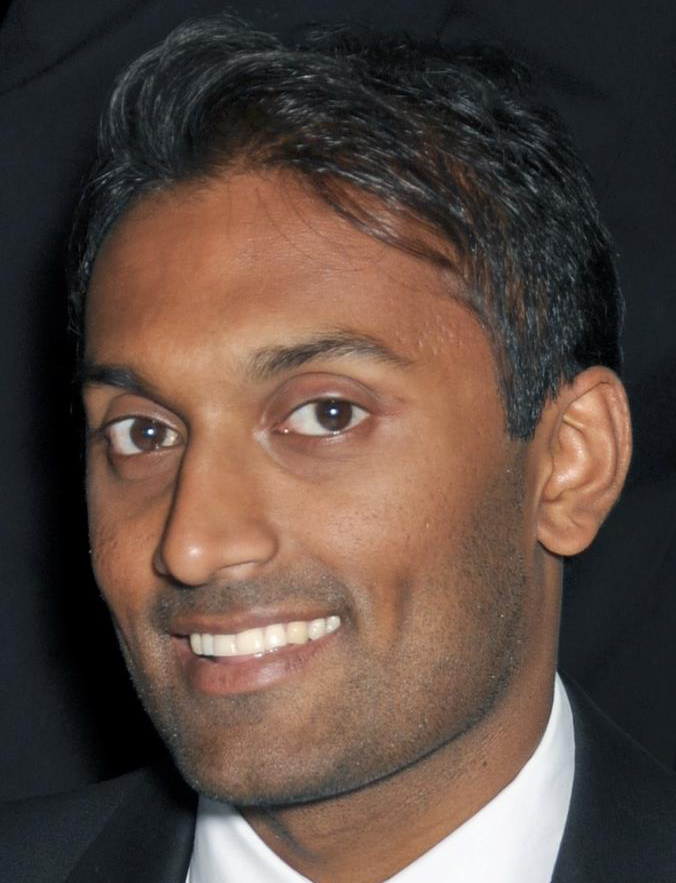
Gautam Prasad
Gautam Prasad is a researcher on the Mobile Vision team at Google. His work revolves around understanding images, videos, audio, and motion sensor data by building datasets and using a variety of machine learning tools to better model and understand affect, gaze, and intent. Prior to his work at Google he was an Assistant Professor in the Neurology Department at the University of Southern California (USC). His research at USC focused on using machine learning techniques to mine the patterns of human brain connectivity in magnetic resonance imaging (MRI) images.
Authored Publications
Sort By
Socio-spatial equity analysis of relative wealth index and emergency obstetric care accessibility in urban Nigeria
Ibukun-Oluwa Omolade Abejirinde
Kerry L. M. Wong
Narayanan Sundararajan
Peter M. Macharia
Aduragbemi Banke-Thomas
Tope Olubodun
Lenka Beňová
Bosede B. Afolabi
Prestige Tatenda Makanga
Jia Wang
Uchenna Gwacham-Anisiobi
Olakunmi Ogunyemi
Mansi Kansal
Charlotte Stanton
Yash Shah
Swapnil Vispute
Communications Medicine, 4 (2024), pp. 34
Preview abstract
Background
Better geographical accessibility to comprehensive emergency obstetric care (CEmOC) facilities can significantly improve pregnancy outcomes. However, with other factors, such as affordability critical for care access, it is important to explore accessibility across groups. We assessed CEmOC geographical accessibility by wealth status in the 15 most-populated Nigerian cities.
Methods
We mapped city boundaries, verified and geocoded functional CEmOC facilities, and assembled population distribution for women of childbearing age and Meta’s Relative Wealth Index (RWI). We used the Google Maps Platform’s internal Directions Application Programming Interface to obtain driving times to public and private facilities. City-level median travel time (MTT) and number of CEmOC facilities reachable within 60 min were summarised for peak and non-peak hours per wealth quintile. The correlation between RWI and MTT to the nearest public CEmOC was calculated.
Results
We show that MTT to the nearest public CEmOC facility is lowest in the wealthiest 20% in all cities, with the largest difference in MTT between the wealthiest 20% and least wealthy 20% seen in Onitsha (26 vs 81 min) and the smallest in Warri (20 vs 30 min). Similarly, the average number of public CEmOC facilities reachable within 60 min varies (11 among the wealthiest 20% and six among the least wealthy in Kano). In five cities, zero facilities are reachable under 60 min for the least wealthy 20%. Those who live in the suburbs particularly have poor accessibility to CEmOC facilities.
Conclusions
Our findings show that the least wealthy mostly have poor accessibility to care. Interventions addressing CEmOC geographical accessibility targeting poor people are needed to address inequities in urban settings.
View details
General Geospatial Inference with a Population Dynamics Foundation Model
Yao Xiao
Krish Eswaran
Shravya Shetty
Niv Efron
Chaitanya Kamath
Adam Boulanger
Luke Barrington
Manjit Chakravarthy Manukonda
Von Nguyen
David Schottlander
Yael Mayer
Joydeep Paul
Jamie McPike
Prithul Sarker
Sheila de Guia
Monica Bharel
(2024) (to appear)
Preview abstract
Supporting the health and well-being of dynamic populations around the world requires governmental agencies, organizations, and researchers to understand and reason over complex relationships between human behavior and local contexts. This support includes identifying populations at elevated risk and gauging where to target limited aid resources. Traditional approaches to these classes of problems often entail developing manually curated, task-specific features and models to represent human behavior and the natural and built environment, which can be challenging to adapt to new, or even related tasks. To address this, we introduce the Population Dynamics Foundation Model (PDFM), which aims to capture the relationships between diverse data modalities and is applicable to a broad range of geospatial tasks. We first construct a geo-indexed dataset for postal codes and counties across the United States, capturing rich aggregated information on human behavior from maps, busyness, and aggregated search trends, and environmental factors such as weather and air quality. We then model this data and the complex relationships between locations using a graph neural network, producing embeddings that can be adapted to a wide range of downstream tasks using relatively simple models. We evaluate the effectiveness of our approach by benchmarking it on 27 downstream tasks spanning three distinct domains: health indicators, socioeconomic factors, and environmental measurements. The approach achieves state-of-the-art performance on geospatial interpolation across all tasks, surpassing existing satellite and geotagged image based location encoders. In addition, it achieves state-of-the-art performance in extrapolation and super-resolution for 25 of the 27 tasks. We also show that the PDFM can be combined with a state-of-the-art forecasting foundation model, TimesFM, to predict unemployment and poverty, achieving performance that surpasses fully supervised forecasting. The full set of embeddings and sample code are publicly available for researchers. In conclusion, we have demonstrated a general purpose approach to geospatial modeling tasks critical to understanding population dynamics by leveraging a rich set of complementary globally available datasets that can be readily adapted to previously unseen machine learning tasks.
View details
Community search signatures as foundation features for human-centered geospatial modeling
Shravya Shetty
Niv Efron
Chaitanya Kamath
Mohit Agarwal
David Schottlander
Shailesh Bavadekar
ICML 2024 Workshop on Data-Centric Machine Learning Research
Preview abstract
Aggregated relative search frequencies offer a unique composite signal reflecting people's habits, concerns, interests, intents, and general information needs, which are not found in other readily available datasets. Temporal search trends have been successfully used to perform nowcasting across a variety of domains such as infectious diseases, unemployment rates, and retail sales. However, most existing applications require curating specialized datasets of individual keywords, queries, or query clusters, and the search data need to be temporally aligned with the outcome variable of interest. We propose a novel approach for generating an aggregated and anonymized representation of search interest as foundation features at the community level for geospatial modeling. We benchmark these features using spatial datasets across multiple domains. In regions with a population greater than 3000 that cover over 95% of the contiguous US population, our models achieve an average R-squared score of 0.74 across 21 health variables, and 0.80 across 6 demographic and environmental variables. Our results demonstrate that these search features can be used for spatial predictions without strict temporal alignment, and that the resulting models outperform spatial interpolation and state of the art methods using satellite imagery features.
View details
Geographical accessibility to emergency obstetric care in urban Nigeria using closer-to-reality travel time estimates
Ngozi Azodoh
Narayanan Sundararajan
Prestige Tatenda Makanga
Kerry L. M. Wong
Aduragbemi Banke-Thomas
Jia Wang
Peter M. Macharia
Ibukun-Oluwa Omolade Abejirinde
Bosede B. Afolabi
Uchenna Gwacham-Anisiobi
Tope Olubodun
Charles Nzelu, PhD
Lenka Beňová
Olakunmi Ogunyemi
Mansi Kansal
Charlotte Stanton
Yash Shah
Swapnil Vispute
Lancet Global Health (2024)
Preview abstract
Background
Better accessibility of emergency obstetric care (CEmOC) facilities can significantly reduce maternal and perinatal deaths. However, pregnant women living in urban settings face additional complex challenges travelling to facilities. We estimated geographical accessibility and coverage to the nearest, second nearest, and third nearest public and private CEmOC facilities in the 15 largest Nigerian cities.
Methods
We mapped city boundaries, verified and geocoded functional CEmOC facilities, and assembled population distribution for women of childbearing age (WoCBA). We used Google Maps Platform’s internal Directions Application Programming Interface (API) to derive driving times to public, private, or either facility-type. Median travel time (MTT) and percentage of WoCBA able to reach care were summarised for eight traffic scenarios (peak and non-peak hours on weekdays and weekends) by city and within-city (wards) under different travel time thresholds (<15, <30, <60 min).
Findings
City-level MTT to the nearest CEmOC facility ranged from 18min (Maiduguri) to 46min (Kaduna). Within cities, MTT varied by location, with informal settlements and peripheral areas being the worst off. The percentages of WoCBA within 60min to their nearest public CEmOC were nearly universal; whilst the percentages of WoCBA within 30min reach to their nearest public CEmOC were between 33% in Aba to over 95% in Ilorin and Maiduguri. During peak traffic times, the median number of public CEmOC facilities reachable by WoCBA under 30min was zero in eight of 15 cities.
Interpretation
This approach provides more context-specific, finer, and policy-relevant evidence to support improving CEmOC service accessibility in urban Africa.
View details
A geospatial database of close to reality travel times to obstetric emergency care in 15 Nigerian conurbations
Ibukun-Oluwa Omolade Abejirinde
Aduragbemi Banke-Thomas
Bosede B. Afolabi
Olakunmi Ogunyemi
Kerry L. M. Wong
Prestige Tatenda Makanga
Lenka Beňová
Narayanan Sundararajan
Jia Wang
Tope Olubodun
Uchenna Gwacham-Anisiobi
Peter M. Macharia
Mansi Kansal
Charlotte Stanton
Yash Shah
Swapnil Vispute
Scientific Data, TBD (2023), TBD
Preview abstract
Travel time estimation accounting for on-the-ground realities between the location where a need for emergency obstetric care (EmOC) arises and the health facility capable of providing such services is essential for improving maternal and neonatal health outcomes. Current understanding of travel time to care is particularly inadequate in urban areas where short distances obscure long travel times, and also in low-resource settings. Here, we describe a database of travel times to facilities that can provide comprehensive EmOC in the 15 most populated extended urban areas (conurbations) in Nigeria. The travel times from cells of approximately 0.6 x 0.6km to facilities were derived based on Google Maps Platform’s internal Directions Application Programming Interface (API). The API incorporates estimates of traffic to provide closer-to-reality estimates of travel time. Computations were done to the first, second and third nearest public or private facilities. Travel time estimates for eight traffic scenarios (including peak and non-peak periods) and number of facilities within specific time thresholds were estimated. The database offers a plethora of opportunities for research and planning towards improving EmOC accessibility.
View details
Sixteen facial expressions occur in similar contexts worldwide
Dacher Keltner
Alan Cowen
Nature, 589 (2020), pp. 251-257
Preview abstract
Understanding the degree to which human facial expressions co-vary with specific social contexts across cultures is central to the theory that emotions enable adaptive responses to important challenges and opportunities. Concrete evidence linking social context to specific facial expressions is sparse and is largely based on survey-based approaches, which are often constrained by language and small sample sizes. Here, by applying machine-learning methods to real-world, dynamic behaviour, we ascertain whether naturalistic social contexts (for example, weddings or sporting competitions) are associated with specific facial expressions across different cultures. In two experiments using deep neural networks, we examined the extent to which 16 types of facial expression occurred systematically in thousands of contexts in 6 million videos from 144 countries. We found that each kind of facial expression had distinct associations with a set of contexts that were 70% preserved across 12 world regions. Consistent with these associations, regions varied in how frequently different facial expressions were produced as a function of which contexts were most salient. Our results reveal fine-grained patterns in human facial expressions that are preserved across the modern world.
View details
Preview abstract
Videos can evoke a range of affective responses in viewers. The ability to predict evoked affect from a video, before viewers watch the video, can help in content creation and video recommendation. We introduce the Evoked Expressions from Videos (EEV) dataset, a large-scale dataset for studying viewer responses to videos. Each video is annotated at 6 Hz with 15 continuous evoked expression labels, corresponding to the facial expression of viewers who reacted to the video. We use an expression recognition model within our data collection framework to achieve scalability. In total, there are 36.7 million annotations of viewer facial reactions to 23,574 videos (1,700 hours). We use a publicly available video corpus to obtain a diverse set of video content. We establish baseline performance on the EEV dataset using an existing multimodal recurrent model. Transfer learning experiments show an improvement in performance on the LIRIS-ACCEDE video dataset when pre-trained on EEV. We hope that the size and diversity of the EEV dataset will encourage further explorations in video understanding and affective computing. A subset of EEV is released at https://github.com/google-research-datasets/eev.
View details
Preview abstract
The visual and audio information from movies can evoke a variety of emotions in viewers. Towards a better understanding of viewer impact, we present our methods for the MediaEval 2018 Emotional Impact of Movies Task to predict the expected valence and arousal continuously in movies. This task, using the LIRIS-ACCEDE dataset, enables researchers to compare different approaches for predicting viewer impact from movies. Our approach leverages image, audio, and face based features computed using pre-trained neural networks. These features were computed over time and modeled using a gated recurrent unit (GRU) based network followed by a mixture of experts model to compute multiclass predictions. We smoothed these predictions using a Butterworth filter for our final result. Our method enabled us to achieve top performance in three evaluation metrics in the MediaEval 2018 task.
View details
Weakly Supervised Action Localization by Sparse Temporal Pooling Network
Bohyung Han
Phuc Nguyen
Proceedings of the IEEE Conference on Computer Vision and Pattern Recognition (2018), pp. 6752-6761
Preview abstract
We propose a weakly supervised temporal action localization algorithm on untrimmed videos using convolutional neural networks. Our algorithm learns from video-level class labels and predicts temporal intervals of human actions with no requirement of temporal localization annotations. We design our network to identify a sparse subset of key segments associated with target actions in a video using an attention module and fuse the key segments through adaptive temporal pooling. Our loss function is comprised of two terms that minimize the video-level action classification error and enforce the sparsity of the segment selection. At inference time, we extract and score temporal proposals using temporal class activations and class-agnostic attentions to estimate the time intervals that correspond to target actions. The proposed algorithm attains state-of-the-art results on the THUMOS14 dataset and outstanding performance on ActivityNet1.3 even with its weak supervision.
View details