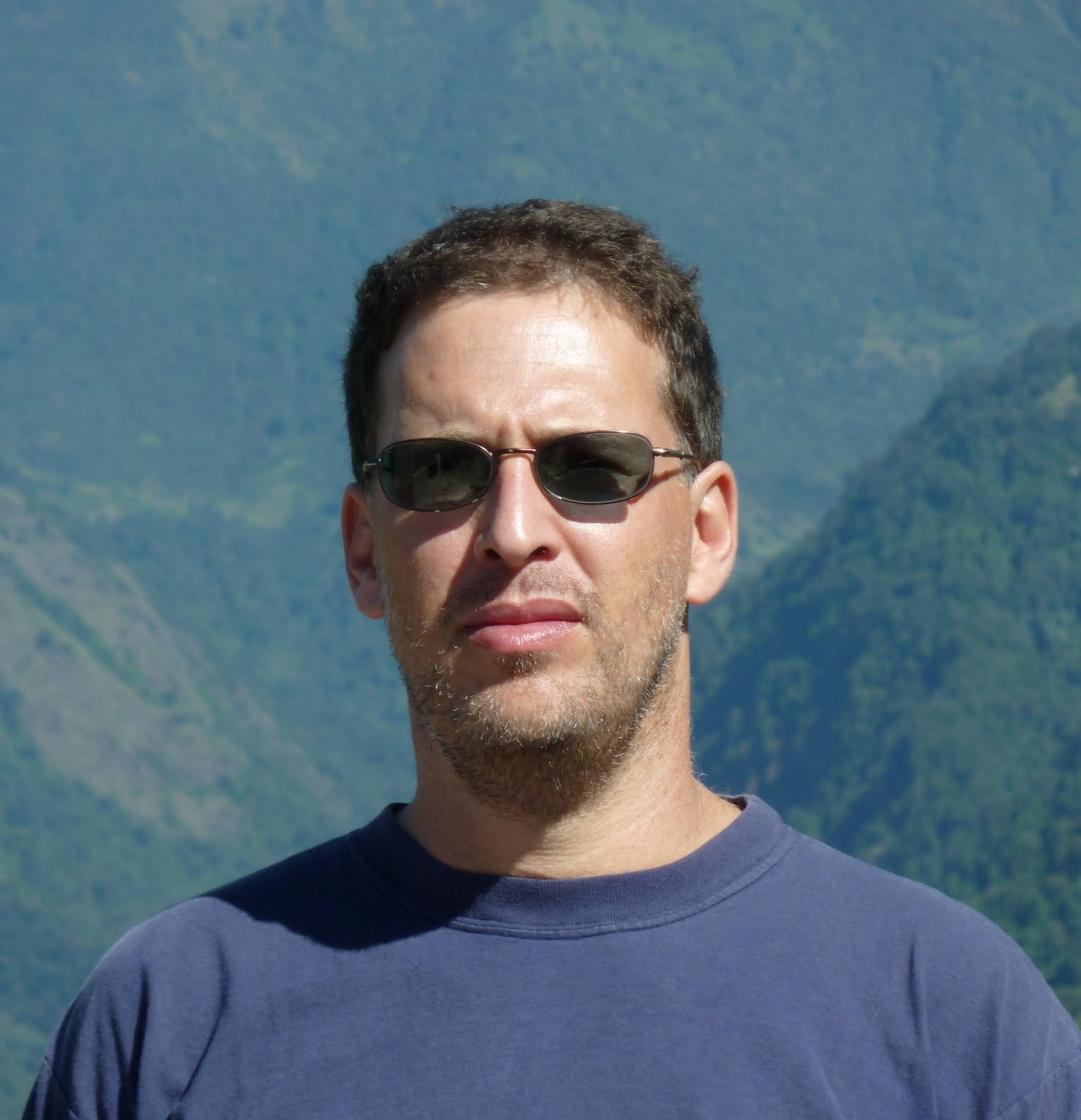
Dotan Emanuel
Dotan Emanuel works on the Audio biomarkers team in Google research, Tel-Aviv. Prior to this position Dotan managed the cloud network-telemetry and fault detection teams in Google cloud. Before joining Google Dotan held a leading technical position in a few Israeli startups; Genio (news personalization) which was acquired by Somoto, Discretix (mobile security) acquired by ARM, Telmap (mobile navigation) acquired by Intel, Appstream (early SaaS) & Comfy (games for young kids).
Dotan Emanuel received his B.SC and M.Sc in computer science from Tel aviv university 1997 and 2003 respectively, and holds an MBA from Recanati Graduate School of Business, TAU (2008).
Research Areas
Authored Publications
Sort By
Preview abstract
Computing efficient traffic signal plans is often based on the amount of traffic in an intersection, its distribution over the various intersection movements and hours as well as on performance metrics such as traffic delay. In their simple and typical form plans are fixed in the same hour over weekdays. This allows low operation costs without the necessity for traffic detection and monitoring tools. A critical factor on the potential efficiency of such plans is the similarity of traffic patterns over the days along each of the intersection movements. We refer to such similarity as the traffic stability of the intersection and define simple metrics to measure it based on traffic volume and traffic delay. In this paper, we propose an automatic probe data based method, for city-wide estimation of traffic stability. We discuss how such measures can be used for signal planning such as in selecting plan resolution or as an indication as which intersections can benefit from dynamic but expensive traffic detection tools. We also identify events of major changes in traffic characteristics of an intersection. We demonstrate the framework by using real traffic statistics to study the traffic stability in the city of Haifa along its 162 intersections. We study the impact of the time of day on the stability, detect major changes in traffic and find intersections with high and low stability.
View details
Shared computational principles for language processing in humans and deep language models
Gina Choe
Amy Price
Colton Casto
Liat Hasenfratz
Roi Reichart
Omer Levy,
Adeen Flinker
Bobbi Aubrey
Zaid Zada
Kenneth A. Norman
Lora Fanda
Daniel Friedman
Orrin Devinsky
Catherine Kim
Aditi Rao
Uri Hasson
Lucia Melloni
Harshvardhan Gazula
Samuel A. Nastase
Patricia Dugan
Werner Doyle
Sasha Devore
Eliav Buchnik
Ariel Goldstein
Nature Neuroscience (2022)
Preview abstract
Departing from traditional linguistic models, advances in deep learning have resulted in a new type of predictive (autoregressive) deep language models (DLMs). Using a self-supervised next-word prediction task, these models generate appropriate linguistic responses in a given context. In the current study, nine participants listened to a 30-min podcast while their brain responses were recorded using electrocorticography (ECoG). We provide empirical evidence that the human brain and autoregressive DLMs share three fundamental computational principles as they process the same natural narrative: (1) both are engaged in continuous next-word prediction before word onset; (2) both match their pre-onset predictions to the incoming word to calculate post-onset surprise; (3) both rely on contextual embeddings to represent words in natural contexts. Together, our findings suggest that autoregressive DLMs provide a new and biologically feasible computational framework for studying the neural basis of language.
View details
Towards Learning a Universal Non-Semantic Representation of Speech
Marco Tagliasacchi
Joel Shor
Ronnie Zvi Maor
Ira Shavitt
Proc. Interspeech 2020 (2020)
Preview abstract
The ultimate goal of transfer learning is to enable learning with a small amount of data, by using a strong embedding. While significant progress has been made in the visual and language domains, the speech domain does not have such a universal method. This paper presents a new representation of speech signals based on an unsupervised triplet-loss objective, which outperforms both existing state of the art and other representations on a number of transfer learning tasks in the non-semantic speech domain. The embedding is learned on a publicly available dataset, and it is tested on a variety of low-resource downstream tasks, including personalization tasks and medical domain. The model will be publicly released.
View details
Personalizing ASR for Dysarthric and Accented Speech with Limited Data
Joel Shor
Interspeech 2019 (2019)
Preview abstract
Automatic speech recognition (ASR) systems have dramatically
improved over the last few years. ASR systems are most often trained from ‘typical’ speech, which means that underrepresented groups don’t experience the same level of improvement.
In this paper, we present and evaluate finetuning techniques to
improve ASR for users with non standard speech. We focus
on two types of non standard speech: speech from people with
amyotrophic lateral sclerosis (ALS) and accented speech. We
train personalized models that achieve 62% and 35% relative
WER improvement on these two groups, bringing the absolute
WER for ALS speakers, on a test set of message bank phrases,
to 10% for mild dysarthria and 20% for more serious dysarthria.
We show that 76% of the improvement comes from only 5 min
of training data. Finetuning a particular subset of layers (with
many fewer parameters) often gives better results than finetuning the entire model. This is the first step towards building state
of the art ASR models for dysarthric speech
Index Terms: speech recognition, personalization, accessibility
View details