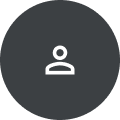
David Reitter
Reitter's research interests cover diverse areas of computational cognitive science. Reitter, with academic colleagues, started a subfield of psycholinguistics that used large-scale observational datasets to understand how the mind processes language and allows us to engage in conversation.
David Reitter has authored more than 120 papers in both cognitive psychology and computer science, and Aquamacs, a widely used software package. Prof. Reitter joined Google from Penn State, where he directed an NSF-funded research group on computational cognition and language processing. He holds a Ph.D. from the University of Edinburgh, prior degrees in linguistics and computer science, and was a fellow at MIT's Media Lab Europe (working on multimodal user interfaces) and a post-doc at Carnegie Mellon University (working on cognitive modeling).