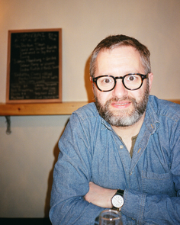
Cyril Allauzen
Cyril Allauzen is a research scientist at Google in New York. His main research interests are in finite-state methods and their applications to text, speech and natural language processing and machine learning. Before joining Google, he worked as a researcher at AT&T Labs Research and at NYU's Courant Institute of Mathematical Sciences. Cyril received his Ph.D. in computer science from the Université de Marne-la-Vallée in 2001.
Cyril is an author of the OpenFst Library, the OpenKernel Library and the GRM Library.
Authored Publications
Sort By
Google
On Weight Interpolation of the Hybrid Autoregressive Transducer Model
David Rybach
Interspeech 2022, Interspeech 2022 (2022) (to appear)
E2E Segmenter: Joint Segmenting and Decoding for Long-Form ASR
David Rybach
Cal Peyser
Zhiyun Lu
Interspeech 2022 (2022) (to appear)
An Efficient Streaming Non-Recurrent On-Device End-to-End Model with Improvements to Rare-Word Modeling
Rami Botros
Ruoming Pang
David Johannes Rybach
James Qin
Quoc-Nam Le-The
Anmol Gulati
Cal Peyser
Chung-Cheng Chiu
Emmanuel Guzman
Jiahui Yu
Qiao Liang
Wei Li
Yonghui Wu
Yu Zhang
Interspeech (2021) (to appear)
Hybrid Autoregressive Transducer (HAT)
David Rybach
ICASSP 2020 - 2020 IEEE International Conference on Acoustics, Speech and Signal Processing, Barcelona, Spain, pp. 6139-6143
Federated Learning of N-gram Language Models
Adeline Wong
The SIGNLL Conference on Computational Natural Language Learning (2019)
Contextual Recovery of Out-of-Lattice Named Entities in Automatic Speech Recognition
Jack Serrino
ISCA Interspeech 2019, ISCA, Graz, Austria (2019), pp. 3830-3834
Algorithms for Weighted Finite Automata with Failure Transitions
International Conference of Implementation and Applications of Automata (CIAA) (2018), pp. 46-58
Transliterated mobile keyboard input via weighted finite-state transducers
Lars Hellsten
Prasoon Goyal
David Rybach
Proceedings of the 13th International Conference on Finite State Methods and Natural Language Processing (FSMNLP) (2017)
Distributed representation and estimation of WFST-based n-gram models
Proceedings of the ACL Workshop on Statistical NLP and Weighted Automata (StatFSM) (2016), pp. 32-41