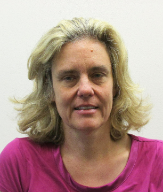
Cordelia Schmid
Cordelia Schmid holds a M.S. degree in Computer Science from the
University of Karlsruhe and a Doctorate, also in Computer Science,
from the Institut National Polytechnique de Grenoble (INPG). Her
doctoral thesis received the best thesis award from INPG in 1996.
Dr. Schmid was a post-doctoral research assistant in the Robotics
Research Group of Oxford University in 1996--1997. Since 1997 she has
held a permanent research position at Inria Grenoble Rhone-Alpes,
where she is a research director and directs an Inria team. Dr. Schmid
has been an Associate Editor for IEEE PAMI (2001--2005) and for IJCV
(2004--2012), editor-in-chief for IJCV (2013---), a program chair of
IEEE CVPR 2005 and ECCV 2012 as well as a general chair of IEEE CVPR
2015 and ECCV 2020. In 2006, 2014 and 2016, she was awarded the
Longuet-Higgins prize for fundamental contributions in computer vision
that have withstood the test of time. She is a fellow of IEEE. She was
awarded an ERC advanced grant in 2013, the Humbolt research award in
2015 and the Inria & French Academy of Science Grand Prix in 2016. She
was elected to the German National Academy of Sciences, Leopoldina, in 2017.
She is working for Google France starting Feb. 2018 part-time (50%).
For more information see http://thoth.inrialpes.fr/people/schmid.
Research Areas
Authored Publications
Sort By
Google
Neptune: The Long Orbit to Benchmarking Long Video Understanding
Ramin Mehran
Rachel Hornung
Nitesh Bharadwaj Gundavarapu
Nilpa Jha
Austin Myers
Xingyi Zhou
Boqing Gong
Yukun Zhu
ArXiv (2024)
UnLoc: a unified framework for video localization tasks
Shen Yan
Xuehan Xiong
Anurag Arnab
Zhonghao Wang
Weina Ge
International Conference on Computer Vision (2023)
Multiview Transformers for Video Recognition
Shen Yan
Xuehan Xiong
Anurag Arnab
Zhichao Lu
Mi Zhang
The IEEE / CVF Computer Vision and Pattern Recognition Conference (CVPR) (2022)
Masking Modalities for Cross-modal Video Retrieval
Valentin Gabeur
Karteek Alahari
Winter Conference on Applications of Computer Vision (WACV) (2022) (to appear)
TL;DW? Summarizing Instructional Videos with Task Relevance & Cross-Modal Saliency
Anna Rohrbach
Medhini Narasimhan
Trevor Darrell
European Conference on Computer Vision (2022)
AVATAR: Unconstrained Audiovisual Speech Recognition
Valentin Gabeur
Paul Hongsuck Seo
Karteek Alahari
Interspeech (2022)
Learning Audio-Video Modalities from Image Captions
Paul Hongsuck Seo
Anja Hauth
Santiago Manen
European Conference on Computer Vision (2022)