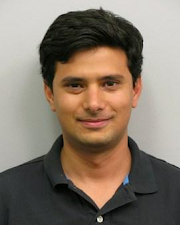
Aranyak Mehta
Research Areas
Authored Publications
Sort By
Google
Auto-bidding and Auctions in Online Advertising: A Survey
Ashwinkumar Badanidiyuru Varadaraja
Christopher Liaw
Haihao (Sean) Lu
Andres Perlroth
Georgios Piliouras
Ariel Schvartzman
Kelly Spendlove
Hanrui Zhang
Mingfei Zhao
ACM SIGecom Exchanges, 22 (2024)
Incentive Compatibility in the Auto-bidding World
Yeganeh Alimohammadi
Andres Perlroth
24th ACM Conference on Economics and Computation, EC 2023
Simple Mechanisms for Welfare Maximization in Rich Advertising Auctions
Divyarthi Mohan
Alex Psomas
Advances in Neural Information Processing Systems 35 (NeurIPS 2022) Main Conference Track
Auction design in an auto-bidding setting: Randomization improves efficiency beyond VCG
Proceedings of the ACM Web Conference 2022, pp. 173-181
Convergence Analysis of No-Regret Bidding Algorithms in Repeated Auctions
Zhe Feng
Guru Prashanth Guruganesh
Chris Liaw
Abhishek Sethi
The Thirty-Fifth AAAI Conference on Artificial Intelligence (AAAI-21) (2021)