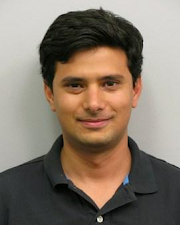
Aranyak Mehta
Research Areas
Authored Publications
Sort By
Auto-bidding and Auctions in Online Advertising: A Survey
Ashwinkumar Badanidiyuru Varadaraja
Christopher Liaw
Haihao (Sean) Lu
Andres Perlroth
Georgios Piliouras
Ariel Schvartzman
Kelly Spendlove
Hanrui Zhang
Mingfei Zhao
ACM SIGecom Exchanges, 22 (2024)
Preview abstract
In this survey, we summarize recent developments in research fueled by the growing adoption of automated bidding strategies in online advertising. We explore the challenges and opportunities that have arisen as markets embrace this autobidding and cover a range of topics in this area, including bidding algorithms, equilibrium analysis and efficiency of common auction formats, and optimal auction design.
View details
Preview abstract
We propose a new Markov Decision Process (MDP) model for ad auctions to capture the
user response to the quality of ads, with the objective of maximizing the long-term discounted
revenue. By incorporating user response, our model takes into consideration all three parties
involved in the auction (advertiser, auctioneer, and user). The state of the user is modeled as a
user-specific click-through rate (CTR) with the CTR changing in the next round according to the
set of ads shown to the user in the current round. We characterize the optimal mechanism for this MDP as a Myerson’s auction with a notion of modified virtual value, which relies on the value distribution of the advertiser, the current user state, and the future impact of showing the ad to the user. Leveraging this characterization, we design a sample-efficient and computationally-efficient algorithm which outputs an approximately optimal policy that requires only sample access to the true MDP and the value distributions of the bidders. Finally, we propose a simple mechanism built upon second price auctions with personalized reserve prices and show it can achieve a constant-factor approximation to the optimal long term discounted revenue.
View details
Preview abstract
Auto-bidding is now widely adopted as an interface between advertisers and internet advertising as it allows advertisers to specify high-level goals, such as maximizing value subject to a value-per-spend constraint. Prior research has mainly focused on auctions that are truthful (such as a second-price auction) because these auctions admit simple (uniform) bidding strategies and are thus simpler to analyze. The main contribution of this paper is to characterize the efficiency across the spectrum of all auctions, including non-truthful auctions for which optimal bidding may be complex.
For deterministic auctions, we show a dominance result: any uniform bidding equilibrium of a second-price auction (SPA) can be mapped to an equilibrium of any other auction – for example, first price auction (FPA) – with identical outcomes. In this sense, SPA with uniform bidding is an instance-wise optimal deterministic auction. Consequently, the price of anarchy (PoA) of any deterministic auction is at least the PoA of SPA with uniform bidding, which is known to be 2. We complement this by showing that the PoA of FPA without uniform bidding is 2.
Next, we show, surprisingly, that truthful pricing is not dominant in the randomized setting. There is a randomized version of FPA that achieves a strictly smaller price of anarchy than its truthful counterpart when there are two bidders per query. Furthermore, this randomized FPA achieves the best-known PoA for two bidders, thus showing the power of non-truthfulness when combined with randomization. Finally, we show that no prior-free auction (even randomized, non-truthful) can improve on a PoA bound of 2 when there are a large number of advertisers per auction. These results should be interpreted qualitatively as follows. When the auction pressure is low, randomization and non-truthfulness is beneficial. On the other hand, if the auction pressure is intense, the benefits diminishes and it is optimal to implement a second-price auction.
View details
Incentive Compatibility in the Auto-bidding World
Yeganeh Alimohammadi
Andres Perlroth
24th ACM Conference on Economics and Computation, EC 2023
Preview abstract
Auto-bidding has recently become a popular feature in ad auctions. This feature enables advertisers to simply provide high-level constraints and goals to an automated agent, which optimizes their auction bids on their behalf. These auto-bidding intermediaries interact in a decentralized manner in the underlying auctions, leading to new interesting practical and theoretical questions on auction design, for example, in understanding the bidding equilibrium properties between auto-bidder intermediaries for different auctions. In this paper, we examine the effect of different auctions on the incentives of advertisers to report their constraints to the auto-bidder intermediaries. More precisely, we study whether canonical auctions such as first price auction (FPA) and second price auction (SPA) are auto-bidding incentive compatible (AIC): whether an advertiser can gain by misreporting their constraints to the autobidder.
View details
Preview abstract
Advertisers in online ad auctions are increasingly using auto-bidding mechanisms to bid into auctions instead of directly bidding their value manually. One of the prominent auto-bidding formats is that of target cost-per-acquisition (tCPA) which maximizes the volume of conversions subject to a return-of-investment constraint. From an auction theoretic perspective however, this trend seems to go against foundational results that postulate that for profit-maximizing (aka quasi-linear) bidders, it is optimal to use a classic bidding system like marginal CPA (mCPA) bidding rather than using strategies like tCPA.
In this paper we rationalize the adoption of such seemingly sub-optimal bidding within the canonical quasi-linear framework. The crux of the argument lies in the notion of commitment. We consider a multi-stage game where first the auctioneer declares the auction rules; then bidders select either the tCPA or mCPA bidding format (and submit bids accordingly); and then, if the auctioneer lacks commitment, it can revisit the rules of the auction (e.g., may readjust reserve prices depending on the bids submitted by the bidders). Our main result is that so long as a bidder believes that the auctioneer lacks commitment to follow the rule of the declared auction then the bidder will make a higher profit by choosing the tCPA format compared to the classic mCPA format.
We then explore the commitment consequences for the auctioneer. In a simplified version of the model where there is only one bidder, we show that the tCPA subgame admits a credible equilibrium while the mCPA format does not. That is, when the bidder chooses the tCPA format the auctioneer can credibly implement the auction rules announced at the beginning of the game. We also show that, under some mild conditions, the auctioneer’s revenue is larger when the bidder uses the tCPA format rather than mCPA. We further quantify the value for the auctioneer to be able to commit to the declared auction rules.
View details
Simple Mechanisms for Welfare Maximization in Rich Advertising Auctions
Divyarthi Mohan
Alex Psomas
Advances in Neural Information Processing Systems 35 (NeurIPS 2022) Main Conference Track
Preview abstract
Internet ad auctions have evolved from a few lines of text to richer informational layouts that include images, sitelinks, videos, etc. Ads in these new formats occupy varying amounts of space, and an advertiser can provide multiple formats, only one of which can be shown.The seller is now faced with a multi-parameter mechanism design problem.Computing an efficient allocation is computationally intractable, and therefore the standard Vickrey-Clarke-Groves (VCG) auction, while truthful and welfare-optimal, is impractical. In this paper, we tackle a fundamental problem in the design of modern ad auctions. We adopt a
Myersonian'' approach and study allocation rules that are monotone both in the bid and set of rich ads. We show that such rules can be paired with a payment function to give a truthful auction. Our main technical challenge is designing a monotone rule that yields a good approximation to the optimal welfare. Monotonicity doesn't hold for standard algorithms, e.g. the incremental bang-per-buck order, that give good approximations to
knapsack-like'' problems such as ours. In fact, we show that no deterministic monotone rule can approximate the optimal welfare within a factor better than 2 (while there is a non-monotone FPTAS). Our main result is a new, simple, greedy and monotone allocation rule that guarantees a 3-approximation. In ad auctions in practice, monotone allocation rules are often paired with the so-called \emph{Generalized Second Price (GSP)} payment rule, which charges the minimum threshold price below which the allocation changes. We prove that, even though our monotone allocation rule paired with GSP is not truthful, its Price of Anarchy (PoA) is bounded. Under standard no-overbidding assumptions, we prove bounds on the a pure and Bayes-Nash PoA. Finally, we experimentally test our algorithms on real-world data.
View details
Auction design in an auto-bidding setting: Randomization improves efficiency beyond VCG
Proceedings of the ACM Web Conference 2022, pp. 173-181
Preview abstract
Auto-bidding is an area of increasing importance in the domain of online advertising. We study the problem of designing auctions in an auto-bidding setting with the goal of maximizing welfare at system equilibrium. Previous results showed that the price of anarchy (PoA) under VCG is 2 and also that this is tight even with two bidders. This raises an interesting question as to whether VCG yields the best efficiency in this setting, or whether the PoA can be improved upon. We present a prior-free randomized auction in which the PoA is approx. 1.896 for the case of two bidders, proving that one can achieve an efficiency strictly better than that under VCG in this setting. We also provide a stark impossibility result for the problem in general as the number of bidders increases -- we show that no (randomized) anonymous truthful auction can have a PoA strictly better than 2 asymptotically as the number of bidders per query increases. While it was shown in previous work that one can improve on the PoA of 2 if the auction is allowed to use the bidder's values for the queries in addition to the bidder's bids, we note that our randomized auction is prior-free and does not use such additional information; our impossibility result also applies to auctions without additional value information.
View details
Convergence Analysis of No-Regret Bidding Algorithms in Repeated Auctions
Zhe Feng
Guru Prashanth Guruganesh
Chris Liaw
Abhishek Sethi
The Thirty-Fifth AAAI Conference on Artificial Intelligence (AAAI-21) (2021)
Preview abstract
The connection between games and no-regret algorithms has been widely studied in the literature. A
fundamental result is that when all players play no-regret strategies, this produces a sequence of actions
whose time-average is a coarse-correlated equilibrium of the game. However, much less is known about
equilibrium selection in the case that multiple equilibria exist.
In this work, we study the convergence of no-regret bidding algorithms in auctions. Besides being of
theoretical interest, bidding dynamics in auctions is an important question from a practical viewpoint as
well. We study repeated game between bidders in which a single item is sold at each time step and the
bidder’s value is drawn from an unknown distribution. We show that if the bidders use any mean-based
learning rule then the bidders converge with high probability to the truthful pure Nash Equilibrium in a
second price auction, in VCG auction in the multi-slot setting and to the Bayesian Nash equilibrium in a
first price auction. We note mean-based algorithms cover a wide variety of known no-regret algorithms
such as Exp3, UCB, -Greedy etc. Also, we analyze the convergence of the individual iterates produced by
such learning algorithms, as opposed to the time-average of the sequence. Our experiments corroborate
our theoretical findings and also find a similar convergence when we use other strategies such as Deep
Q-Learning.
View details
Hitting the High Notes: Subset Selection for Maximizing Expected Order Statistics
Alex Psomas
Aviad Rubinstein
Advances in Neural Information Processing Systems (2020)
Preview abstract
We consider the fundamental problem of selecting k out of n random variables
in a way that the expected highest or second-highest value is maximized. This
question captures several applications where we have uncertainty about the quality
of candidates (e.g. auction bids, search results) and have the capacity to explore
only a small subset due to an exogenous constraint. For example, consider a second
price auction where system constraints (e.g., costly retrieval or model computation)
allow the participation of only k out of n bidders, and the goal is to optimize the
expected efficiency (highest bid) or expected revenue (second highest bid).
We study the case where we are given an explicit description of each random
variable. We give a PTAS for the problem of maximizing the expected highest value.
For the second-highest value, we prove a hardness result: assuming the Planted
Clique Hypothesis, there is no constant factor approximation algorithm that runs
in polynomial time. Surprisingly, under the assumption that each random variable
has monotone hazard rate (MHR), a simple score-based algorithm, namely picking
the k random variables with the largest 1/\sqrt{k} top quantile value, is a constant
approximation to the expected highest and second highest value, simultaneously.
View details
Preview abstract
Autobidding is becoming increasingly important in the domain of online advertising, and has become a critical tool used by many advertisers for optimizing their ad campaigns. We formulate fundamental questions around the problem of bidding for performance under very general affine cost constraints. We design optimal single-agent bidding strategies for the general bidding problem, in multi-slot truthful auctions. We show that there is an intimate connection between bidding and auction design, in that the bidding formula is optimal if and only if the underlying auction is truthful. We show how a MWU algorithm can be used to learn this optimal bidding formula.
Next, we move from the single-agent view to taking a full-system view: What happens when all advertisers adopt optimal autobidding? We prove that in general settings, there exists an equilibrium between the bidding agents for all the advertisers. Further, we prove a Price of Anarchy result: For the general affine constraints, the total value (conversions) obtained by the advertisers in the bidding agent equilibrium is no less than 1/2 of what we could generate via a centralized ad allocation scheme, one which does not consider any auction incentives or provide any per-advertiser guarantee.
View details