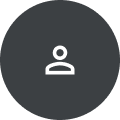
Fernando Diaz
Fernando Diaz is a research scientist at Google. Fernando’s research focuses on the design of information access systems, including search engines, music recommendation services and crisis response platforms. He is particularly interested in understanding and addressing the societal implications of artificial intelligence more generally. Previously, Fernando was the assistant managing director of Microsoft Research Montréal, where he also led FATE Montréal, and a director of research at Spotify, where he helped establish its research organization on recommendation, search, and personalization. Fernando’s work has received special recognition and awards at SIGIR, CIKM, CSCW, WSDM, ISCRAM, and ECIR. He is the recipient of the 2017 British Computer Society Karen Spärck Jones Award and holds a CIFAR AI Chair. Fernando has co-organized several NIST TREC tracks, WSDM (2013), Strategic Workshop on Information Retrieval (2018), FAT* (2019), SIGIR (2021), and the CIFAR Workshop on Artificial Intelligence and the Curation of Culture (2019). He received his BS in Computer Science from the University of Michigan Ann Arbor and his MS and PhD from the University of Massachusetts Amherst.
Authored Publications
Sort By
Google
Density-based User Representation through Gaussian Process Regression for Multi-interest Personalized Retrieval
Haolun Wu
Xue Liu
Thirty-Eighth Annual Conference on Neural Information Processing Systems (NeurIPS-24), Vancouver (2024)
On Natural Language User Profiles for Transparent and Scrutable Recommendation
Proceedings of the 45th International ACM SIGIR Conference on Research and Development in Information Retrieval (SIGIR '22) (2022)
Joint Multisided Exposure Fairness for Recommendation
Bhaskar Mitra
Chen Ma
Haolun Wu
Xue Liu
Proceedings of the 45th International ACM SIGIR Conference on Research and Development in Information Retrieval (2022)
Measuring Commonality in Recommendation of Cultural Content: Recommender Systems to Enhance Cultural Citizenship
Andres Ferraro
Georgina Born
Gustavo Ferreira
Proceedings of the 16th ACM Conference on Recommender Systems (2022)
Retrieval Enhanced Machine Learning
Hamed Zamani
Mike Bendersky
SIGIR 2022: Proceedings of the 45th International ACM SIGIR Conference on Research and Development in Information Retrieval (Perspectives Track)
Offline Retrieval Evaluation Without Evaluation Metrics
Andres Ferraro
Proceedings of the 45th International ACM SIGIR Conference on Research and Development in Information Retrieval (2022)
On Evaluating Session-Based Recommendation with Implicit Feedback
Perspectives on the Evaluation of Recommender Systems Workshop (PERSPECTIVES 2021)
Art Sheets for Art Datasets
Ramya Malur Srinivasan
Jordan Jennifer Famularo
Beth Coleman
NeurIPS Dataset & Benchmark track (2021)