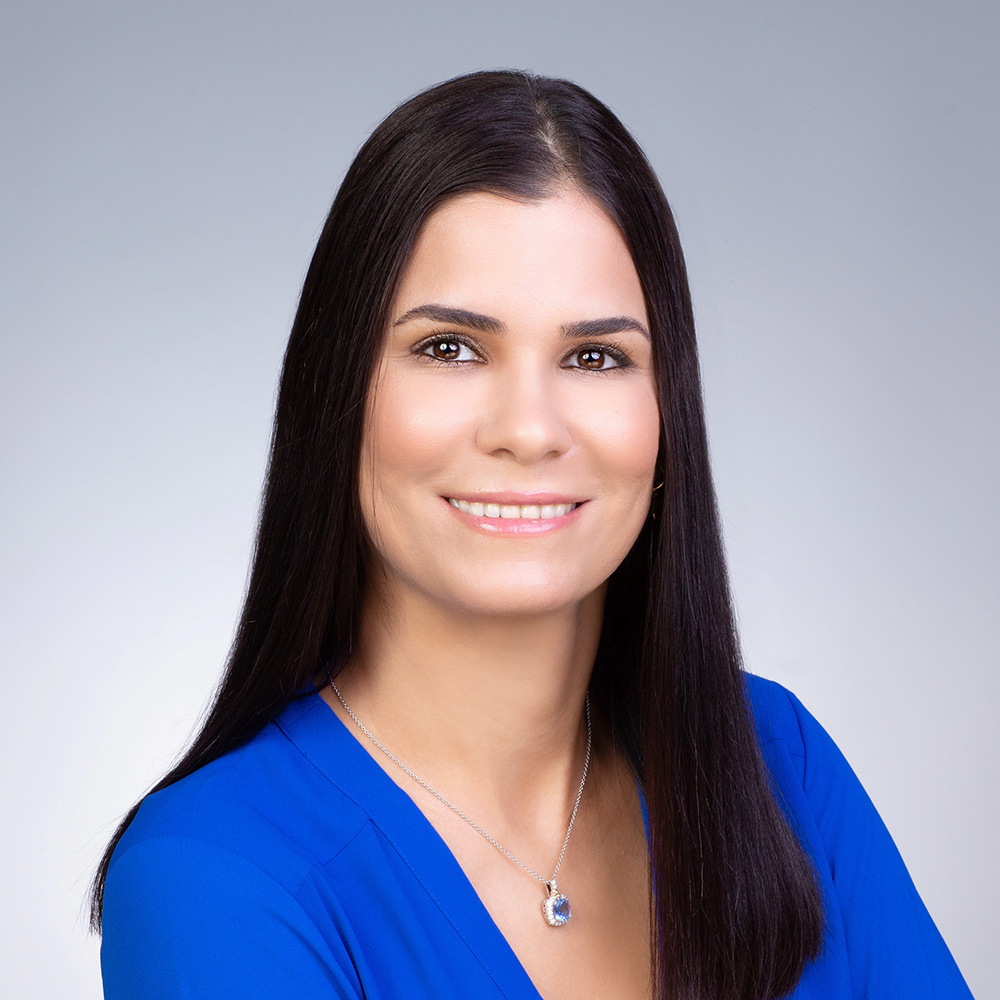
Maryam Karimzadehgan
As the Engineering Lead for Android Intelligence, Dr. Maryam Karimzadehgan is at the forefront of developing cutting-edge Generative AI models. Her impressive record includes over 35 research papers, 10 patents, and prestigious awards like the Google PhD Fellowship and the Yahoo! Key Scientific Challenges Award. She earned her PhD from the University of Illinois at Urbana-Champaign.
Research Areas
Authored Publications
Sort By
Google
Density-based User Representation through Gaussian Process Regression for Multi-interest Personalized Retrieval
Haolun Wu
Xue Liu
Thirty-Eighth Annual Conference on Neural Information Processing Systems (NeurIPS-24), Vancouver (2024)
Overcoming Prior Misspecification in Online Learning to Rank
Mohammadjavad Azizi
International Conference on Artificial Intelligence and Statistics, PMLR (2023), pp. 594-614
Ever Evolving Evaluator (EV3): Towards Flexible and Reliable Meta-Optimization for Knowledge Distillation
Li Ding
NeurIPS 2023 Workshop on Adaptive Experimental Design and Active Learning in the Real World (2023)
IMO^3: Interactive Multi-Objective Off-Policy Optimization
Nan Wang
Hongning Wang
Branislav Kveton
Proceedings of the 31st International Joint Conference on Artificial Intelligence (IJCAI-22), Vienna (2022), pp. 3523-3529
Separate And Attend in Personal Email Search
Yu Meng
Proceedings of the 13th ACM International Conference on Web Search and Data Mining (WSDM) (2020)
Domain Adaptation for Enterprise Email Search
Brandon Tran
Mike Bendersky
Proceedings of the 42nd International ACM SIGIR Conference on Research and Development in Information Retrieval (SIGIR) (2019)
Multi-Task Learning for Personal Search Ranking with Query Clustering
Jiaming Shen
Michael Bendersky
Proceedings of ACM Conference on Information and Knowledge Management (CIKM) (2018)