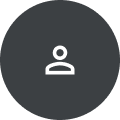
Krista Reymann
Program Manager, Robotics at Google
Authored Publications
Sort By
Robotic Table Tennis: A Case Study into a High Speed Learning System
Jon Abelian
Saminda Abeyruwan
Michael Ahn
Justin Boyd
Erwin Johan Coumans
Omar Escareno
Wenbo Gao
Navdeep Jaitly
Juhana Kangaspunta
Satoshi Kataoka
Gus Kouretas
Yuheng Kuang
Corey Lynch
Thinh Nguyen
Ken Oslund
Barney J. Reed
Anish Shankar
Avi Singh
Grace Vesom
Peng Xu
Robotics: Science and Systems (2023)
Preview abstract
We present a deep-dive into a learning robotic system that, in previous work, was shown to be capable of hundreds of table tennis rallies with a human and has the ability to precisely return the ball to desired targets. This system puts together a highly optimized and novel perception subsystem, a high-speed low-latency robot controller, a simulation paradigm that can prevent damage in the real world and also train policies for zero-shot transfer, and automated real world environment resets that enable autonomous training and evaluation on physical robots. We complement a complete system description including numerous design decisions that are typically not widely disseminated, with a collection of ablation studies that clarify the importance of mitigating various sources of latency, accounting for training and deployment distribution shifts, robustness of the perception system, and sensitivity to policy hyper-parameters and choice of action space. A video demonstrating the components of our system and details of experimental results is included in the supplementary material.
View details
Google Scanned Objects: A High-Quality Dataset of 3D Scanned Household Items
Anthony G. Francis
Brandon Kinman
Laura Downs
Nathan Koenig
Ryan M. Hickman
Thomas B. McHugh
(2022)
Preview abstract
Interactive 3D simulations have enabled breakthroughs in robotics and computer vision, but simulating the broad diversity of environments needed for deep learning requires large corpora of photo-realistic 3D object models. To address this need, we present Google Scanned Objects, an open-source collection of over one thousand 3D-scanned household items; these models are preprocessed for use in Ignition Gazebo and the Bullet simulation platforms, but are easily adaptable to other simulators.
We describe our object scanning and curation pipeline, then provide statistics about the contents of the dataset and its usage. We hope that the diversity, quality, and flexibility that Google Scanned Objects provides will lead to further advances in interactive simulation, synthetic perception, and robotic learning.
View details