Impossible? Let’s see.
Whether we're shaping the future of sustainability, or optimizing algorithms, or even exploring epidemiological studies, Google Research strives to continuously progress science, advance society, and improve the lives of billions of people.

Impossible? Let’s see.

Advancing the state of the art
Our teams advance the state of the art through research, systems engineering, and collaboration across Google. We publish hundreds of research papers each year across a wide range of domains, sharing our latest developments in order to collaboratively progress computing and science.
Learn more about our philosophy.
Our research drives real-world change
Med-PaLM 2
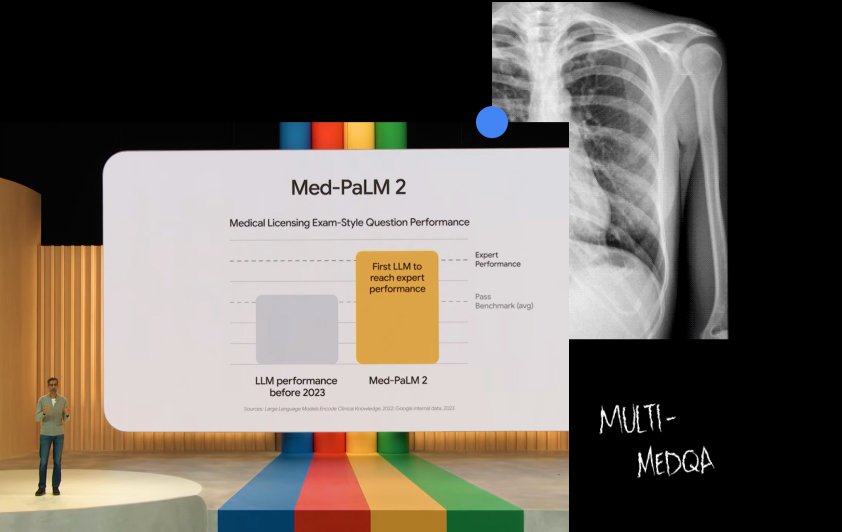
Improving our LLM designed for the medical domain
See the project Explore the research behind the projectProject Contrails
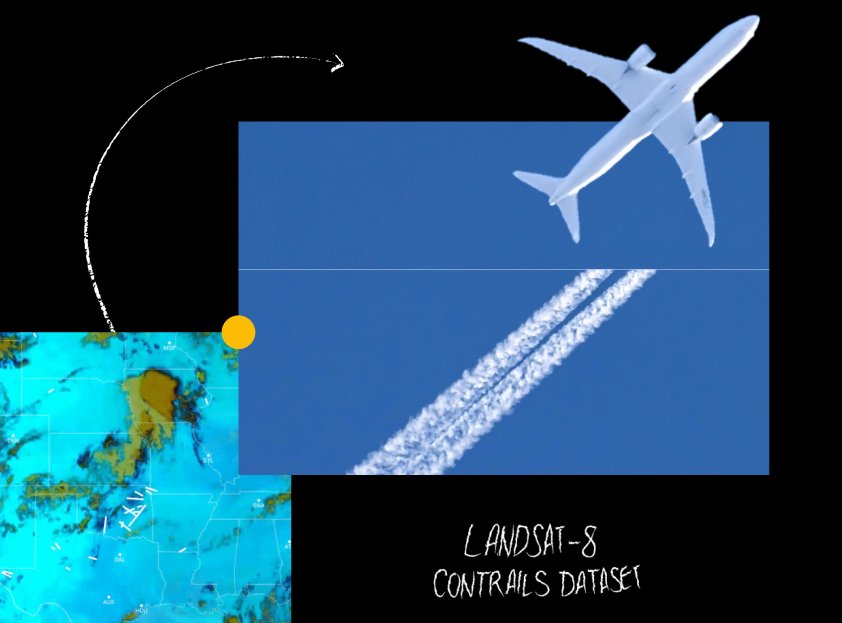
A cost-effective and scalable way AI is helping to mitigate aviation’s climate impact
See the project Explore the research behind the projectSee our impact across other projects
One research paper started it all
The research we do today becomes the Google of the future. Google itself began with a research paper, published in 1998, and was the foundation of Google Search. Our ongoing research over the past 25 years has transformed not only the company, but how people are able to interact with the world and its information.
See the original publication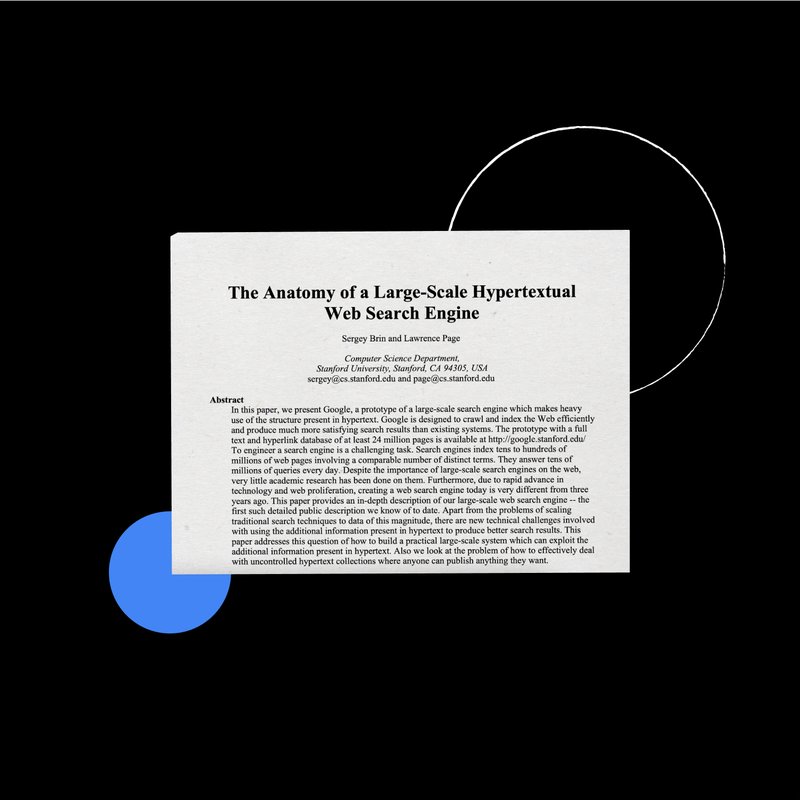
Responsible research is at the heart of what we do
The impact we create from our research has the potential to reach billions of people. That's why everything we do is guided by methodology that is grounded in responsible practices and thorough consideration.
See how we're advancing AI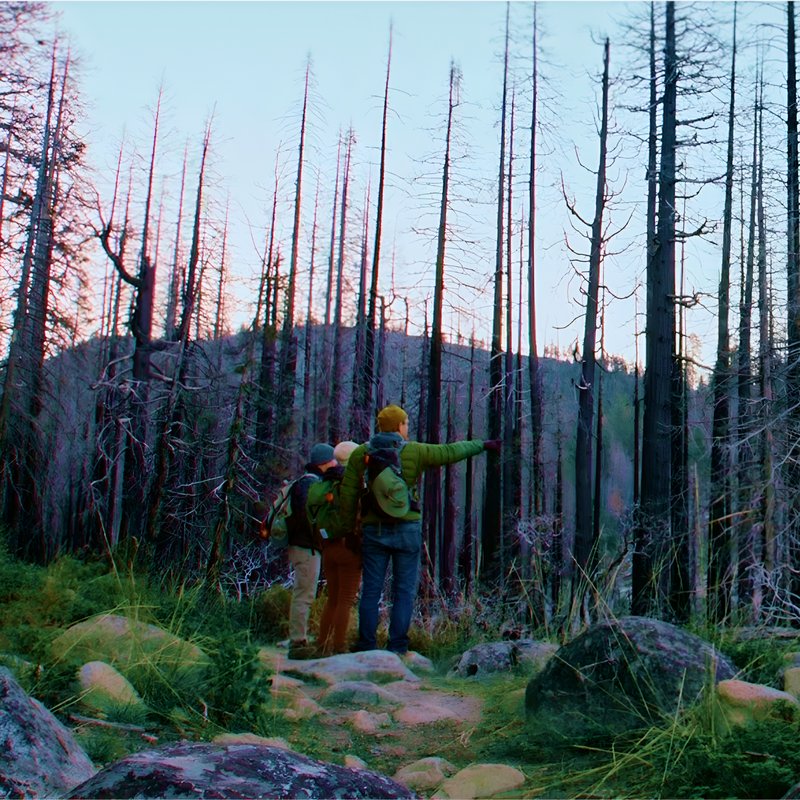